Multi-sensor integrated mapping of global XCO2 from 2015 to 2021 with a local random forest model
ISPRS JOURNAL OF PHOTOGRAMMETRY AND REMOTE SENSING(2024)
摘要
Carbon dioxide (CO2) is one of the most important greenhouse gases in the atmosphere, and carbon satellites play a vital role in monitoring its concentration. However, a single carbon satellite often has inadequate spatial coverage, resulting in numerous gaps. Utilizing the complementary advantages of multiple satellites in spatial coverage for high-coverage mapping of CO2 may be an effective means. To this end, this study proposes a local random forest (LRF) model to generate a global high-coverage and high-precision column-averaged dry-air mole fraction of CO2 (XCO2) product from 2015 to 2021, which integrates multi-source data from OCO-2, OCO-3, GOSAT, and GOSAT-2. The results indicate that the LRF reconstructions agree well with the ground-based site observations, with RMSE, MAE, and R2 of 1.08 ppm, 0.82 ppm, and 0.96, respectively, outperforming the global random forest and artificial neural network models. Meanwhile, multi-source satellite fusion effectively improves the global XCO2 coverage, with a maximum improvement rate of 122.2% compared to the single satellite of OCO2. Based on the reconstructed dataset, regional and seasonal differences in the global XCO2 distribution are observed, and an average growth rate of 2.18 ppm/year of global XCO2 is revealed during 2015-2021. This study combines data from multi-source satellites to generate high-coverage global XCO2 products from 2015 to 2021, which can be freely accessed from https://github.com/ch00en/Multi-source-satellite-XCO2-fusion-products.git and would hold great potential for carbon cycle research.
更多查看译文
关键词
Local random forest,Global distribution,Spatial and temporal variation
AI 理解论文
溯源树
样例
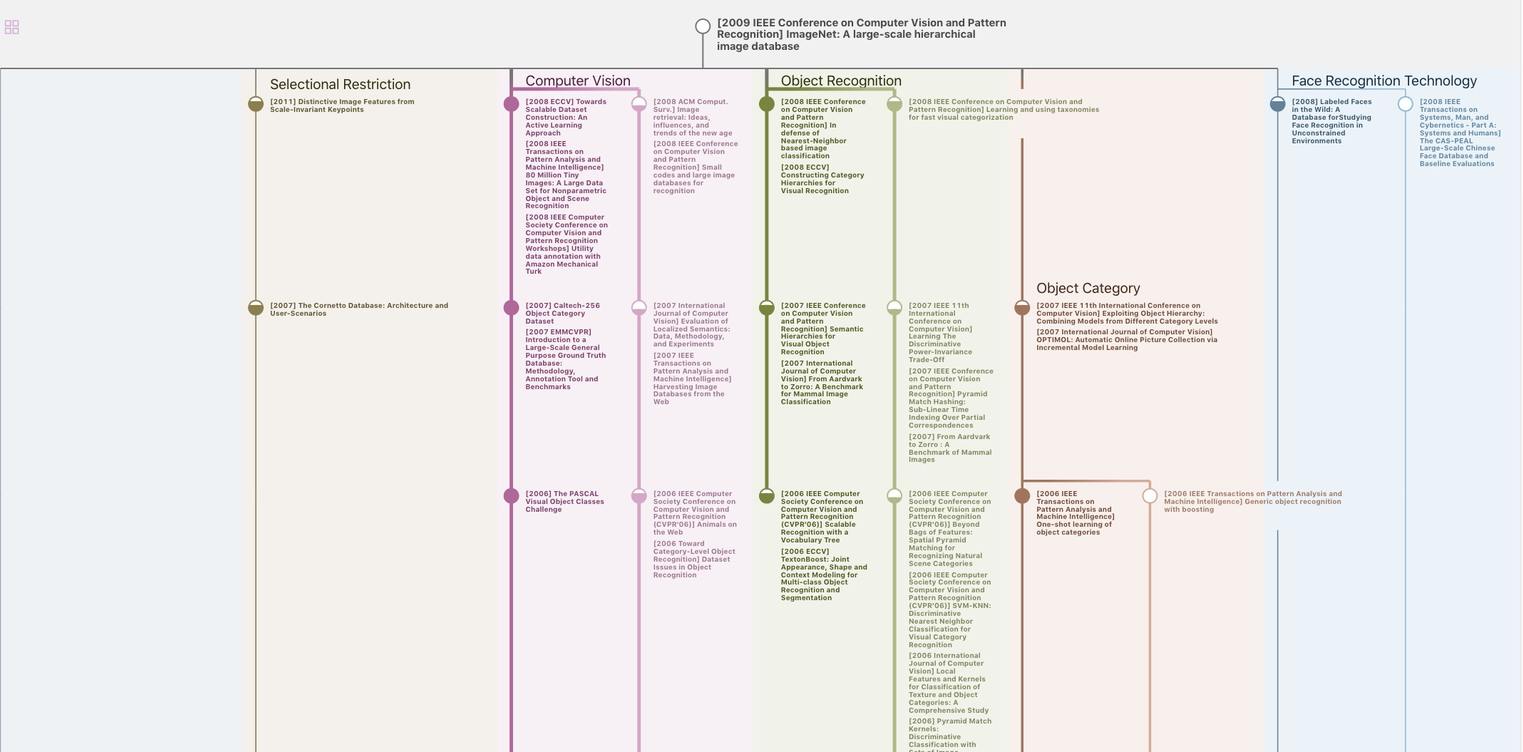
生成溯源树,研究论文发展脉络
Chat Paper
正在生成论文摘要