Enhancing Soil Texture Classification with Multivariate Scattering Correction and Residual Neural Networks Using Visible Near-Infrared Spectra
Journal of Environmental Management(2024)
Key words
Soil texture,Visible near-infrared spectra,Multiplicative scatter correction,Residual neural network,Deep learning,Land cover
AI Read Science
Must-Reading Tree
Example
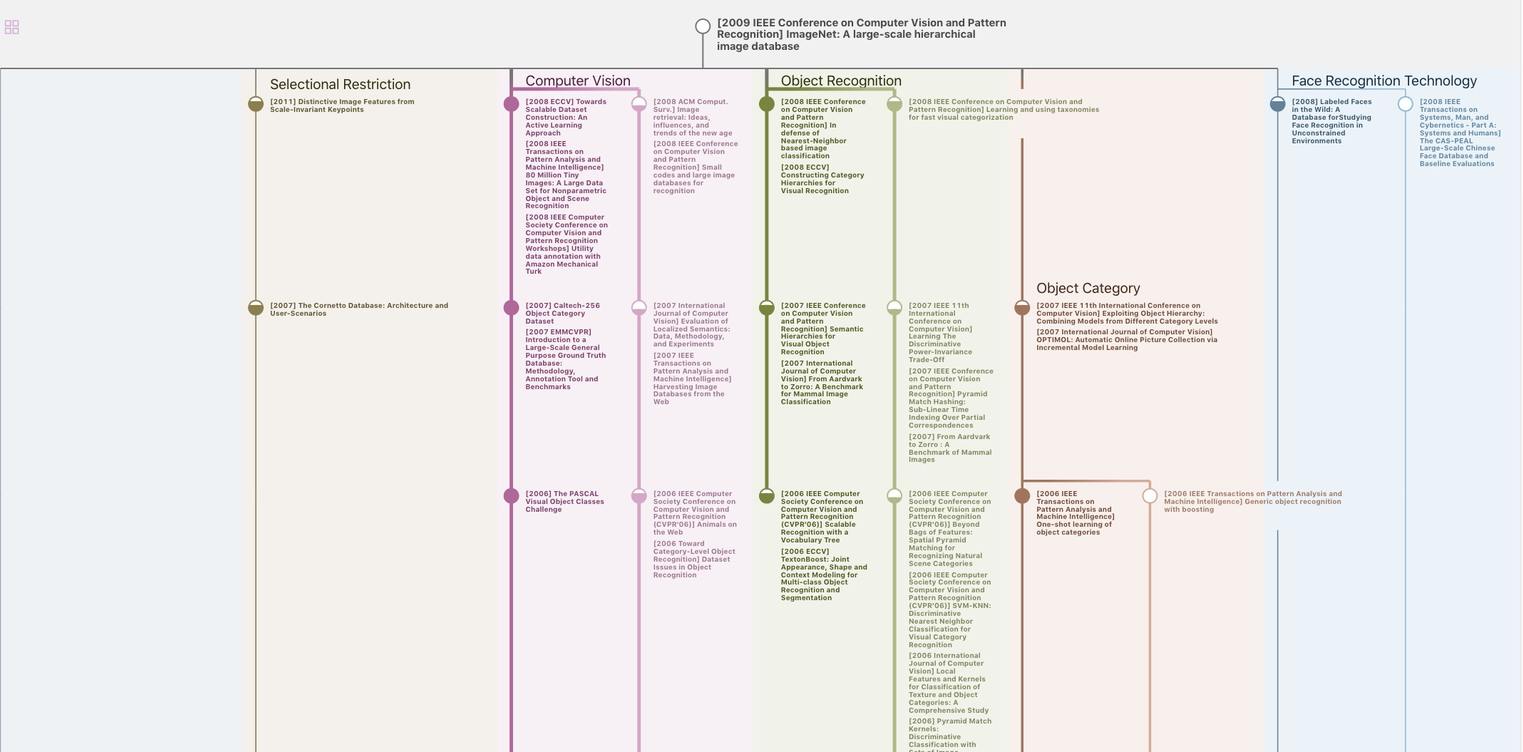
Generate MRT to find the research sequence of this paper
Chat Paper
Summary is being generated by the instructions you defined