Merging uncertainty sets via majority vote
arxiv(2024)
摘要
Given K uncertainty sets that are arbitrarily dependent – for example,
confidence intervals for an unknown parameter obtained with K different
estimators, or prediction sets obtained via conformal prediction based on K
different algorithms on shared data – we address the question of how to
efficiently combine them in a black-box manner to produce a single uncertainty
set. We present a simple and broadly applicable majority vote procedure that
produces a merged set with nearly the same error guarantee as the input sets.
We then extend this core idea in a few ways: we show that weighted averaging
can be a powerful way to incorporate prior information, and a simple
randomization trick produces strictly smaller merged sets without altering the
coverage guarantee. Along the way, we prove an intriguing result that Rüger's
combination rules (eg: twice the median of dependent p-values is a p-value) can
be strictly improved with randomization. When deployed in online settings, we
show how the exponential weighted majority algorithm can be employed in order
to learn a good weighting over time. We then combine this method with adaptive
conformal inference to deliver a simple conformal online model aggregation
(COMA) method for nonexchangeable data.
更多查看译文
AI 理解论文
溯源树
样例
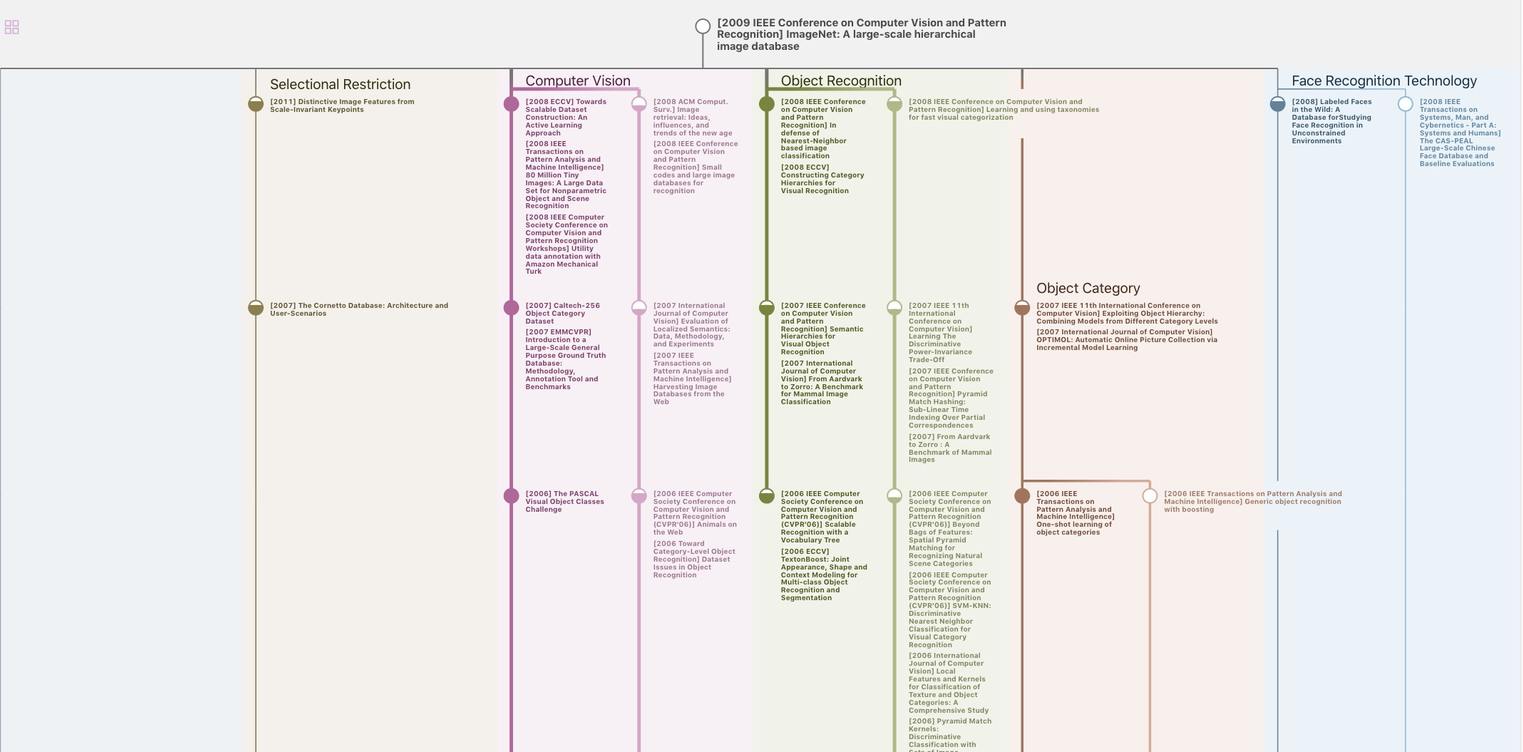
生成溯源树,研究论文发展脉络
Chat Paper
正在生成论文摘要