PixelDINO: Semi-Supervised Semantic Segmentation for Detecting Permafrost Disturbances
CoRR(2024)
摘要
Arctic Permafrost is facing significant changes due to global climate change.
As these regions are largely inaccessible, remote sensing plays a crucial rule
in better understanding the underlying processes not just on a local scale, but
across the Arctic. In this study, we focus on the remote detection of
retrogressive thaw slumps (RTS), a permafrost disturbance comparable to
landslides induced by thawing. For such analyses from space, deep learning has
become an indispensable tool, but limited labelled training data remains a
challenge for training accurate models. To improve model generalization across
the Arctic without the need for additional labelled data, we present a
semi-supervised learning approach to train semantic segmentation models to
detect RTS. Our framework called PixelDINO is trained in parallel on labelled
data as well as unlabelled data. For the unlabelled data, the model segments
the imagery into self-taught pseudo-classes and the training procedure ensures
consistency of these pseudo-classes across strong augmentations of the input
data. Our experimental results demonstrate that PixelDINO can improve model
performance both over supervised baseline methods as well as existing
semi-supervised semantic segmentation approaches, highlighting its potential
for training robust models that generalize well to regions that were not
included in the training data. The project page containing code and other
materials for this study can be found at
.
更多查看译文
AI 理解论文
溯源树
样例
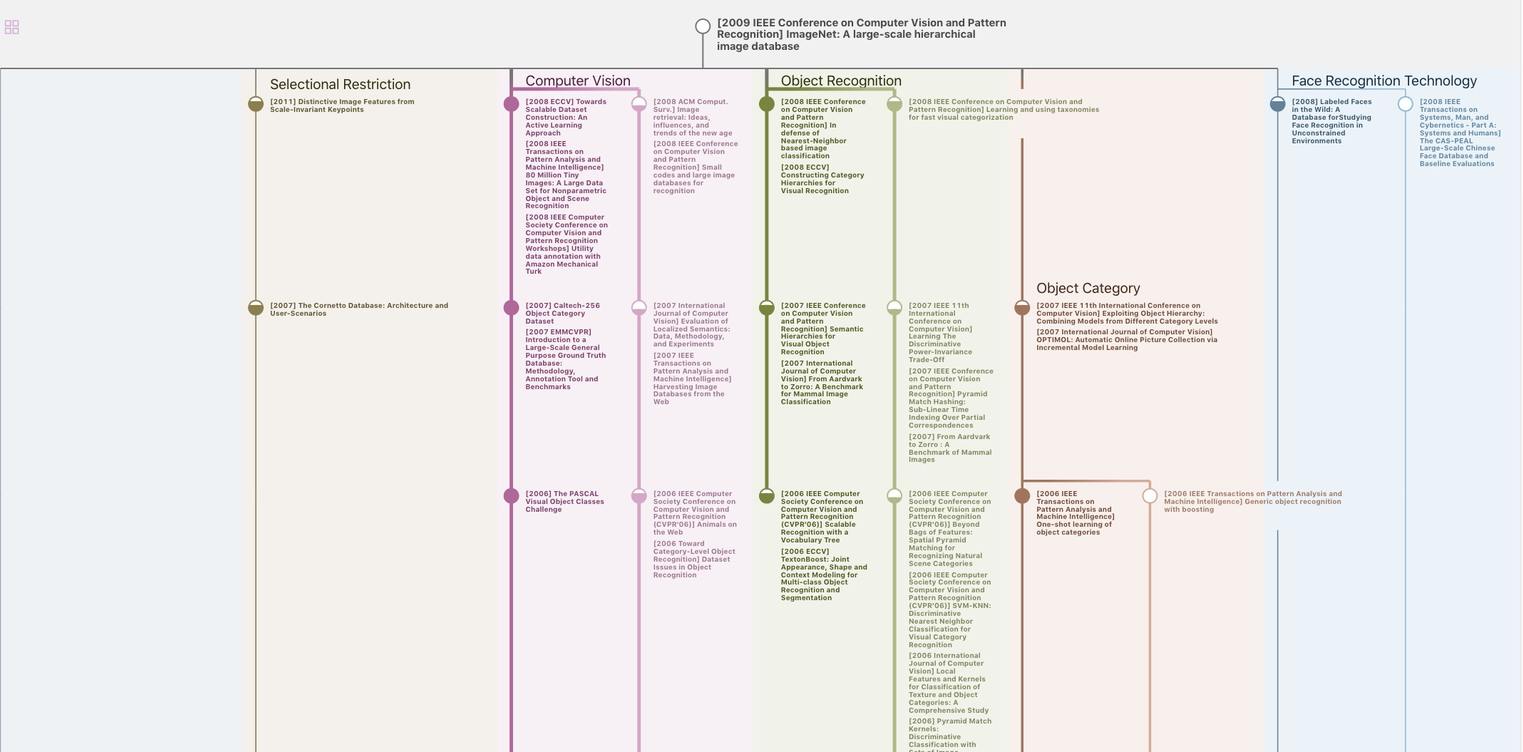
生成溯源树,研究论文发展脉络
Chat Paper
正在生成论文摘要