Rigid Protein-Protein Docking via Equivariant Elliptic-Paraboloid Interface Prediction
CoRR(2024)
摘要
The study of rigid protein-protein docking plays an essential role in a
variety of tasks such as drug design and protein engineering. Recently, several
learning-based methods have been proposed for the task, exhibiting much faster
docking speed than those computational methods. In this paper, we propose a
novel learning-based method called ElliDock, which predicts an elliptic
paraboloid to represent the protein-protein docking interface. To be specific,
our model estimates elliptic paraboloid interfaces for the two input proteins
respectively, and obtains the roto-translation transformation for docking by
making two interfaces coincide. By its design, ElliDock is independently
equivariant with respect to arbitrary rotations/translations of the proteins,
which is an indispensable property to ensure the generalization of the docking
process. Experimental evaluations show that ElliDock achieves the fastest
inference time among all compared methods and is strongly competitive with
current state-of-the-art learning-based models such as DiffDock-PP and Multimer
particularly for antibody-antigen docking.
更多查看译文
关键词
Equivariant Graph Neural Network,rigid body protein-protein docking,interface fitting
AI 理解论文
溯源树
样例
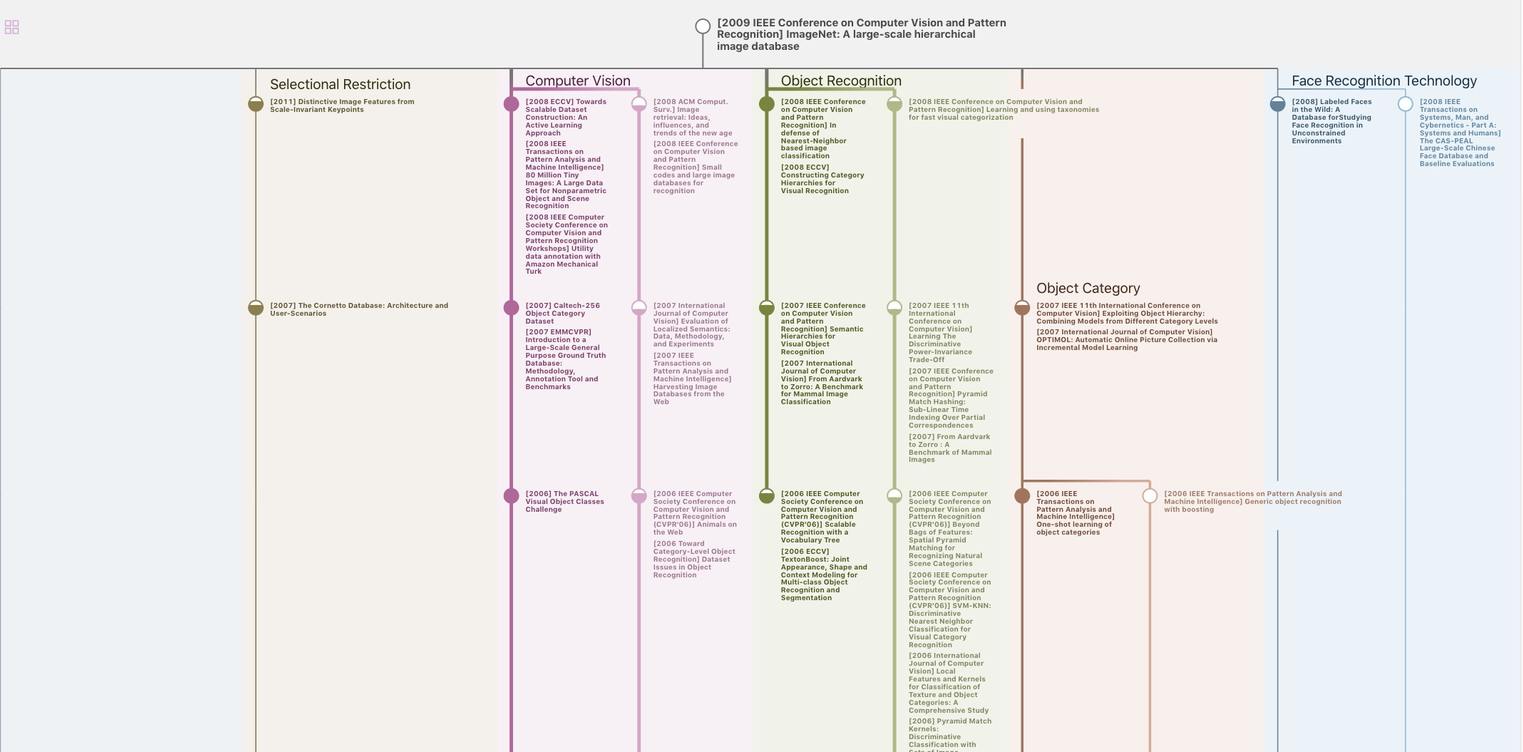
生成溯源树,研究论文发展脉络
Chat Paper
正在生成论文摘要