Fluid Dynamic DNNs for Reliable and Adaptive Distributed Inference on Edge Devices
CoRR(2024)
摘要
Distributed inference is a popular approach for efficient DNN inference at
the edge. However, traditional Static and Dynamic DNNs are not
distribution-friendly, causing system reliability and adaptability issues. In
this paper, we introduce Fluid Dynamic DNNs (Fluid DyDNNs), tailored for
distributed inference. Distinct from Static and Dynamic DNNs, Fluid DyDNNs
utilize a novel nested incremental training algorithm to enable independent and
combined operation of its sub-networks, enhancing system reliability and
adaptability. Evaluation on embedded Arm CPUs with a DNN model and the MNIST
dataset, shows that in scenarios of single device failure, Fluid DyDNNs ensure
continued inference, whereas Static and Dynamic DNNs fail. When devices are
fully operational, Fluid DyDNNs can operate in either a High-Accuracy mode and
achieve comparable accuracy with Static DNNs, or in a High-Throughput mode and
achieve 2.5x and 2x throughput compared with Static and Dynamic DNNs,
respectively.
更多查看译文
关键词
Fluid Dynamic DNNs,Distributed Inference
AI 理解论文
溯源树
样例
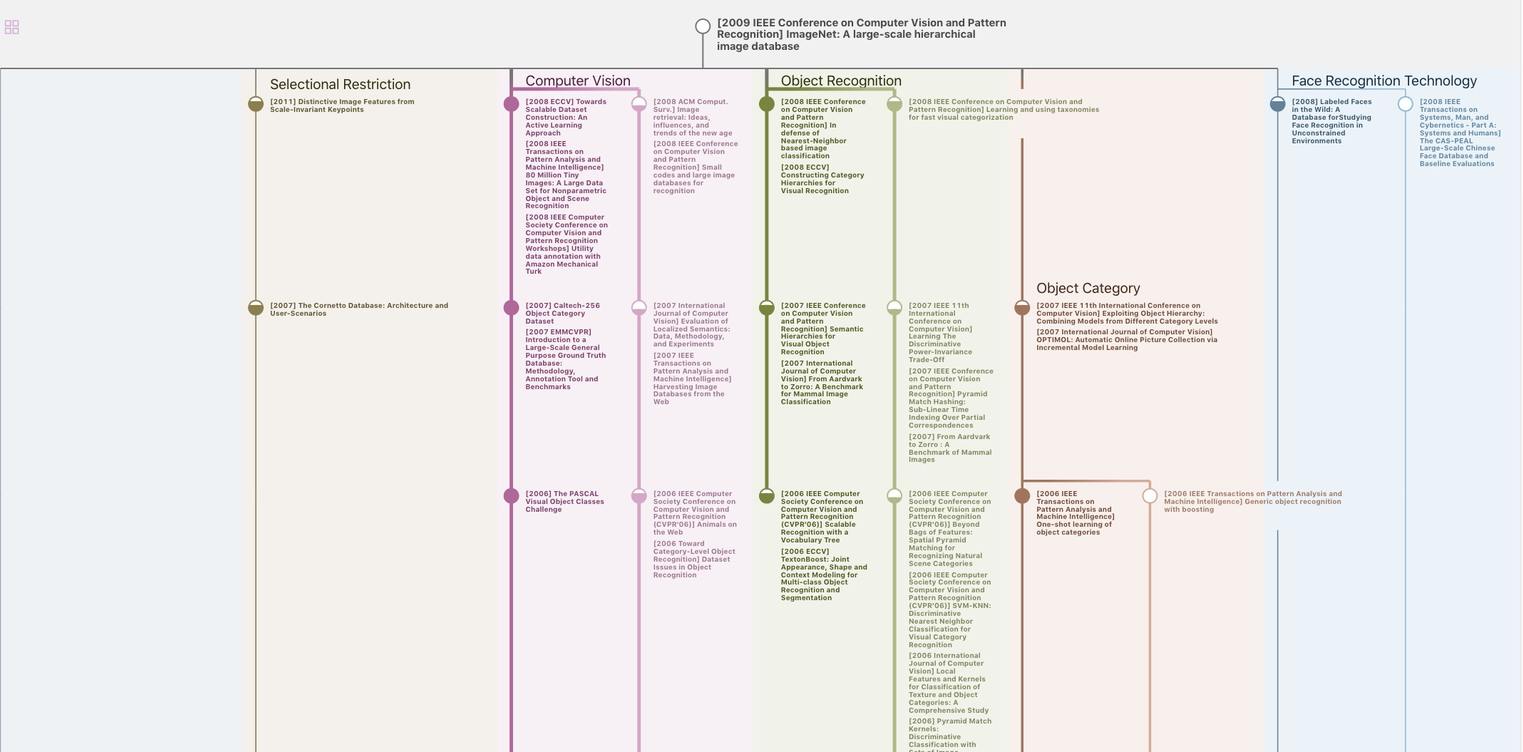
生成溯源树,研究论文发展脉络
Chat Paper
正在生成论文摘要