Evaluating the Utility of Conformal Prediction Sets for AI-Advised Image Labeling
CoRR(2024)
摘要
As deep neural networks are more commonly deployed in high-stakes domains,
their lack of interpretability makes uncertainty quantification challenging. We
investigate the effects of presenting conformal prediction
setsx2013a method for generating valid confidence sets in
distribution-free uncertainty quantificationx2013to express
uncertainty in AI-advised decision-making. Through a large pre-registered
experiment, we compare the utility of conformal prediction sets to displays of
Top-1 and Top-k predictions for AI-advised image labeling. We find that the
utility of prediction sets for accuracy varies with the difficulty of the task:
while they result in accuracy on par with or less than Top-1 and Top-k displays
for easy images, prediction sets excel at assisting humans in labeling
out-of-distribution (OOD) images especially when the set size is small. Our
results empirically pinpoint the practical challenges of conformal prediction
sets and provide implications on how to incorporate them for real-world
decision-making.
更多查看译文
AI 理解论文
溯源树
样例
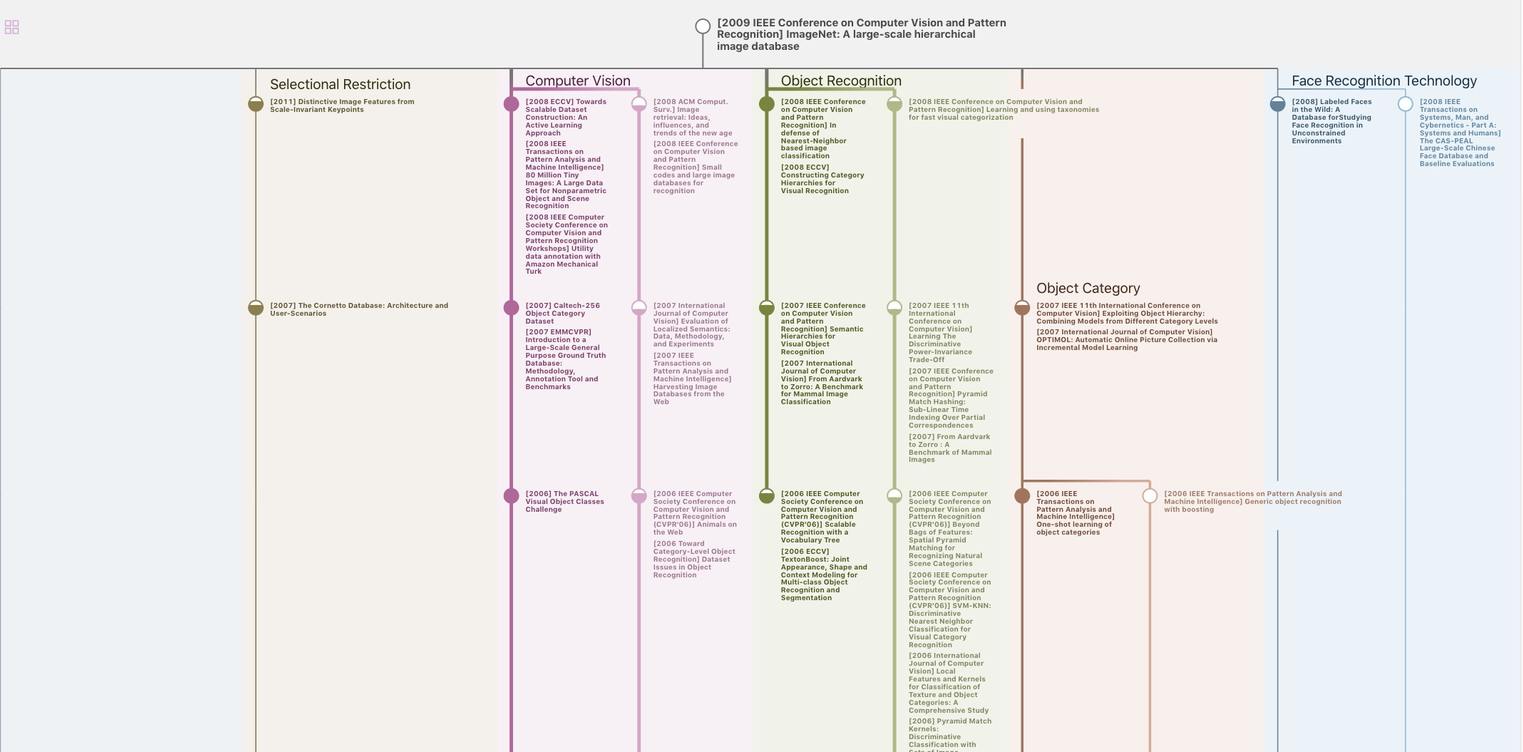
生成溯源树,研究论文发展脉络
Chat Paper
正在生成论文摘要