Implementing Quantization to Indonesian BERT Language Model
2023 10th International Conference on Advanced Informatics: Concept, Theory and Application (ICAICTA)(2023)
摘要
In last several years, BERT has gain its own popularity and become widely adopted for various task and language, including Indonesian. Despite its effectiveness, the humongous size and the costly attention mechanism of BERT yield various efficiency challenges related to latency and model size. Many efforts have been introduce to improve the efficiency of BERT models, but unfortunately, there are very limited efforts to evaluate the model's performance in Indonesian. To address this issue, this paper evaluates compression techniques through quantization for an Indonesian version of the BERT model, namely IndoBERT. Experiments on 7 downstream tasks show a similar trend reported in the previous work where the quantized model achieves slightly degraded performance compared to the full precision model. However, we observe a huge degradation in extreme cases such as 4-bit quantization and sequence labeling tasks when using post-training quantization. While quantization aware training is found to be effective and efficient regardless of the bit depth. Furthermore, our experimental results show that the quantized model can achieve in terms of size and inference speed compared to the full precision model. Furthermore, our experimental results show that the quantized model can achieve in terms of size and inference speed compared to the full precision model. The analysis presented in this work provides that quantization can perform well in Indonesian model, which is an important avenue for bringing better efficiency to various Indonesian language tasks.
更多查看译文
关键词
BERT,transformer-based models,quantization,natural language processing
AI 理解论文
溯源树
样例
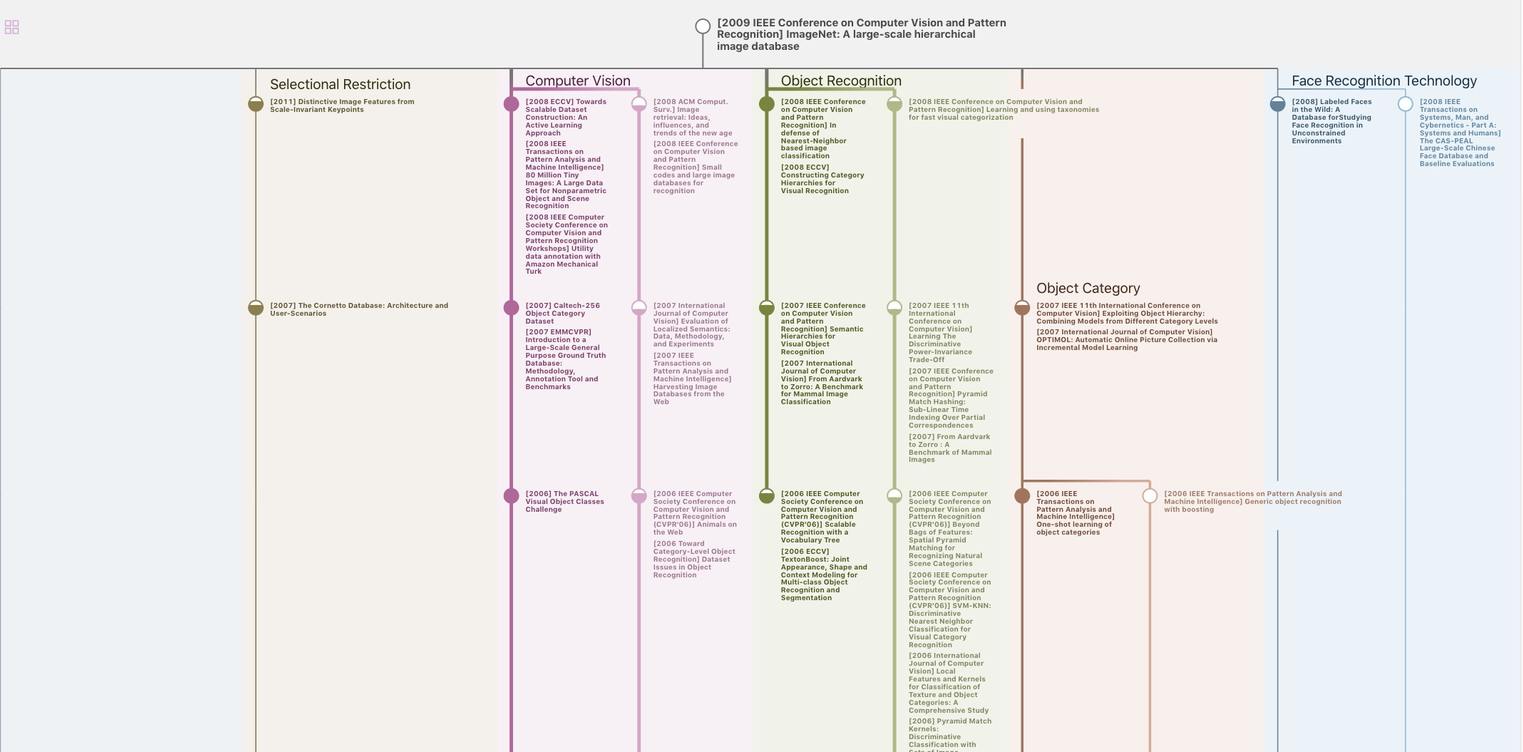
生成溯源树,研究论文发展脉络
Chat Paper
正在生成论文摘要