Sexism Detection and Classification Using RoBERTa and Data Augmentation
2023 10th International Conference on Advanced Informatics: Concept, Theory and Application (ICAICTA)(2023)
摘要
Sexism has been growing in social media due to the lack of consequences one would get for doing it. Explainable Detection of Online Sexism is a dataset provided by Rewire Online to develop models that can detect sexism and explain it by further classifying sexist texts into categories. Using provided dataset, we build models to detect and classify sexism into categories and vectors (sub-categories) using transformer-based model, RoBERTa. As the dataset provided contained a significant imbalance between the classes, the impact of different data augmentation method is also experimented to find out which method will give the best result. The experiment was done by using three different methods: random oversampling, easy data augmentation, and backtranslations. As a result, EDA gave the best performance for sexism detection, back-translations gave the best performance for category classification, and random oversampling gave the best performance for vector classification.
更多查看译文
关键词
sexism,text classification,RoBERTa,data augmentation
AI 理解论文
溯源树
样例
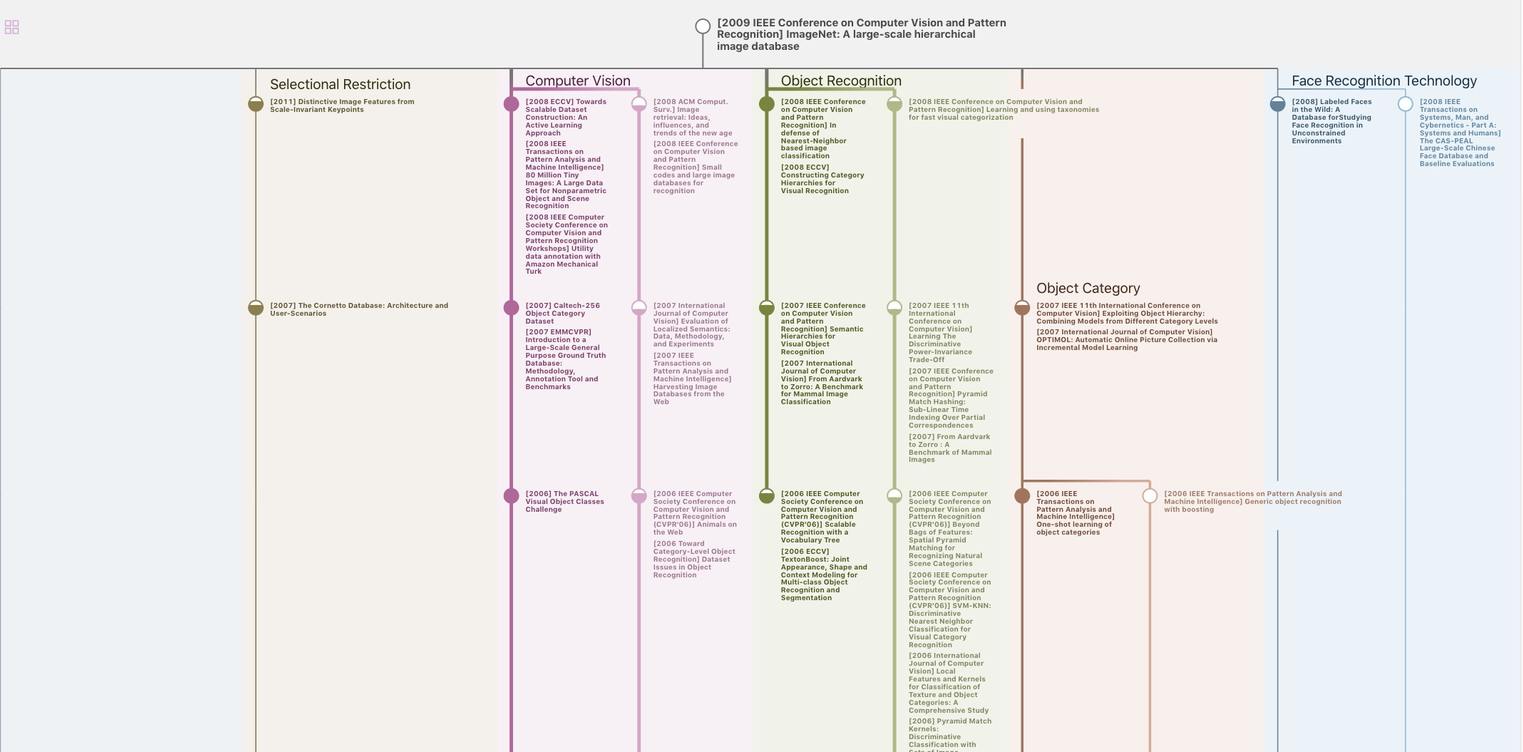
生成溯源树,研究论文发展脉络
Chat Paper
正在生成论文摘要