Investigating Self-supervised Learning for Predicting Stress and Stressors from Passive Sensing
2023 11TH INTERNATIONAL CONFERENCE ON AFFECTIVE COMPUTING AND INTELLIGENT INTERACTION WORKSHOPS AND DEMOS, ACIIW(2023)
摘要
The application of machine learning (ML) techniques for well-being tasks has grown in popularity due to the abundance of passively-sensed data generated by devices. However, the performance of ML models are often limited by the cost associated with obtaining ground truth labels and the variability of well-being annotations. Self-supervised representations learned from large-scale unlabeled datasets have been shown to accelerate the training process, with subsequent fine-tuning to the specific downstream tasks with a relatively small set of annotations. In this paper, we investigate the potential and effectiveness of self-supervised pre-training for well-being tasks, specifically predicting both workplace daily stress as well as the most impactful stressors. Through a series of experiments, we find that self-supervised methods are effective when predicting on unseen users, relative to supervised baselines. Scaling both data size and encoder depth, we observe the superior performance obtained by self-supervised methods, further showcasing their utility for well-being applications. In addition, we present future research directions and insights for applying self-supervised representation learning on well-being tasks.
更多查看译文
关键词
self-supervised learning,workplace stress prediction,well-being
AI 理解论文
溯源树
样例
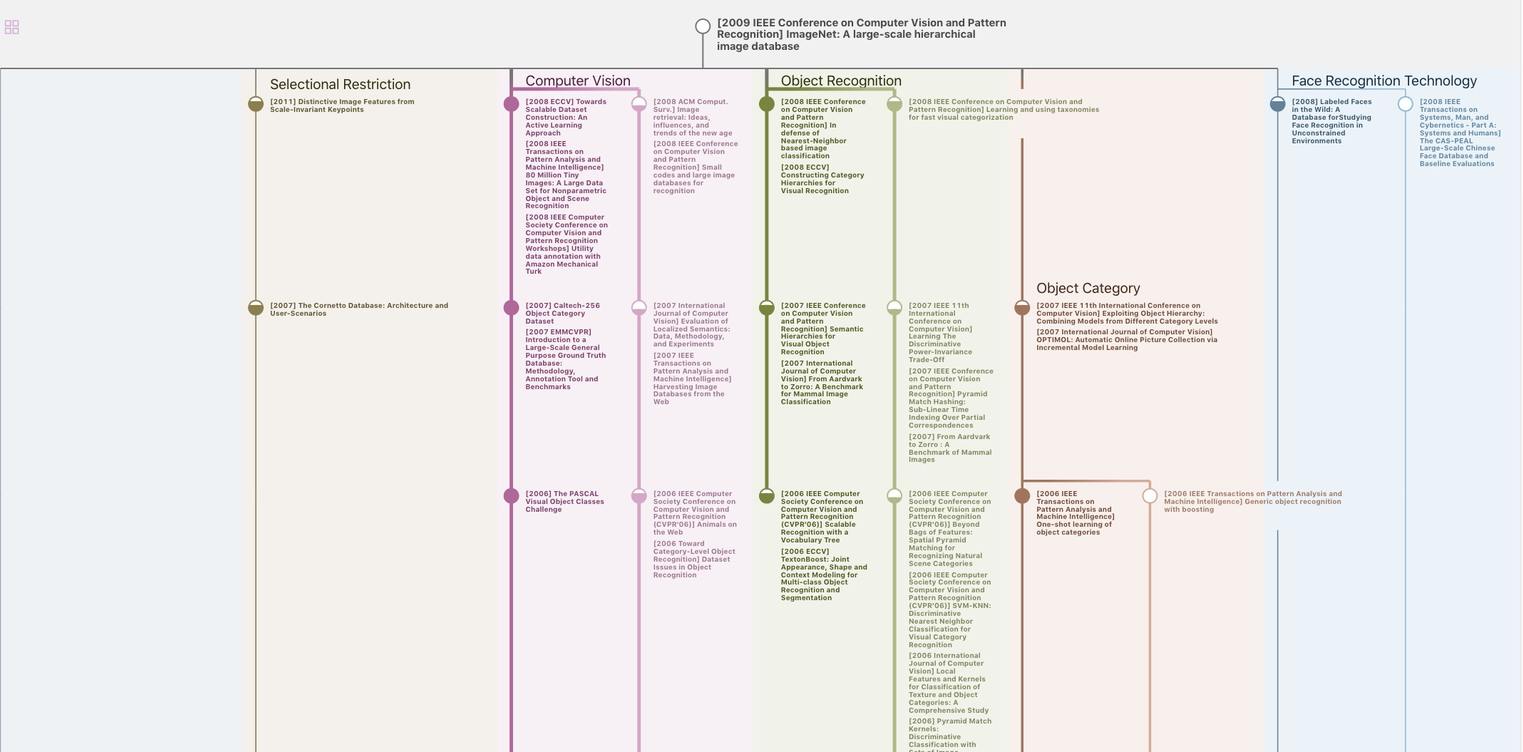
生成溯源树,研究论文发展脉络
Chat Paper
正在生成论文摘要