Predicting Stress and Providing Counterfactual Explanations: A Pilot Study on Caregivers
2023 11TH INTERNATIONAL CONFERENCE ON AFFECTIVE COMPUTING AND INTELLIGENT INTERACTION WORKSHOPS AND DEMOS, ACIIW(2023)
Abstract
Caregiving for spouses with Alzheimer's disease or related dementias (ADRD) is one of the most stressful experiences. Evidence-based treatments for caregivers who have a high risk of mental health issues are needed. In this study, we designed models for predicting changes in perceived stress scale (PSS) (increase/not increase) in one week and generated some examples of counterfactual ('what-if') explanations to change the stress state for helping manage stress. Using self-report (positive and negative affect and sleep quality) and sensor data (heart rate, sleep, and steps) collected in 132 week-long study sessions from 57 participants, we compared explainable PSS change prediction models (Random Forest, XGBoost, LightGBM, EBM, and Neural Network) along with 'what-if' explanations. First, we developed machine learning models for classifying the change in PSS scores before and after the session period. Second, we identified feature importance using our explainable models. Our results showed that XGBoost performed the best with an accuracy of 0.79 and an F1 score of 0.78 for predicting changes in perceived stress. Our results also showed that minimum heart rate, mean steps per day, and negative affect are the most predictive features. Our preliminary counterfactual examples about sleep parameters would be able to provide suggestions for improving one's health. We discussed our ideas to provide better suggestions using DiCE.
MoreTranslated text
Key words
caregiver,healthcare,XAI,DiCE,PSS
AI Read Science
Must-Reading Tree
Example
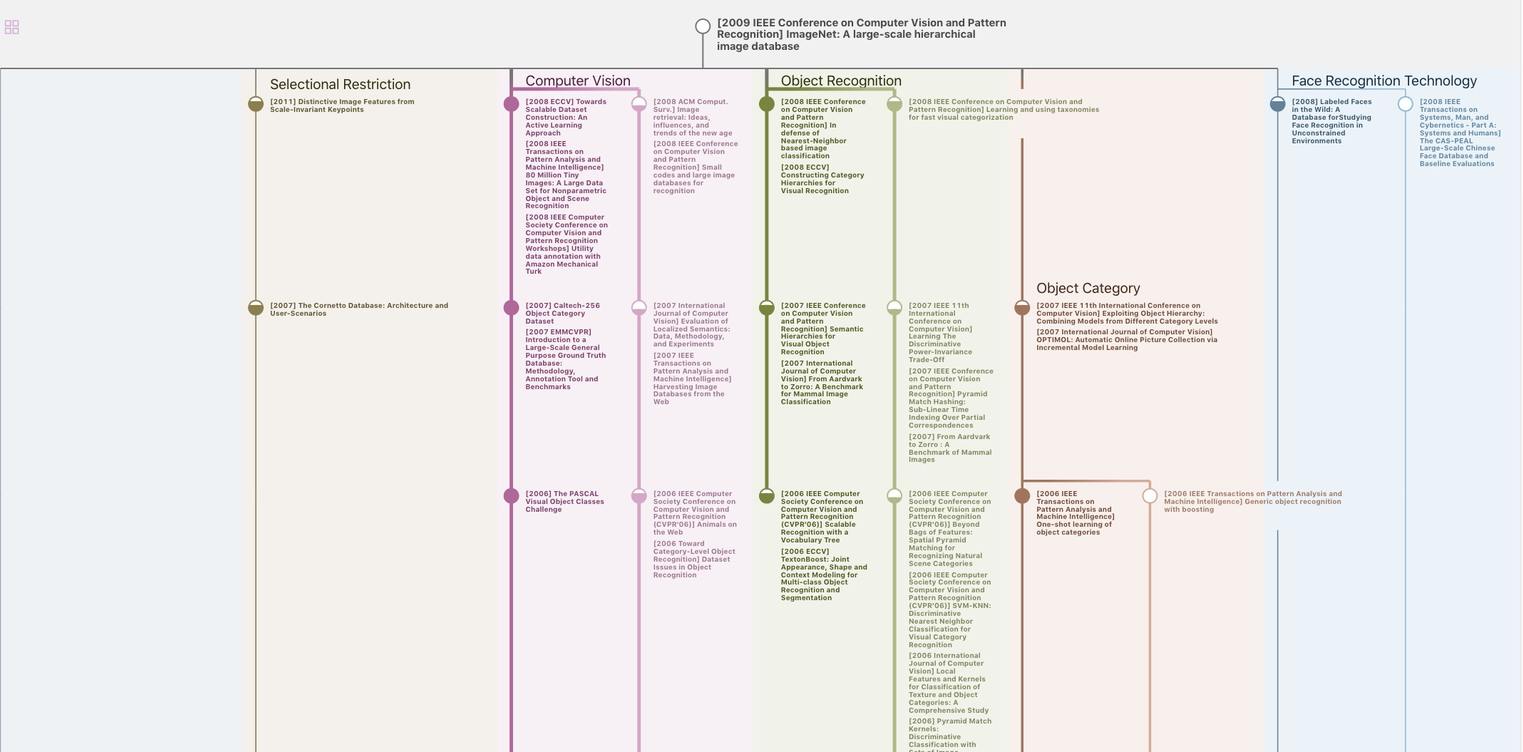
Generate MRT to find the research sequence of this paper
Chat Paper
Summary is being generated by the instructions you defined