Feature-Space Reinforcement Learning for Robotic Manipulation
2023 Fifth International Conference on Transdisciplinary AI (TransAI)(2023)
Abstract
Reinforcement Learning (RL) has gained popularity for developing intelligent robots, but challenges such as sample inefficiency and lack of generalization persist. The choice of observation space significantly influences RL algorithms' sample efficiency in robotics. While end-to-end learning has been emphasized, it increases complexity and inefficiency as the agent must re-learn forward and inverse kinematics. To address these issues, we propose a straightforward approach that utilizes readily available control techniques, such as forward and inverse kinematics, to capitalize on domain knowledge. Our approach involves enhancing the observation space with task-space features and utilizing task-space inverse kinematics. Our contributions include a proposal for mathematical formulation and a framework for RL algorithms in robotics.
MoreTranslated text
Key words
reinforcement learning,robotics application,task space representation
AI Read Science
Must-Reading Tree
Example
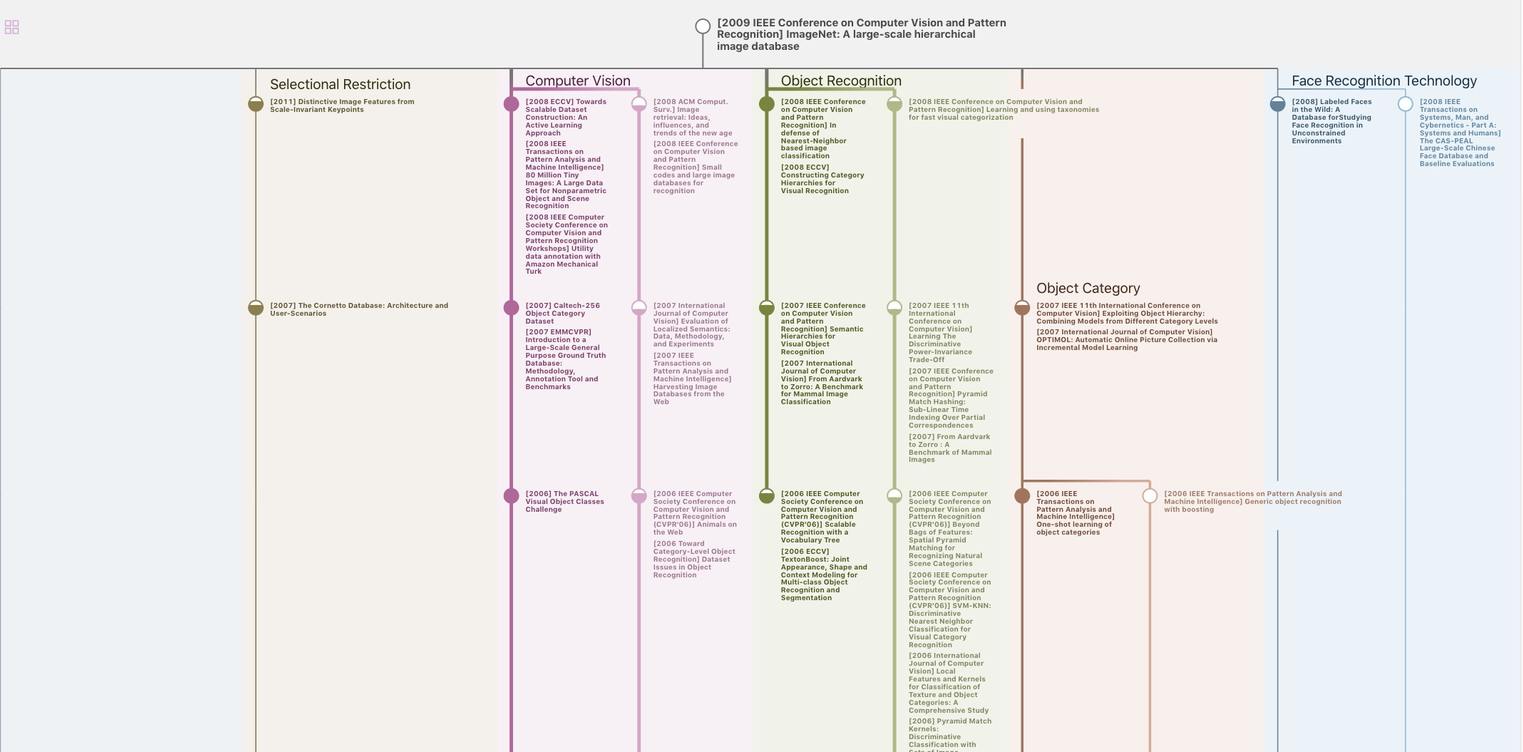
Generate MRT to find the research sequence of this paper
Chat Paper
Summary is being generated by the instructions you defined