ERTNet: an interpretable transformer-based framework for EEG emotion recognition
FRONTIERS IN NEUROSCIENCE(2024)
摘要
Background Emotion recognition using EEG signals enables clinicians to assess patients' emotional states with precision and immediacy. However, the complexity of EEG signal data poses challenges for traditional recognition methods. Deep learning techniques effectively capture the nuanced emotional cues within these signals by leveraging extensive data. Nonetheless, most deep learning techniques lack interpretability while maintaining accuracy.Methods We developed an interpretable end-to-end EEG emotion recognition framework rooted in the hybrid CNN and transformer architecture. Specifically, temporal convolution isolates salient information from EEG signals while filtering out potential high-frequency noise. Spatial convolution discerns the topological connections between channels. Subsequently, the transformer module processes the feature maps to integrate high-level spatiotemporal features, enabling the identification of the prevailing emotional state.Results Experiments' results demonstrated that our model excels in diverse emotion classification, achieving an accuracy of 74.23% +/- 2.59% on the dimensional model (DEAP) and 67.17% +/- 1.70% on the discrete model (SEED-V). These results surpass the performances of both CNN and LSTM-based counterparts. Through interpretive analysis, we ascertained that the beta and gamma bands in the EEG signals exert the most significant impact on emotion recognition performance. Notably, our model can independently tailor a Gaussian-like convolution kernel, effectively filtering high-frequency noise from the input EEG data.Discussion Given its robust performance and interpretative capabilities, our proposed framework is a promising tool for EEG-driven emotion brain-computer interface.
更多查看译文
关键词
EEG,emotion recognition,deep learning,transformer,interpretability
AI 理解论文
溯源树
样例
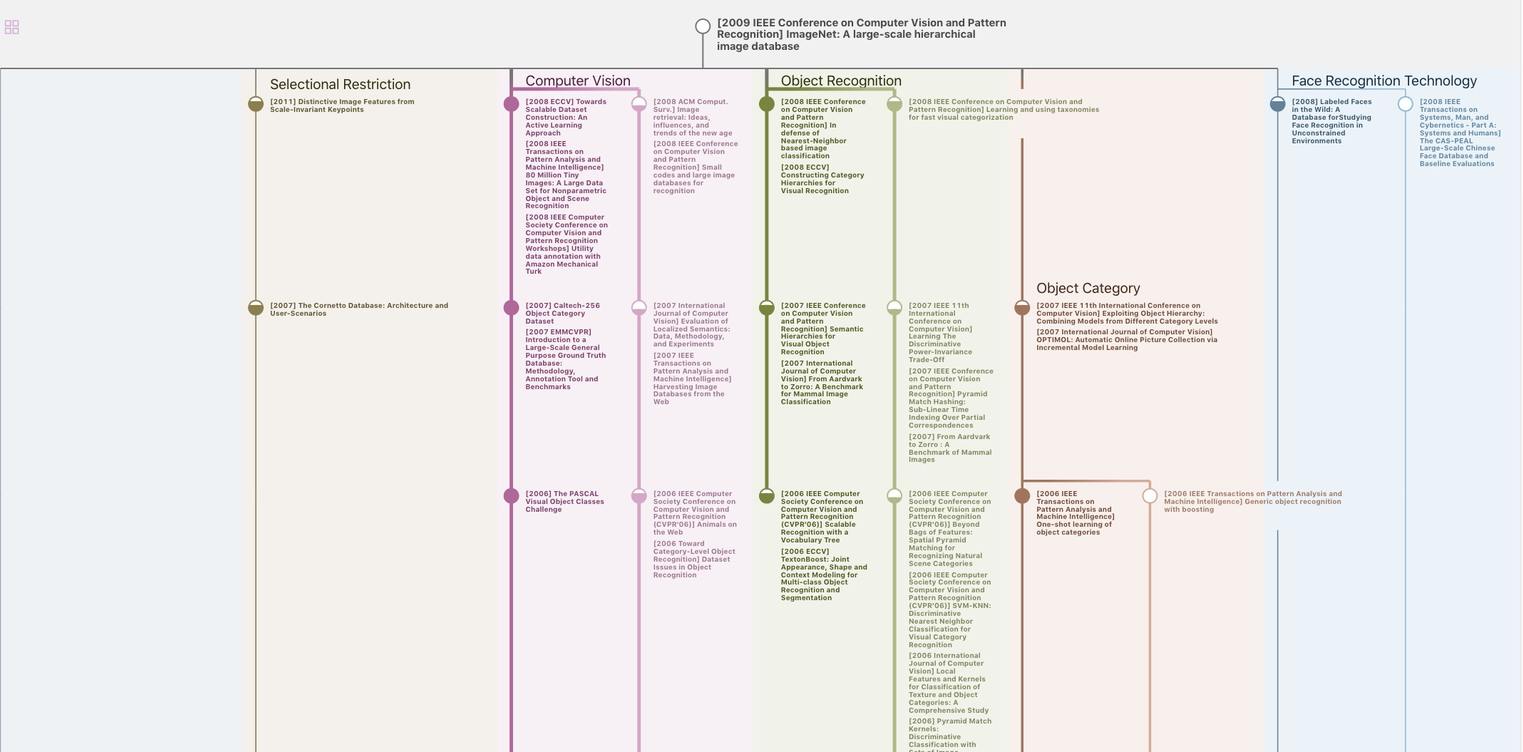
生成溯源树,研究论文发展脉络
Chat Paper
正在生成论文摘要