Discovering common information in multi-view data
Information Fusion(2024)
摘要
We introduce an innovative and mathematically rigorous definition for computing common information from multi-view data, drawing inspiration from Gács-Körner common information in information theory. Leveraging this definition, we develop a novel supervised multi-view learning framework to capture both common and unique information. By explicitly minimizing a total correlation term, the extracted common information and the unique information from each view are forced to be independent of each other, which, in turn, theoretically guarantees the effectiveness of our framework. To estimate information-theoretic quantities, our framework employs matrix-based Rényi’s α-order entropy functional, which forgoes the need for variational approximation and distributional estimation in high-dimensional space. Theoretical proof is provided that our framework can faithfully discover both common and unique information from multi-view data. Experiments on synthetic and seven benchmark real-world datasets demonstrate the superior performance of our proposed framework over state-of-the-art approaches.
更多查看译文
关键词
Multi-view learning,Common information,Matrix-based Rényi’s α-order entropy functional,Total correlation
AI 理解论文
溯源树
样例
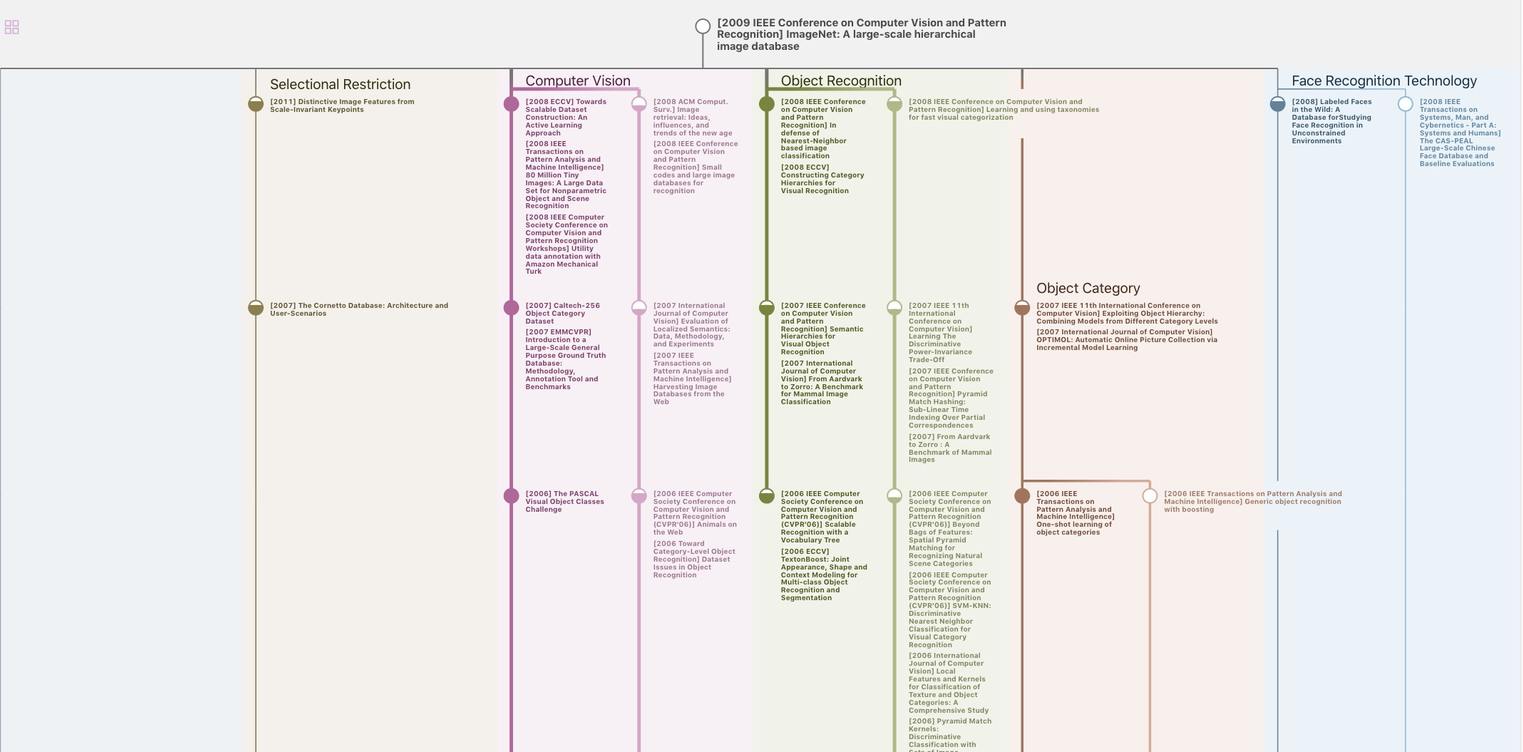
生成溯源树,研究论文发展脉络
Chat Paper
正在生成论文摘要