Explaining Time Series via Contrastive and Locally Sparse Perturbations
CoRR(2024)
摘要
Explaining multivariate time series is a compound challenge, as it requires
identifying important locations in the time series and matching complex
temporal patterns. Although previous saliency-based methods addressed the
challenges, their perturbation may not alleviate the distribution shift issue,
which is inevitable especially in heterogeneous samples. We present ContraLSP,
a locally sparse model that introduces counterfactual samples to build
uninformative perturbations but keeps distribution using contrastive learning.
Furthermore, we incorporate sample-specific sparse gates to generate more
binary-skewed and smooth masks, which easily integrate temporal trends and
select the salient features parsimoniously. Empirical studies on both synthetic
and real-world datasets show that ContraLSP outperforms state-of-the-art
models, demonstrating a substantial improvement in explanation quality for time
series data. The code is available for review:
https://anonymous.4open.science/r/ContraLSP-1146/
更多查看译文
关键词
time series,explainability,perturbation
AI 理解论文
溯源树
样例
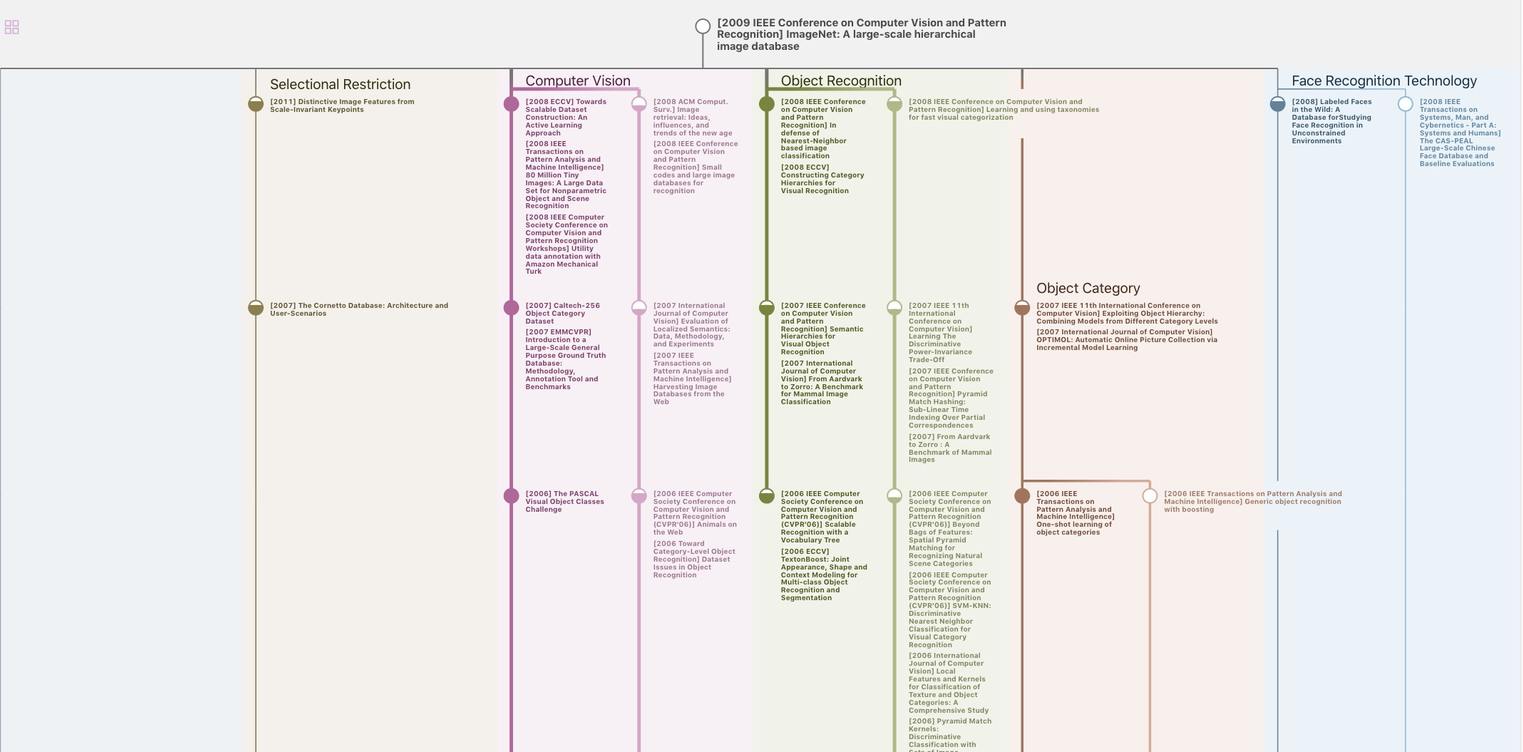
生成溯源树,研究论文发展脉络
Chat Paper
正在生成论文摘要