Contrastive Preference Optimization: Pushing the Boundaries of LLM Performance in Machine Translation
ICML 2024(2024)
Abstract
Moderate-sized large language models (LLMs) -- those with 7B or 13B parameters -- exhibit promising machine translation (MT) performance. However, they do not match the performance of state-of-the-art conventional encoder-decoder translation models or larger-scale LLMs such as GPT-4. In this study, we bridge this performance gap. We first assess the shortcomings of supervised fine-tuning for LLMs in the MT task, emphasizing the quality issues present in the reference data, despite being human-generated. Then, in contrast to supervised fine-tuning which mimics reference translations, we introduce Contrastive Preference Optimization (CPO), a novel approach that trains models to avoid generating adequate but not perfect translations. Applying CPO to ALMA models with only 22K parallel sentences and 0.1% parameters yields significant improvements. The resulting model, called ALMA-R, can match or exceed the performance of the WMT competition winners and GPT-4 on WMT'21, WMT'22 and WMT'23 test datasets.
MoreTranslated text
AI Read Science
Must-Reading Tree
Example
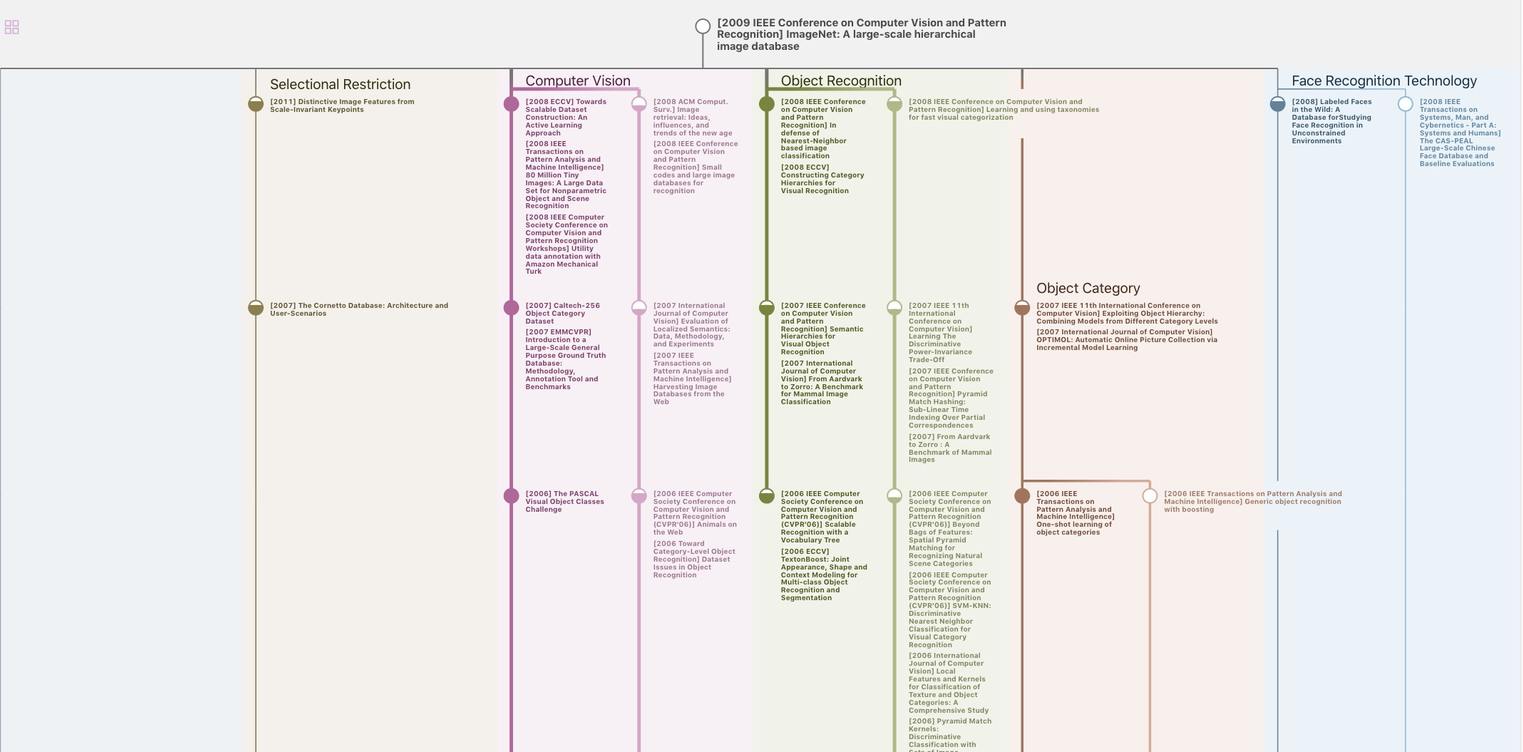
Generate MRT to find the research sequence of this paper
Chat Paper
Summary is being generated by the instructions you defined