Efficient Nonparametric Tensor Decomposition for Binary and Count Data
AAAI 2024(2024)
摘要
In numerous applications, binary reactions or event counts are observed and stored within high-order tensors. Tensor decompositions (TDs) serve as a powerful tool to handle such high-dimensional and sparse data. However, many traditional TDs are explicitly or implicitly designed based on the Gaussian distribution, which is unsuitable for discrete data. Moreover, most TDs rely on predefined multi-linear structures, such as CP and Tucker formats. Therefore, they may not be effective enough to handle complex real-world datasets. To address these issues, we propose ENTED, an Efficient Nonparametric TEnsor Decomposition for binary and count tensors. Specifically, we first employ a nonparametric Gaussian process (GP) to replace traditional multi-linear structures. Next, we utilize the Pólya-Gamma augmentation which provides a unified framework to establish conjugate models for binary and count distributions. Finally, to address the computational issue of GPs, we enhance the model by incorporating sparse orthogonal variational inference of inducing points, which offers a more effective covariance approximation within GPs and stochastic natural gradient updates for nonparametric models. We evaluate our model on several real-world tensor completion tasks, considering binary and count datasets. The results manifest both better performance and computational advantages of the proposed model.
更多查看译文
关键词
ML: Matrix & Tensor Methods,ML: Bayesian Learning,ML: Dimensionality Reduction/Feature Selection
AI 理解论文
溯源树
样例
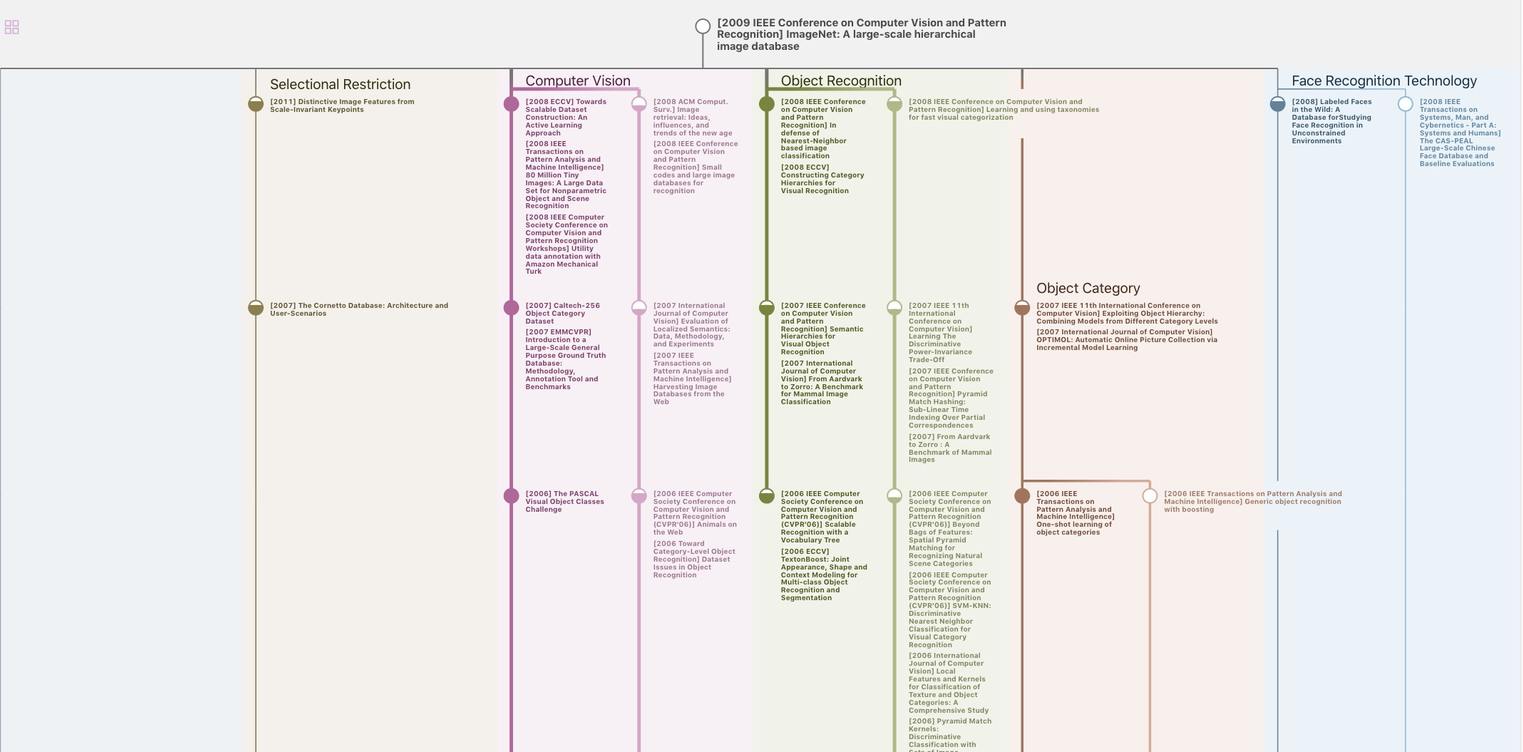
生成溯源树,研究论文发展脉络
Chat Paper
正在生成论文摘要