Rapid Estimation of Left Ventricular Contractility with a Physics-Informed Neural Network Inverse Modeling Approach
CoRR(2024)
摘要
Physics-based computer models based on numerical solution of the governing
equations generally cannot make rapid predictions, which in turn, limits their
applications in the clinic. To address this issue, we developed a
physics-informed neural network (PINN) model that encodes the physics of a
closed-loop blood circulation system embedding a left ventricle (LV). The PINN
model is trained to satisfy a system of ordinary differential equations (ODEs)
associated with a lumped parameter description of the circulatory system. The
model predictions have a maximum error of less than 5
obtained by solving the ODEs numerically. An inverse modeling approach using
the PINN model is also developed to rapidly estimate model parameters (in
∼ 3 mins) from single-beat LV pressure and volume waveforms. Using
synthetic LV pressure and volume waveforms generated by the PINN model with
different model parameter values, we show that the inverse modeling approach
can recover the corresponding ground truth values, which suggests that the
model parameters are unique. The PINN inverse modeling approach is then applied
to estimate LV contractility indexed by the end-systolic elastance E_es
using waveforms acquired from 11 swine models, including waveforms acquired
before and after administration of dobutamine (an inotropic agent) in 3
animals. The estimated E_es is about 58
associated with dobutamine compared to those without, which implies that this
approach can be used to estimate LV contractility using single-beat
measurements. The PINN inverse modeling can potentially be used in the clinic
to simultaneously estimate LV contractility and other physiological parameters
from single-beat measurements.
更多查看译文
AI 理解论文
溯源树
样例
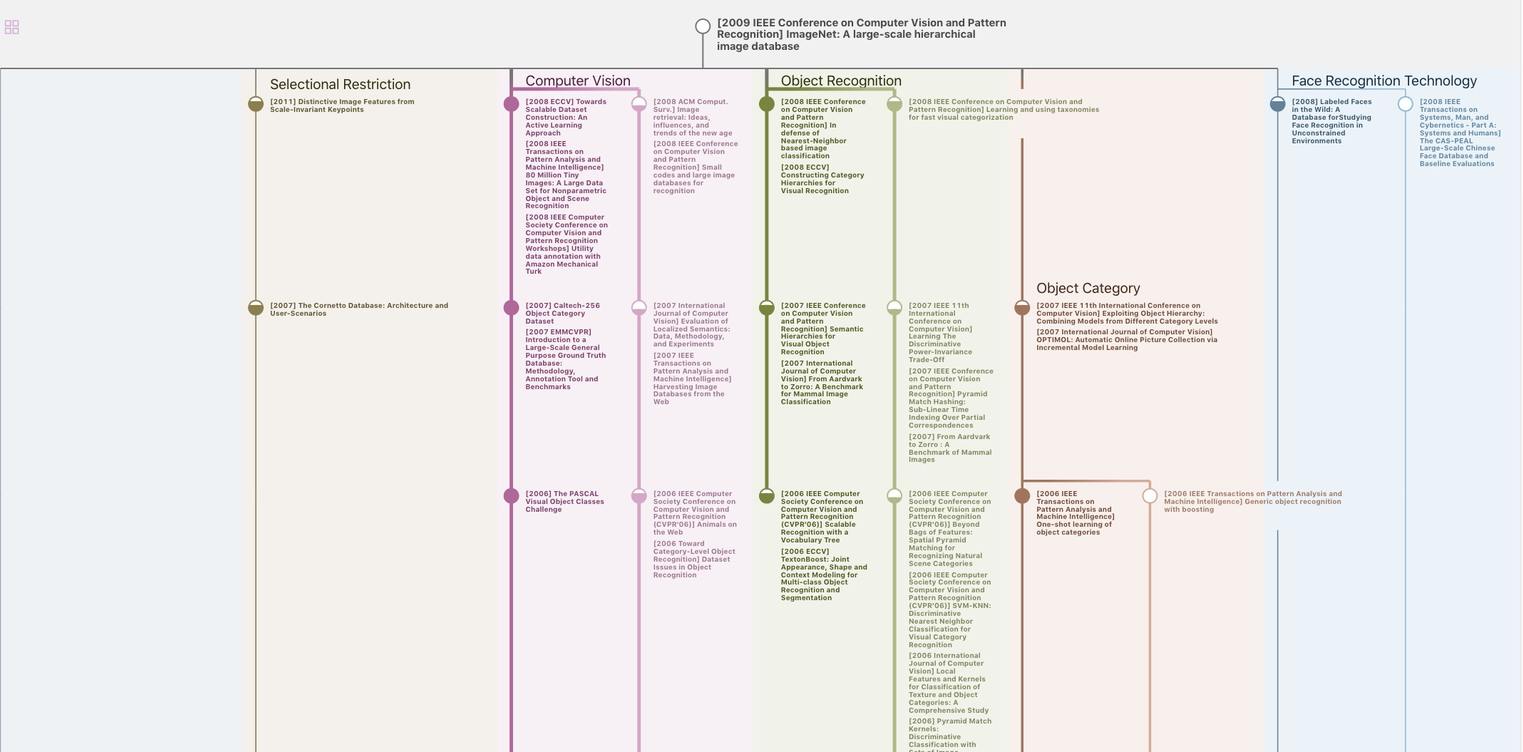
生成溯源树,研究论文发展脉络
Chat Paper
正在生成论文摘要