Bayesian Signal Matching for Transfer Learning in ERP-Based Brain Computer Interface
arxiv(2024)
摘要
An Event-Related Potential (ERP)-based Brain-Computer Interface (BCI) Speller
System assists people with disabilities communicate by decoding
electroencephalogram (EEG) signals. A P300-ERP embedded in EEG signals arises
in response to a rare, but relevant event (target) among a series of irrelevant
events (non-target). Different machine learning methods have constructed binary
classifiers to detect target events, known as calibration. Existing calibration
strategy only uses data from participants themselves with lengthy training
time, causing biased P300 estimation and decreasing prediction accuracy. To
resolve this issue, we propose a Bayesian signal matching (BSM) framework for
calibrating the EEG signals from a new participant using data from source
participants. BSM specifies the joint distribution of stimulus-specific EEG
signals among source participants via a Bayesian hierarchical mixture model. We
apply the inference strategy: if source and new participants are similar, they
share the same set of model parameters, otherwise, they keep their own sets of
model parameters; we predict on the testing data using parameters of the
baseline cluster directly. Our hierarchical framework can be generalized to
other base classifiers with clear likelihood specifications. We demonstrate the
advantages of BSM using simulations and focus on the real data analysis among
participants with neuro-degenerative diseases.
更多查看译文
AI 理解论文
溯源树
样例
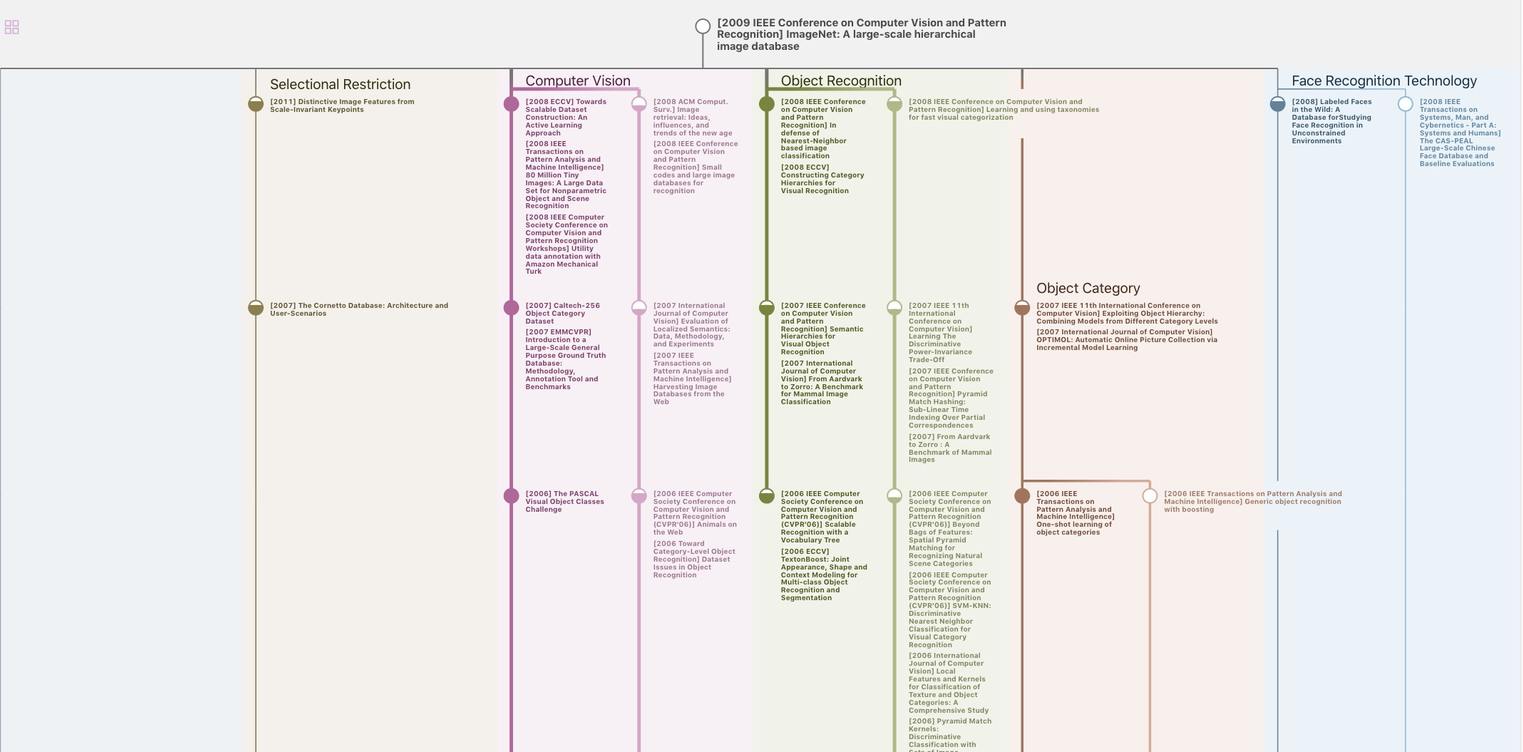
生成溯源树,研究论文发展脉络
Chat Paper
正在生成论文摘要