Automatic Implementation of Neural Networks through Reaction Networks–Part II: Error Analysis
arxiv(2024)
摘要
This paired article aims to develop an automated and programmable biochemical
fully connected neural network (BFCNN) with solid theoretical support. In Part
I, a concrete design for BFCNN is presented, along with the validation of the
effectiveness and exponential convergence of computational modules. In this
article, we establish the framework for specifying the realization errors by
monitoring the errors generated from approaching equilibrium points in
individual modules, as well as their vertical propagation from upstream modules
and horizontal accumulation from previous iterations. Ultimately, we derive the
general error upper bound formula for any iteration and illustrate its
exponential convergence order with respect to the phase length of the utilized
chemical oscillator. The numerical experiments, based on the classification
examples, reveal the tendency of total errors related to both the phase length
and iteration number.
更多查看译文
AI 理解论文
溯源树
样例
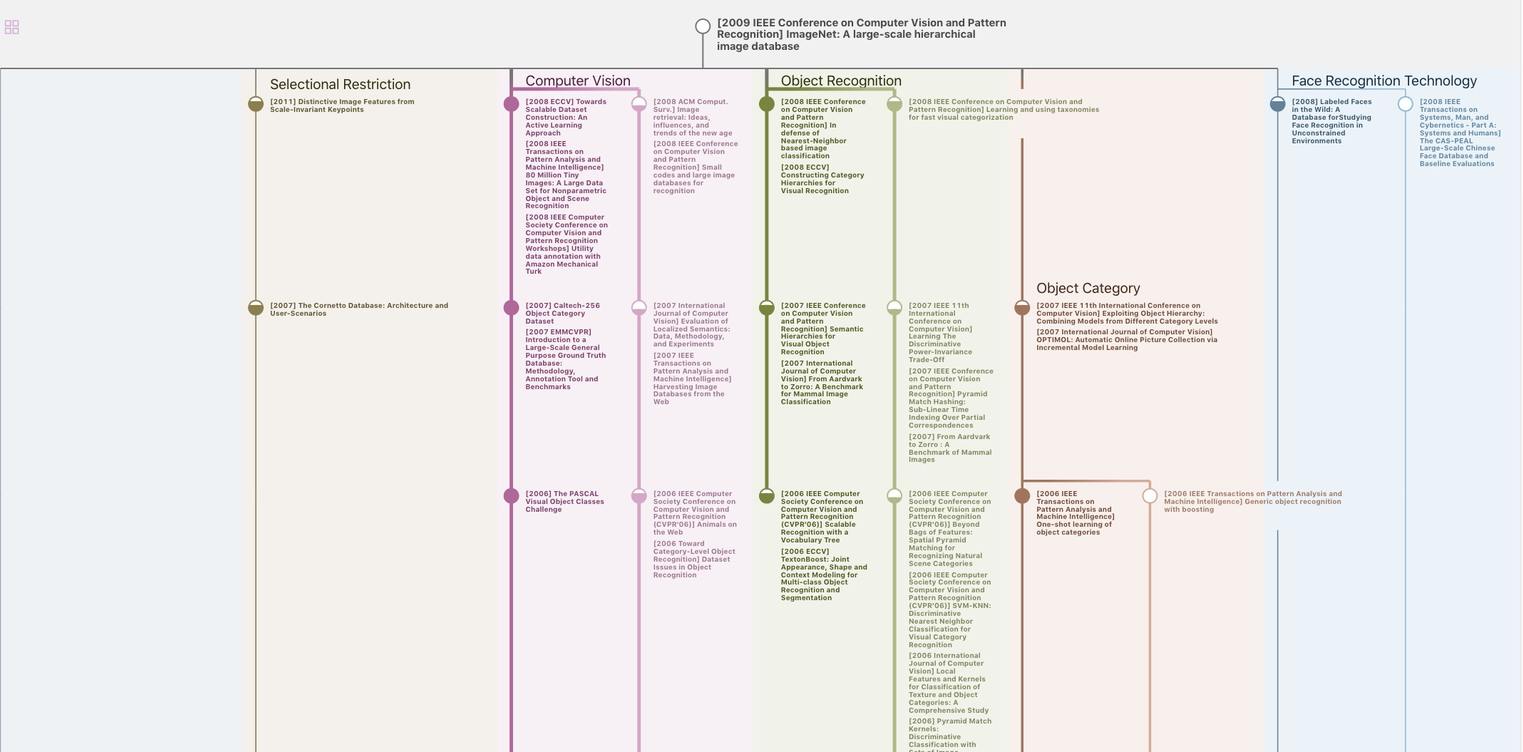
生成溯源树,研究论文发展脉络
Chat Paper
正在生成论文摘要