Simulation-based Inference of Deep Fields: Galaxy Population Model and Redshift Distributions
JOURNAL OF COSMOLOGY AND ASTROPARTICLE PHYSICS(2024)
Key words
galaxy surveys,galaxy evolution,Bayesian reasoning,cosmological simulations
AI Read Science
Must-Reading Tree
Example
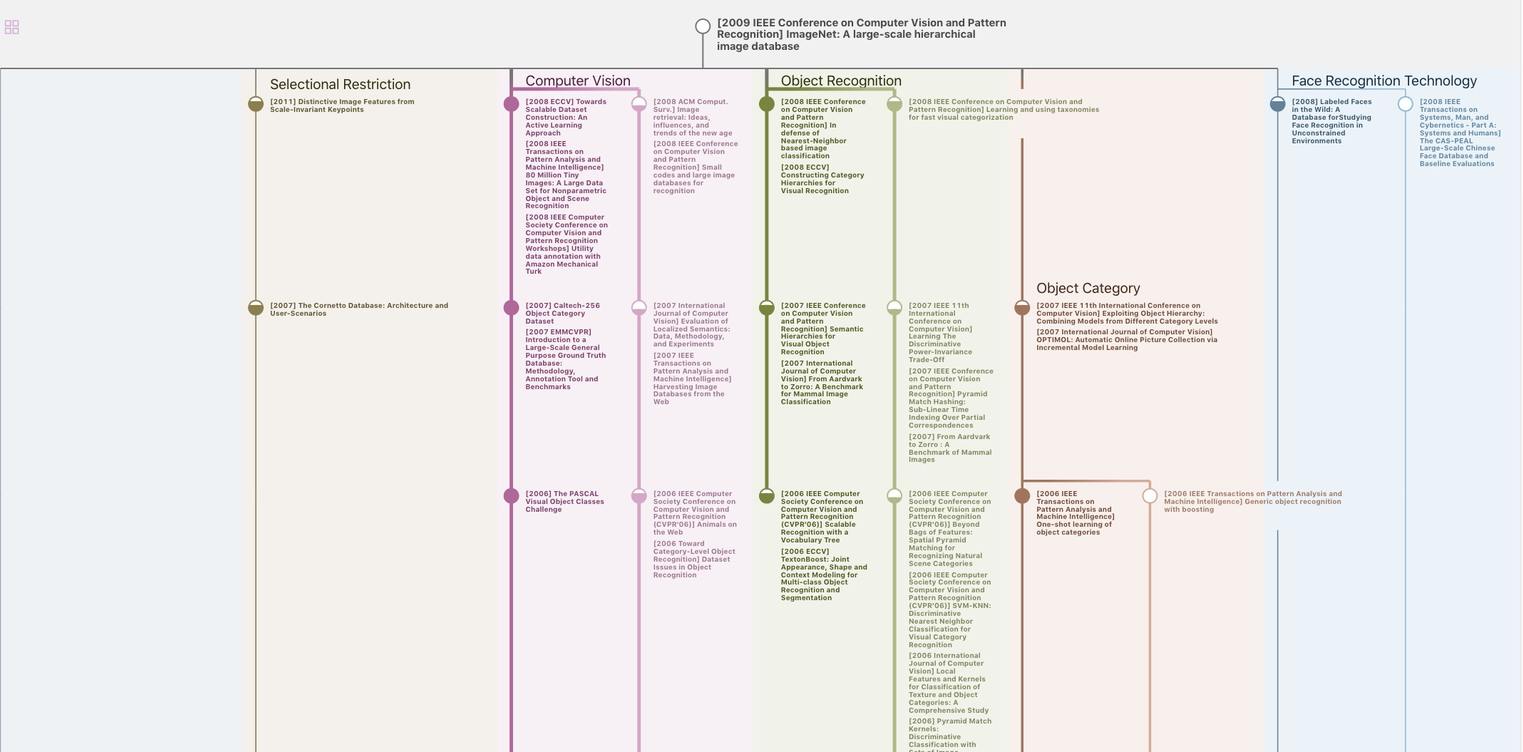
Generate MRT to find the research sequence of this paper
Chat Paper
Summary is being generated by the instructions you defined