AugSumm: towards generalizable speech summarization using synthetic labels from large language model
CoRR(2024)
摘要
Abstractive speech summarization (SSUM) aims to generate human-like summaries
from speech. Given variations in information captured and phrasing, recordings
can be summarized in multiple ways. Therefore, it is more reasonable to
consider a probabilistic distribution of all potential summaries rather than a
single summary. However, conventional SSUM models are mostly trained and
evaluated with a single ground-truth (GT) human-annotated deterministic summary
for every recording. Generating multiple human references would be ideal to
better represent the distribution statistically, but is impractical because
annotation is expensive. We tackle this challenge by proposing AugSumm, a
method to leverage large language models (LLMs) as a proxy for human annotators
to generate augmented summaries for training and evaluation. First, we explore
prompting strategies to generate synthetic summaries from ChatGPT. We validate
the quality of synthetic summaries using multiple metrics including human
evaluation, where we find that summaries generated using AugSumm are perceived
as more valid to humans. Second, we develop methods to utilize synthetic
summaries in training and evaluation. Experiments on How2 demonstrate that
pre-training on synthetic summaries and fine-tuning on GT summaries improves
ROUGE-L by 1 point on both GT and AugSumm-based test sets. AugSumm summaries
are available at https://github.com/Jungjee/AugSumm.
更多查看译文
关键词
Speech summarization,synthetic summary,large language model,data augmentation,ChatGPT
AI 理解论文
溯源树
样例
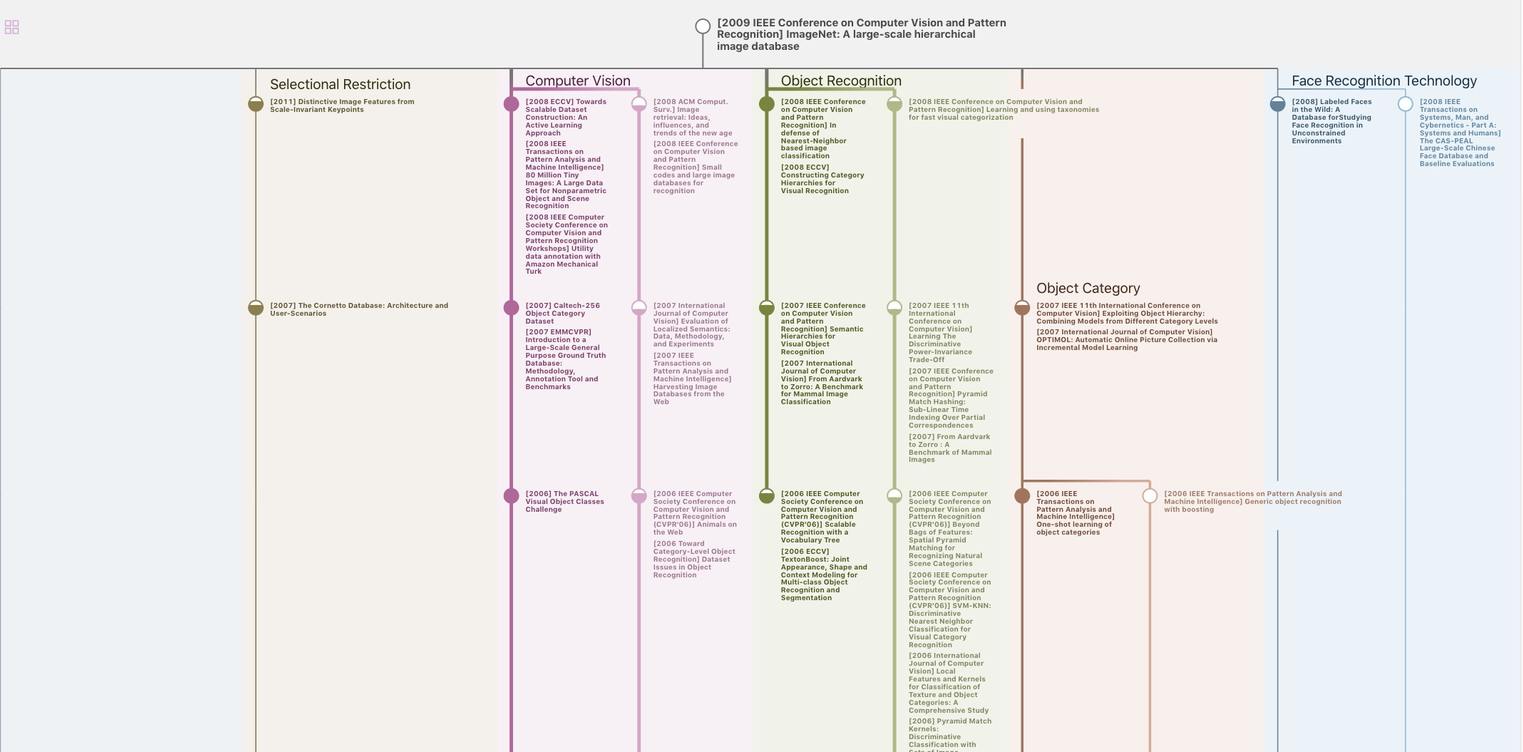
生成溯源树,研究论文发展脉络
Chat Paper
正在生成论文摘要