Examining the synergistic effects through machine learning prediction and optimisation in the anaerobic Co-digestion (ACoD) of palm oil mill effluent (POME) and decanter cake (DC) with economic analysis
JOURNAL OF CLEANER PRODUCTION(2024)
摘要
The anaerobic co-digestion (ACoD) of palm oil mill effluent (POME) and decanter cake (DC) is gaining considerable attention as it helps to overcome insufficient feedstock issues in anaerobic digesters during low crop seasons. However, the ACoD process involves complex non-linear relationships between input parameters and process outcomes, posing challenges in accurately evaluating the synergistic effects and optimising biogas production on an industrial scale using traditional modelling approaches. In this study, three supervised machine learning algorithms, i.e., Random Forest (RF), Artificial Neural Network (ANN) and Elastic Net (EN) are applied to model the biogas production and COD removal from a biogas plant. These algorithms are used to learn and uncover the intricate interdependencies between input variables (e.g., temperature, organic loading rate, dilution ratio) and resulting synergistic effects on output variables (biogas production and COD removal) through their non-linear modelling capabilities. Results demonstrate that the Random Forest (RF) model provides the most accurate predictions for biogas production and COD removal, with a high coefficient of determination (R-2) of 0.9961 and the lowest root mean square error (RMSE) of 0.110. Optimal conditions for the anaerobic digester, including a temperature of 41.4 degrees C, an organic loading rate (OLR) of 0.87 kg COD/m(3)day, and a dilution ratio of 1:10, led to a 14.9% improvement in biogas production (corresponding methane yield of 0.3 Nm(3) CH4/kgCOD(removed)) and a 17.5% increment in COD removal efficiency (88.2%). Feature importance analysis revealed that the dilution ratio significantly influenced biogas production, while OLR played a dominant role in COD removal. Future research efforts will focus on implementing pre-treatment methods for DC in the ACoD system to further enhance biogas production.
更多查看译文
关键词
Palm oil mill effluent (POME),Decanter cake (DC),Random forest (RF),Artificial neural network (ANN),Elastic net (EN)
AI 理解论文
溯源树
样例
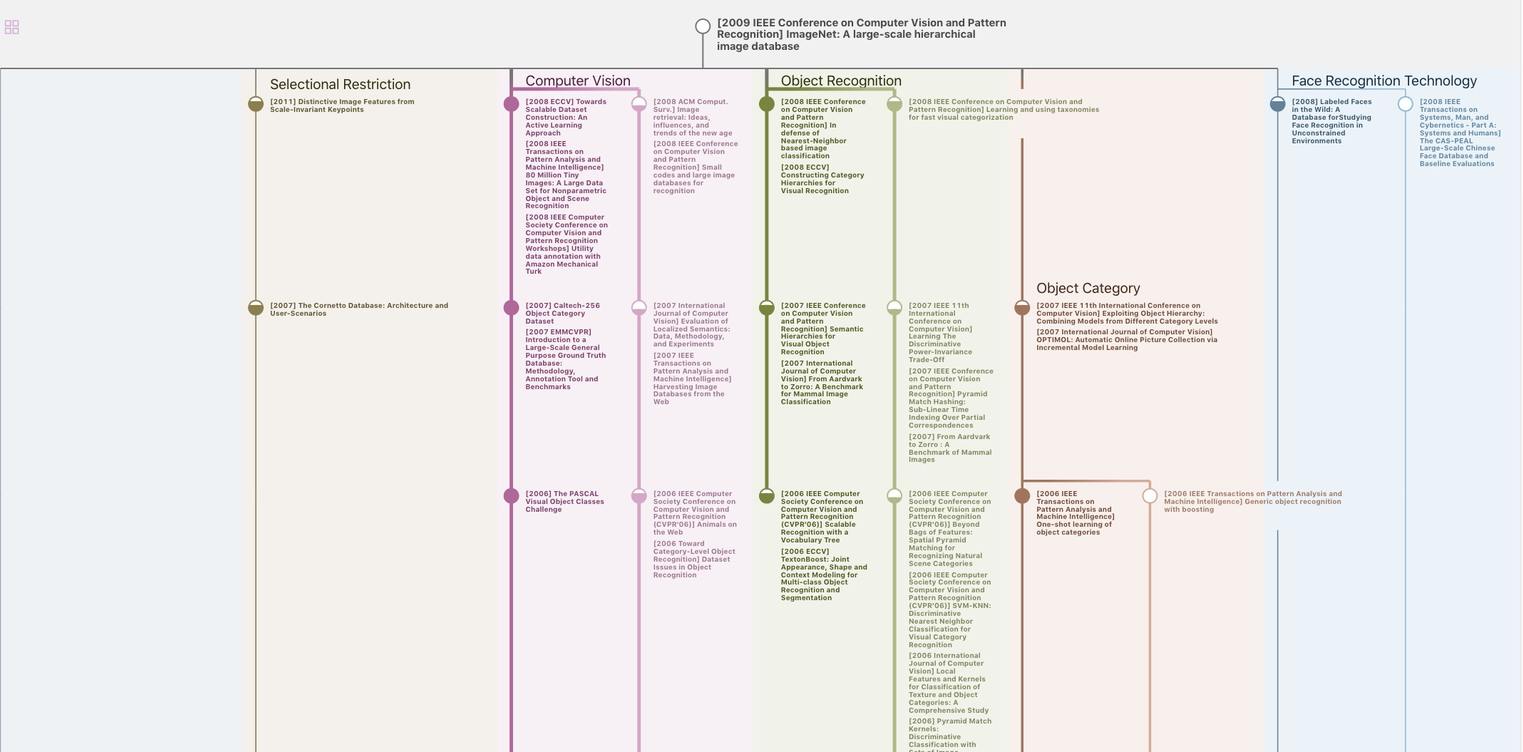
生成溯源树,研究论文发展脉络
Chat Paper
正在生成论文摘要