Estimation of sequential transient flow around cylinders using recurrent neural network coupled graph convolutional network
OCEAN ENGINEERING(2024)
摘要
This paper develops a novel reduced-order model called SGCNN, which combines the Graph Convolutional Networks (GCN) with the Recurrent Neural Networks (RNN), to predict sequential transient flow around cylinders using unstructured flow field data. In flow field prediction, we use GCN's unique message passing mechanism to extract and aggregate the characteristics of the flow field grid in non-Euclidean space, eliminating the pixelization process of CNN in non-Euclidean space, and significantly improving the prediction accuracy. GRU is used as the unit of the RNN network to obtain the changeable physical characteristics through the hidden layer, thereby achieving continuous prediction of the flow field. According to our literature review, this is the first investigation to estimate sequential transient flow fields around cylinders. The proposed model is initially verified with the flow around single cylinder. Taking the numerical simulation as the reference, the relative errors of the predicted sequential velocity and the pressure fields are less than 3% and 2%, respectively. Subsequently, the complexity of the investigation is increased by varying the Reynolds number and introducing additional cylinders in the flow region. The predicted physical fields consistently align well with CFD simulations, and the computational time is remarkably reduced by more than 90%.
更多查看译文
关键词
Computational fluid dynamics,GCN,RNN,Dimensionality reduction,Flow field prediction
AI 理解论文
溯源树
样例
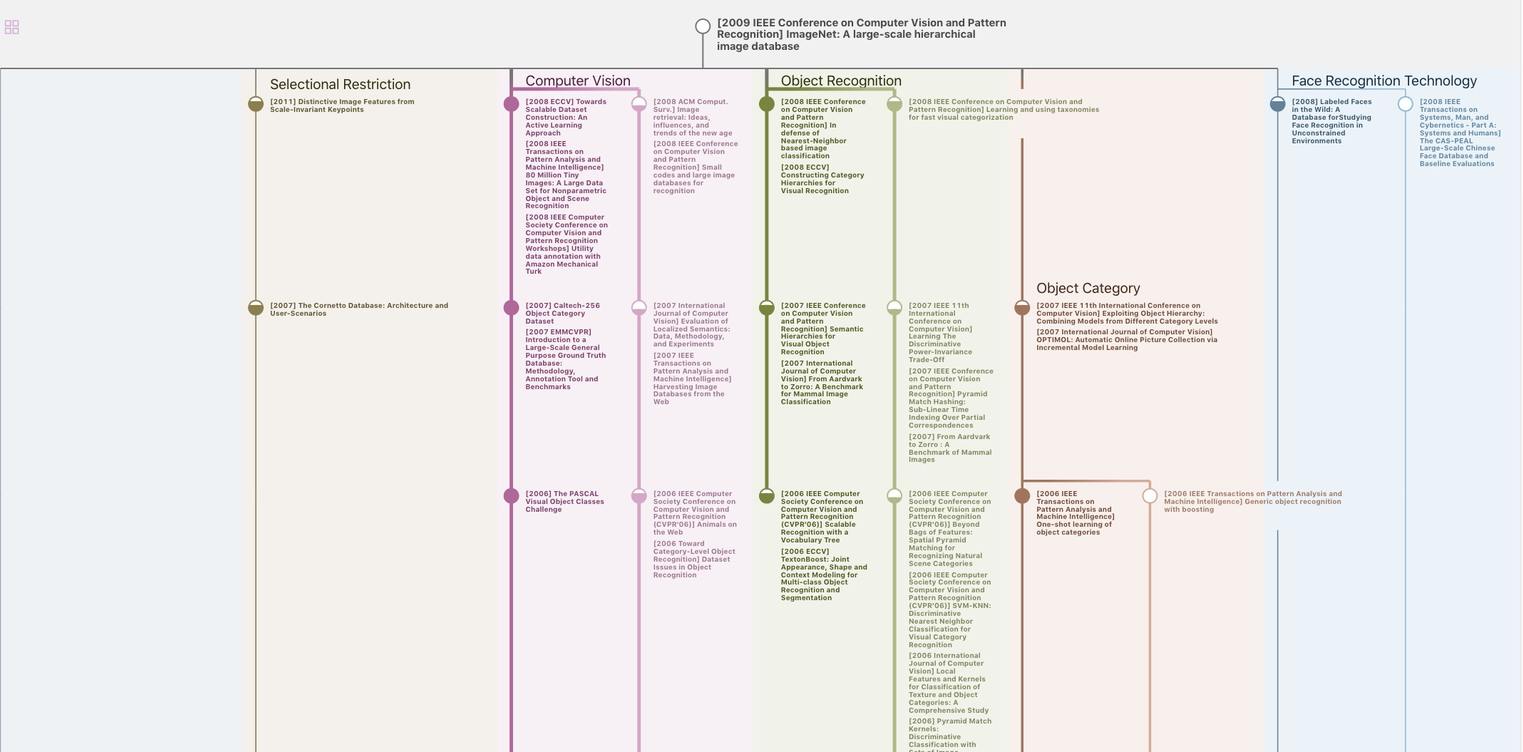
生成溯源树,研究论文发展脉络
Chat Paper
正在生成论文摘要