Robust autoencoder feature selector for unsupervised feature selection
INFORMATION SCIENCES(2024)
摘要
Unsupervised Feature Selection (UFS) methods are known to produce models with excellent ability to select high -quality features. This advantage, however, is challenged when analyzing noisy real -world data, where anomalies are prevalent. For example, there may be a feature (anomalous feature) that is corrupted across many samples or a sample (anomalous sample) that has more corruptions than its peers. Previous literature focused on addressing anomalous samples, and methods that are robust to both types of anomalies have been under -explored. This paper proposes a novel general framework for reconstruction -based UFS methods, which can be embedded into the feature learning process to simultaneously remove anomalous samples and features. Specifically, the framework learns double binary weight vectors to assign 0 weights to samples or features with the highest reconstruction errors and 1 weights to the others when computing reconstruction errors. By discarding the 0 -weighted samples and features when updating the model parameters, the anomalies in the data are excluded. This allows the model to focus more on learning from the clean part of the noisy data. Our proposed framework is then integrated with AutoEncoder Feature Selector (AEFS [10]) to develop a new method, which jointly performs anomaly removal and feature selection. The experimental results demonstrate the effectiveness of the proposed framework. Particularly, processing both types of anomalies provides better robustness than processing only one type. Moreover, our proposed method outperforms several state-of-the-art methods on various real -world datasets.
更多查看译文
关键词
Unsupervised feature selection,Auto-encoder,Anomaly detection,Robustness,Unsupervised learning
AI 理解论文
溯源树
样例
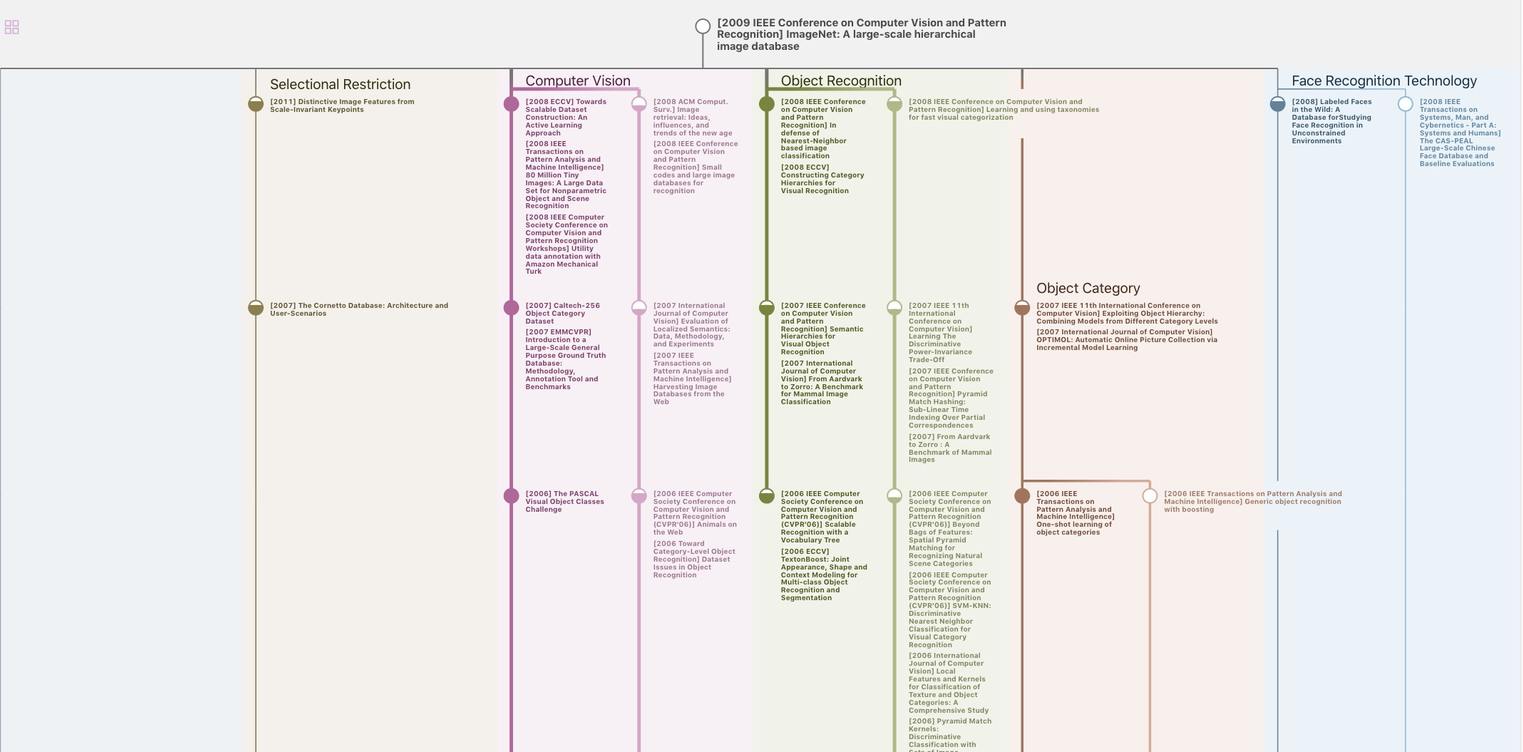
生成溯源树,研究论文发展脉络
Chat Paper
正在生成论文摘要