SOML: Structure-Wise Ordinal Measure Learning for Hyperspectral Image Classification.
IEEE Transactions on Instrumentation and Measurement(2024)
摘要
The hyperspectral image (HSI) classification has achieved the great successes in many practical applications. In this article, we propose a novel structure-wise ordinal measure-based learning method (SOML) for the HSI classification. Specifically, the ordinal measure (OM) tool is used to construct the feature representation model, which yields the ordinal features reflecting the spectral direction information of the HSI. Additionally, the selected support vector machine (SVM) classifier is integrated into such feature representation model, which aims to guarantee the consistency between ordinal features and the SVM classifier. Further, the
$\epsilon $
-dragging technique and low-rank representation are introduced for capturing the structure information of the HSI, which encourage the output ordinal features to be more separable and discriminative. We also design a proximal alternating optimization strategy to solve the constructed SOML model, and thus the optimal projection can be learned to map all samples into the ordinal features. The SVM is further used to classify these features to predict the results. Different from traditional classification methods, our proposed SOML method fully considers the information utilization of the HSI, relationship with the selected SVM classifier, and distribution of features in the stage of feature extraction, which further improve the discrimination and separability. Extensive experiments have been given to validate that the proposed SOML method achieves more competitive classification performance compared with several state-of-the-art algorithms.
更多查看译文
关键词
Classification,hyperspectral image (HSI),ordinal measure (OM),structure-wise information,support vector machine (SVM)
AI 理解论文
溯源树
样例
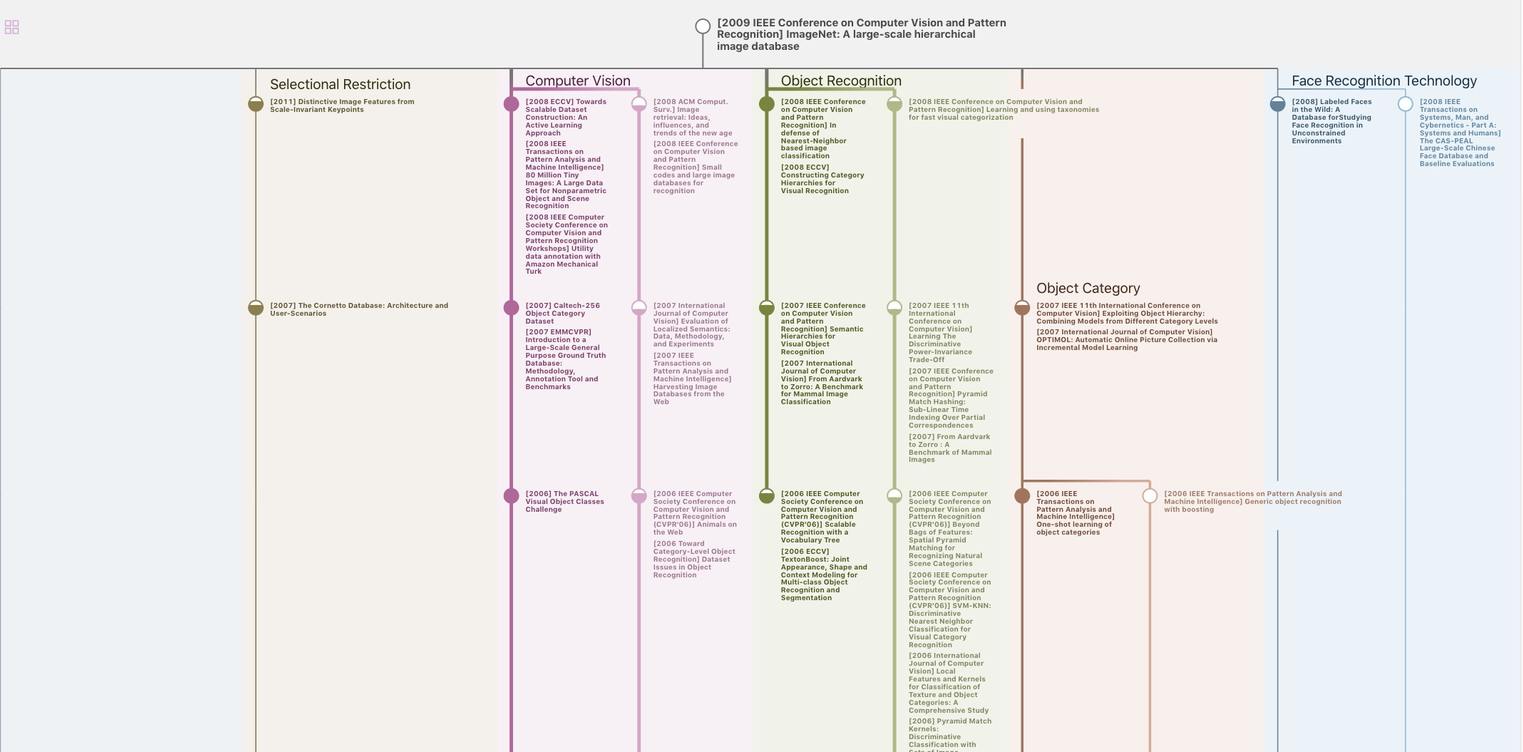
生成溯源树,研究论文发展脉络
Chat Paper
正在生成论文摘要