Privacy-Preserving Federated Class-Incremental Learning
IEEE Transactions on Machine Learning in Communications and Networking(2024)
摘要
Federated Learning (FL) offers a collaborative training framework, aggregating model parameters from decentralized clients. Many existing models, however, assume static, predetermined data classes within FLa frequently unrealistic assumption. Real-time data additions from clients can degrade global model recognition of established classes due to catastrophic forgetting. This is exacerbated when new clients, unfamiliar to previous participants, join sporadically. Additionally, there’s an imperative for client data privacy. Addressing these, we propose the Privacy-Preserving Federated Class-Incremental Learning (PP-FCIL) approach. This methodology ensures content-level privacy and significantly alleviates the risk of catastrophic forgetting in FCIL. To our knowledge, this is the first research seeks to embed differential privacy into the FCIL settings. Specifically, we introduce a dual-model structure that uses adaptive fusion of new and old knowledge to obtain a new global model. We also propose a multi-factor dynamic weighted aggregation strategy that considers several factors, such as data imbalance timeliness of the model, to speed up global model aggregation and accuracy. For privacy protection, we use Bayesian differential privacy to provide more balanced privacy protection for different datasets. Finally, we conducted experiments on CIFAR-100 and ImageNet to compare our method with other methods and verify its superiority.
更多查看译文
关键词
Federated learning,class-incremental learning,catastrophic forgetting,local differential privacy,dynamic aggregation
AI 理解论文
溯源树
样例
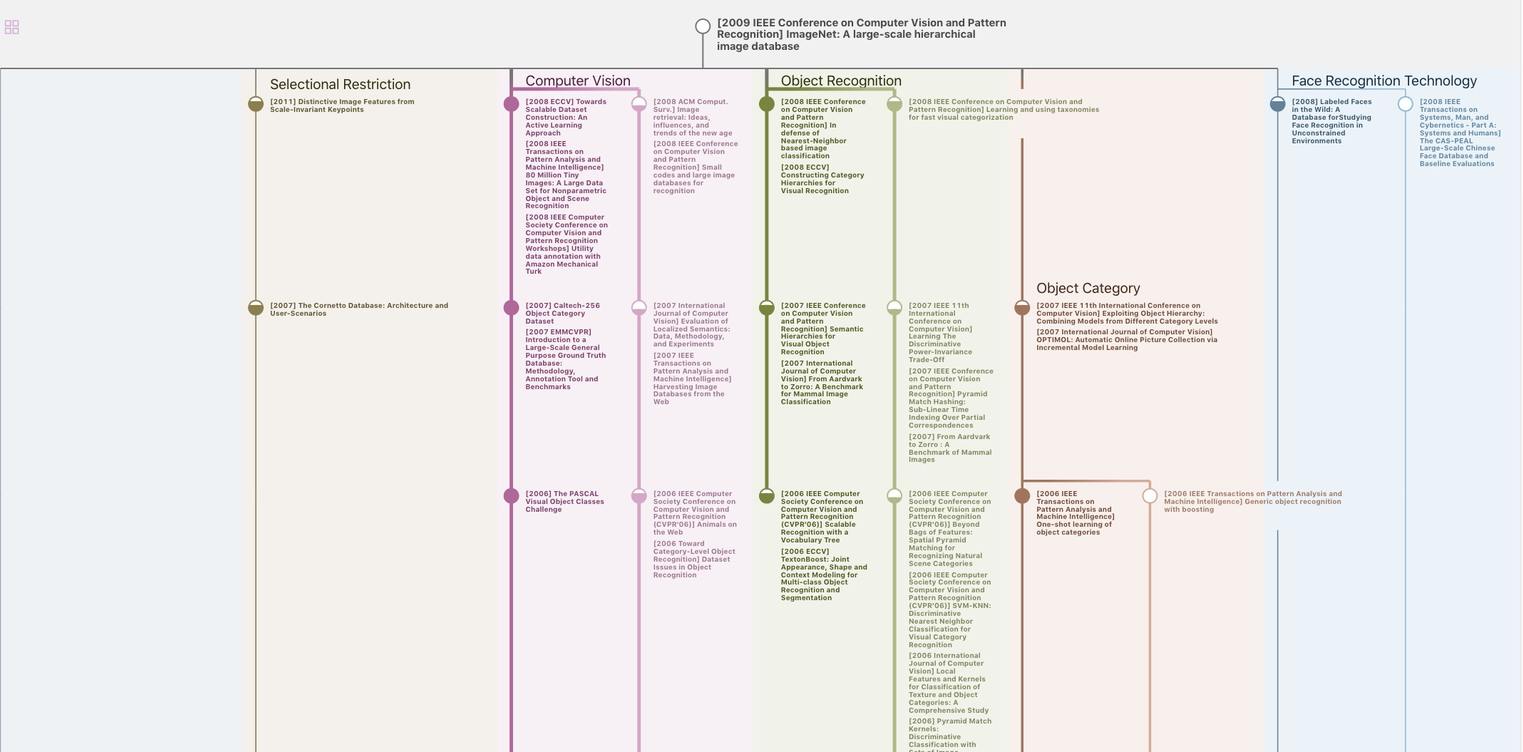
生成溯源树,研究论文发展脉络
Chat Paper
正在生成论文摘要