Rotating Machinery Fault Detection Using a New Version of Intrinsic Time-Scale Decomposition
IEEE SENSORS JOURNAL(2024)
摘要
The healthy state of bearings directly affects the safety and performance of rotating machinery. Developing effective fault diagnosis techniques is pivotal for the maintenance of rotating machinery. Given its adaptive decomposition capability, intrinsic time-scale decomposition (ITD) has gained wide acceptance and utilization in fault diagnosis. However, the decomposition ability of ITD is easily restricted when the raw signal contains a certain background noise. A new version of ITD is proposed to improve the noise immunity of ITD. Unlike the traditional ITD, the main contribution lies in combining parallel ITD decomposition with spectral subtraction to highlight fault information. A decomposition strategy is proposed to fully decompose the signal and to extract the fault component more robustly. Finally, the fault type can be detected using Hilbert envelope spectrum analysis. Simulated and experimental studies on faulty bearings are carried out to demonstrate the reliability and validity of the presented version of ITD. Compared with ITD and other commonly used empirical mode decomposition (EMD), experimental results verify the advantage of the new version of ITD in rotating machinery faults diagnosis.
更多查看译文
关键词
Feature extraction,Fault diagnosis,Machinery,Sensors,Fault detection,Deep learning,Compounds,Bearing,decomposition strategy,fault detection,intrinsic time-scale decomposition (ITD),spectral subtraction
AI 理解论文
溯源树
样例
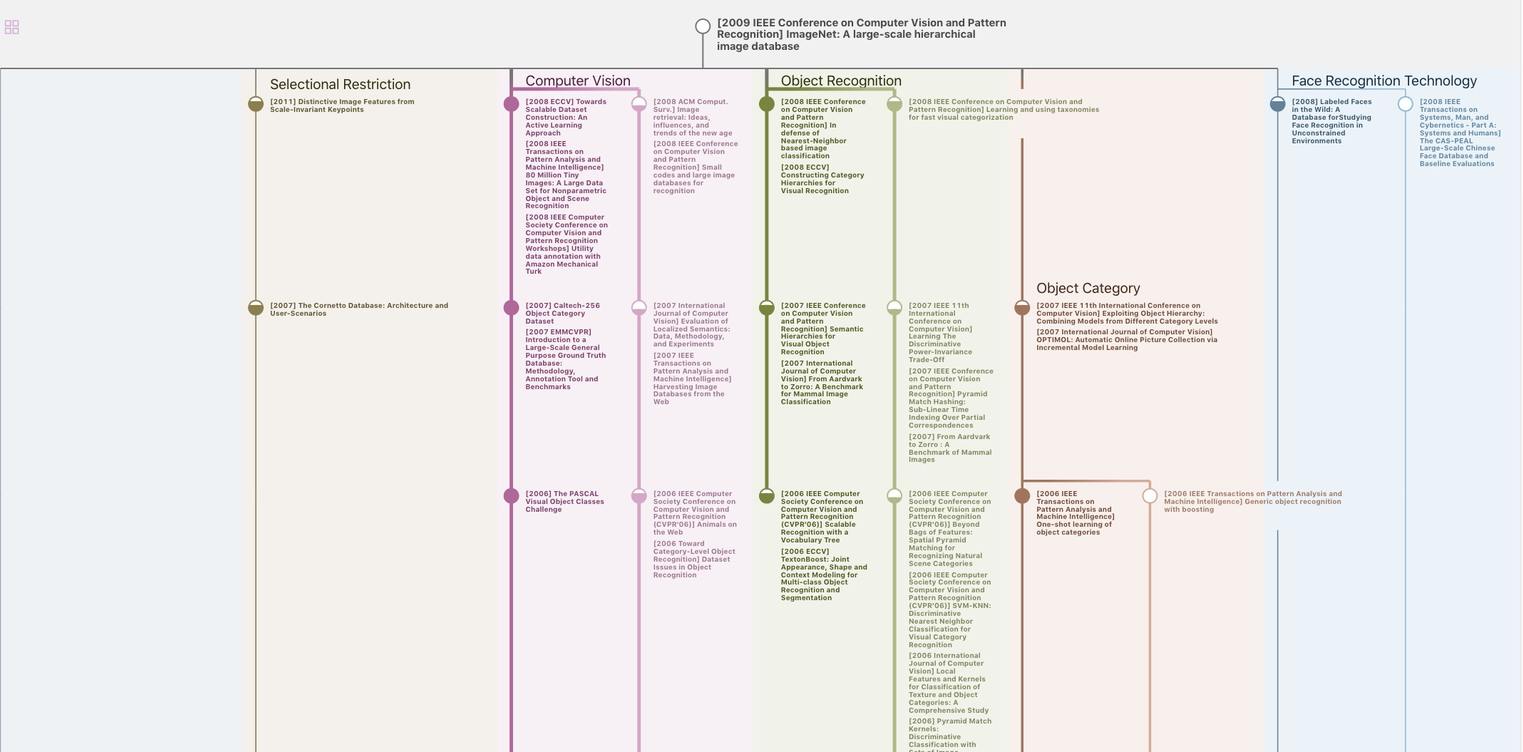
生成溯源树,研究论文发展脉络
Chat Paper
正在生成论文摘要