KSLD-TNet: Key Sample Location and Distillation Transformer Network for Multistep Ahead Prediction in Industrial Processes
IEEE Sensors Journal(2024)
摘要
The multistep ahead prediction of crucial quality indicators is the cornerstone for optimizing and controlling industrial processes. The accurate multistep ahead prediction over long prediction horizons holds great potential for improving production performance in industrial processes. However, extracting historical features presents a significant obstacle in achieving this objective. Recent advancements have demonstrated that transformer networks offer a promising technical solution to this challenge. Nevertheless, the lack of a sample simplification mechanism makes deep feature extraction difficult. It requires a lot of computational costs, which makes the traditional transformer network less applicable in industrial processes. To explore strategies to overcome these obstacles and enhance the suitability of transformer networks for effective multistep ahead prediction, this article proposes a novel key sample location and distillation transformer network (KSLD-TNet). Specifically, it first locates key samples with strong interactions using the attention score matrix. Then, nonkey samples are filtered out layer by layer in the KSLD-TNet encoder–decoder structure. In this way, the number of input samples for each layer can be lowered exponentially, reducing the difficulty and calculation amount of deep feature extraction significantly. It is worth noting that this article also designs an information storage structure to avoid information loss during the sample distillation process. Two industrial process datasets are utilized to construct extensive experiments to demonstrate the effectiveness of the proposed method.
更多查看译文
关键词
Deep learning,industrial process,key sample location (KSL) and distillation transformer,multistep ahead prediction
AI 理解论文
溯源树
样例
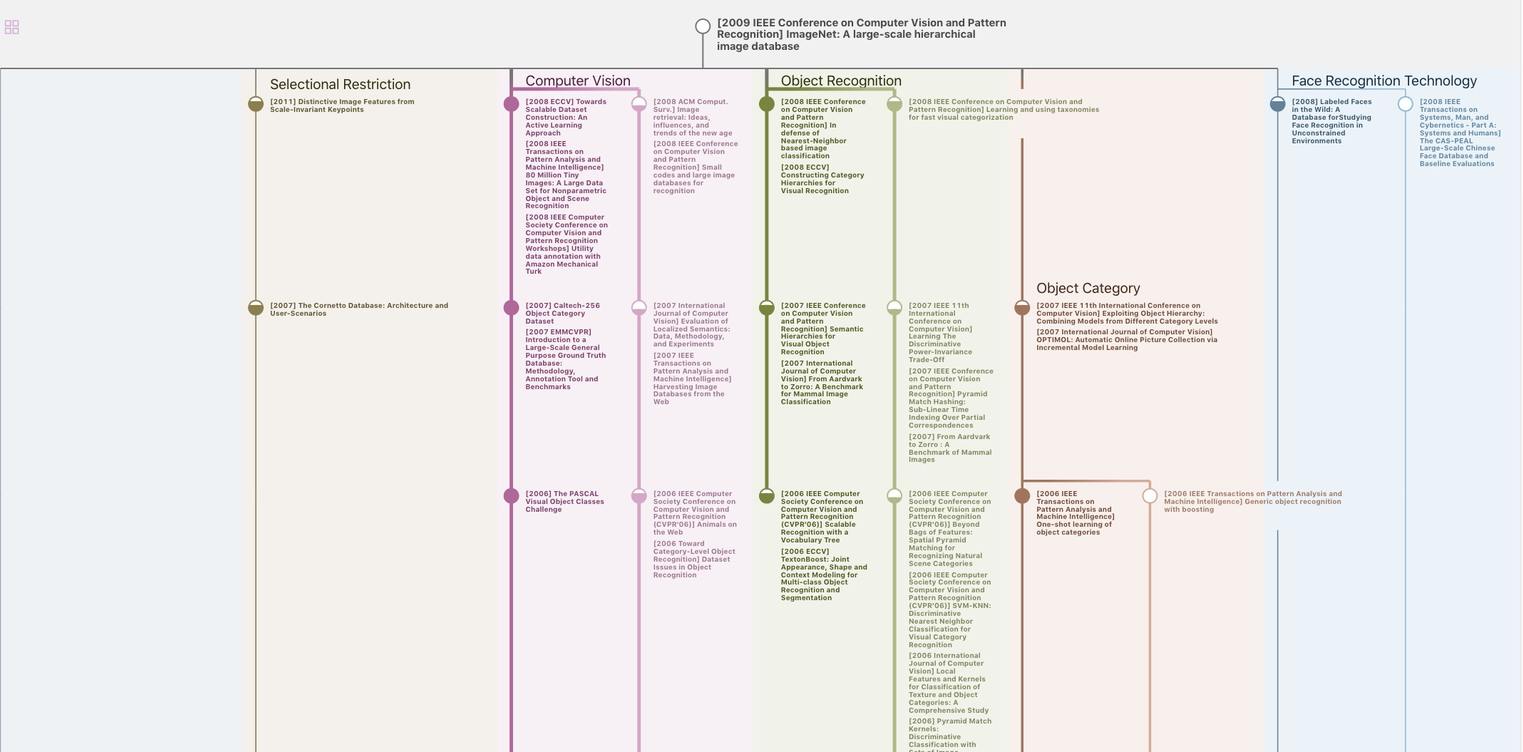
生成溯源树,研究论文发展脉络
Chat Paper
正在生成论文摘要