GADet: A Geometry-Aware X-Ray Prohibited Items Detector
IEEE SENSORS JOURNAL(2024)
摘要
X-ray prohibited items detection is an essential and highly effective method that has been widely used in inspection scenarios. To satisfy the practical demand for high accuracy in security auxiliary diagnosis systems, we propose a geometry-aware X-ray prohibited items detector (GADet) based on the classic and advanced general object detector, YOLOX. Specifically, we first analyze and discover that the relationship between diagonal length and prohibited items category in X-ray images is highly robust and distinctive. Therefore, we introduce the physical diagonal length constraint (PDLC), which can utilize this underlying relationship to align classification and localization tasks in object detection. In addition, although the general label assignment (LA) strategies for natural images can alleviate the foreground-background class imbalance, they cannot handle the unique foreground-background overlapping phenomenon in X-ray images. Thus, we propose the IoU-aware LA (IAA), which can assist the network to concentrate on learning how to extract informative features from overlapping foreground and background. Similar to YOLOX, our GADet offers three different versions, including small, large, and extreme for adapting different application requirements. Extensive experiments demonstrate that the proposed method outperforms the state-of-the-art object detection methods, indicating its potential applications in the prohibited items detection field. The source code will be released at https://github.com/Limingyuan001/GADet.
更多查看译文
关键词
Label assignment (LA),object detection,task alignment,X-ray inspection
AI 理解论文
溯源树
样例
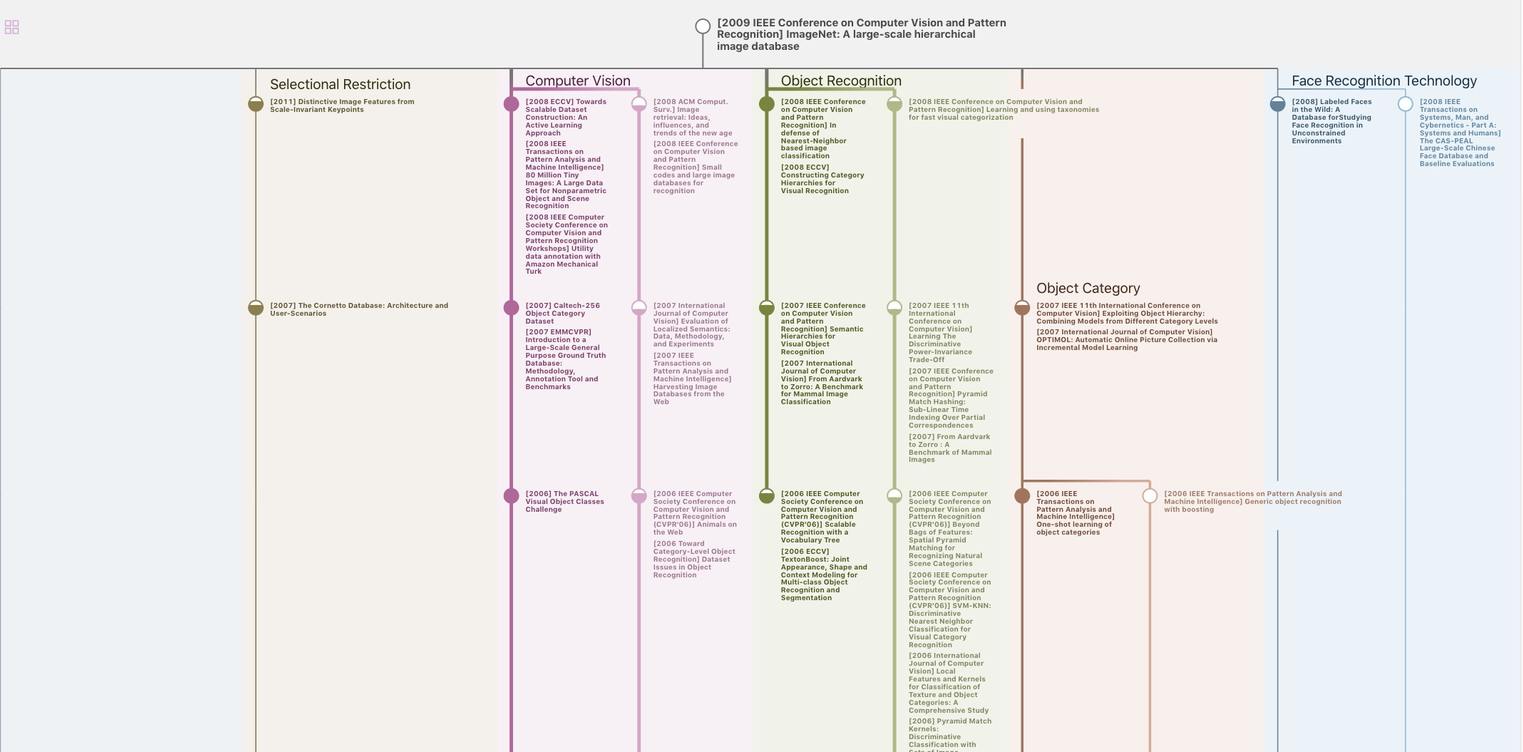
生成溯源树,研究论文发展脉络
Chat Paper
正在生成论文摘要