scFed: federated learning for cell type classification with scRNA-seq
BRIEFINGS IN BIOINFORMATICS(2024)
摘要
The advent of single-cell RNA sequencing (scRNA-seq) has revolutionized our understanding of cellular heterogeneity and complexity in biological tissues. However, the nature of large, sparse scRNA-seq datasets and privacy regulations present challenges for efficient cell identification. Federated learning provides a solution, allowing efficient and private data use. Here, we introduce scFed, a unified federated learning framework that allows for benchmarking of four classification algorithms without violating data privacy, including single-cell-specific and general-purpose classifiers. We evaluated scFed using eight publicly available scRNA-seq datasets with diverse sizes, species and technologies, assessing its performance via intra-dataset and inter-dataset experimental setups. We find that scFed performs well on a variety of datasets with competitive accuracy to centralized models. Though Transformer-based model excels in centralized training, its performance slightly lags behind single-cell-specific model within the scFed framework, coupled with a notable time complexity concern. Our study not only helps select suitable cell identification methods but also highlights federated learning's potential for privacy-preserving, collaborative biomedical research.
更多查看译文
关键词
federated learning,scRNA-seq,classification,cell type
AI 理解论文
溯源树
样例
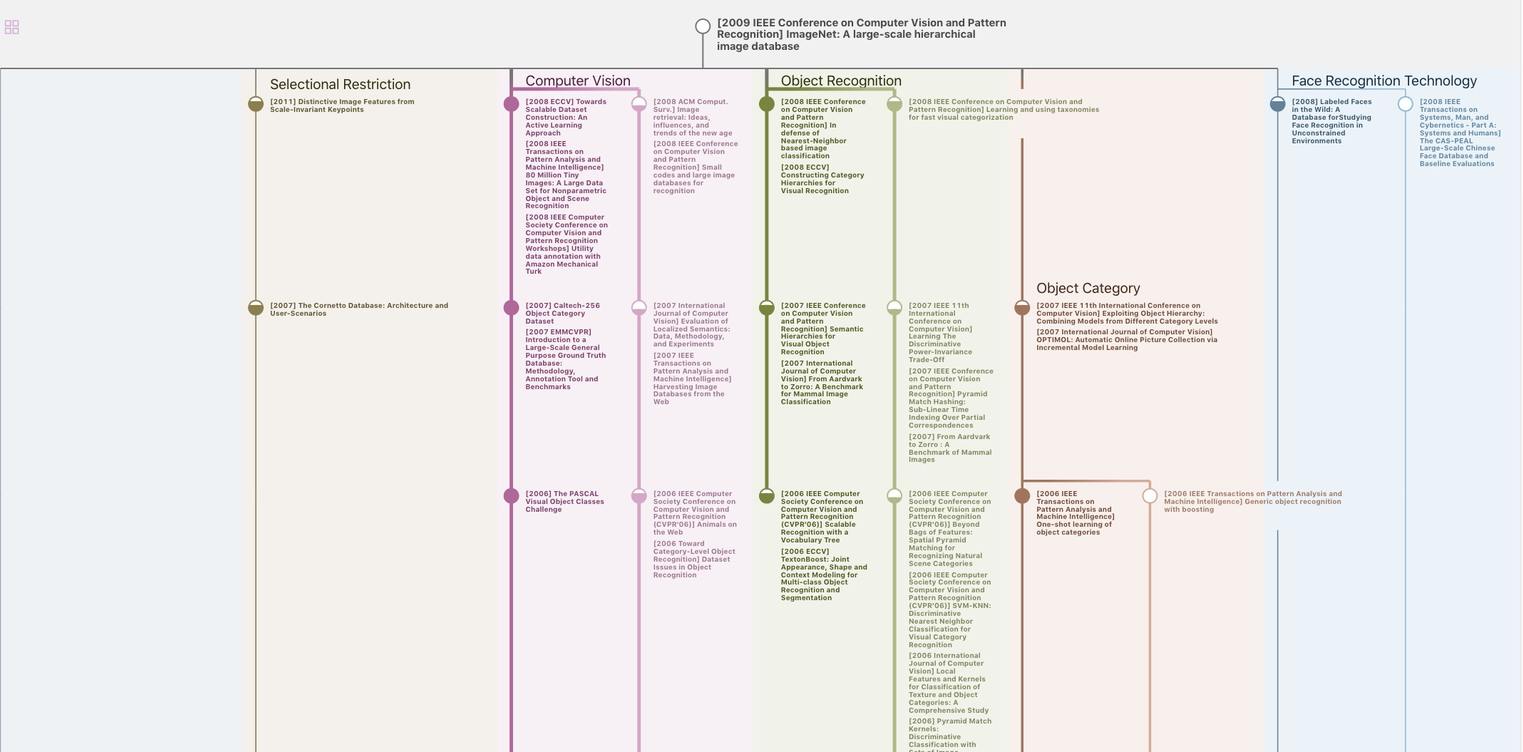
生成溯源树,研究论文发展脉络
Chat Paper
正在生成论文摘要