Bayesian diagnostic test evaluation and true prevalence estimation of malnutrition in gastric cancer patients
CLINICAL NUTRITION ESPEN(2024)
摘要
Background & aims: Malnutrition is prevalent among gastric cancer (GC) patients, necessitating early assessment of nutritional status to guide monitoring and interventions for improved outcomes. We aim to evaluate the accuracy and prognostic capability of three nutritional tools in GC patients, providing insights for clinical implementation. Methods: The present study is an analysis of data from 1308 adult GC patients recruited in a multicenter from July 2013 to July 2018. Nutritional status was assessed using Nutritional Risk Screening 2002 (NRS20 02), Patient-Generated Subjective Global Assessment (PG-SGA) and Global Leadership Initiative on Malnutrition (GLIM) criteria. Bayesian latent class model (LCM) estimated the malnutrition prevalence of GC patients, sensitivity and specificity of nutritional tools. Cox regression model analyzed the relationship between nutritional status and overall survival (OS) in GC patients. Results: Among 1308 GC patients, NRS-20 02, PG-SGA, and GLIM identified 50.46%, 76.76%, and 68.81% as positive, respectively. Bayesian LCM analysis revealed that PG-SGA had the highest sensitivity (0.96) for malnutrition assessment, followed by GLIM criteria (0.78) and NRS-20 02 (0.65). Malnutrition or being at risk of malnutrition were identified as independent prognostic factors for OS. Use any of these tools improved survival prediction in TNM staging system. Conclusion: PG-SGA is the most reliable tool for diagnosing malnutrition in GC patients, whereas NRS20 02 is suitable for nutritional screening in busy clinical practice. Given the lower sensitivity of NRS20 02, direct utilization of GLIM for nutritional assessment may be necessary. Each nutritional tool should be associated with a specific course of action, although further research is needed. (c) 2023 Published by Elsevier Ltd on behalf of European Society for Clinical Nutrition and Metabolism.
更多查看译文
关键词
Gastric cancer,Bayesian latent class model,Diagnostic accuracy,GLIM,PG-SGA,NRS-20 02
AI 理解论文
溯源树
样例
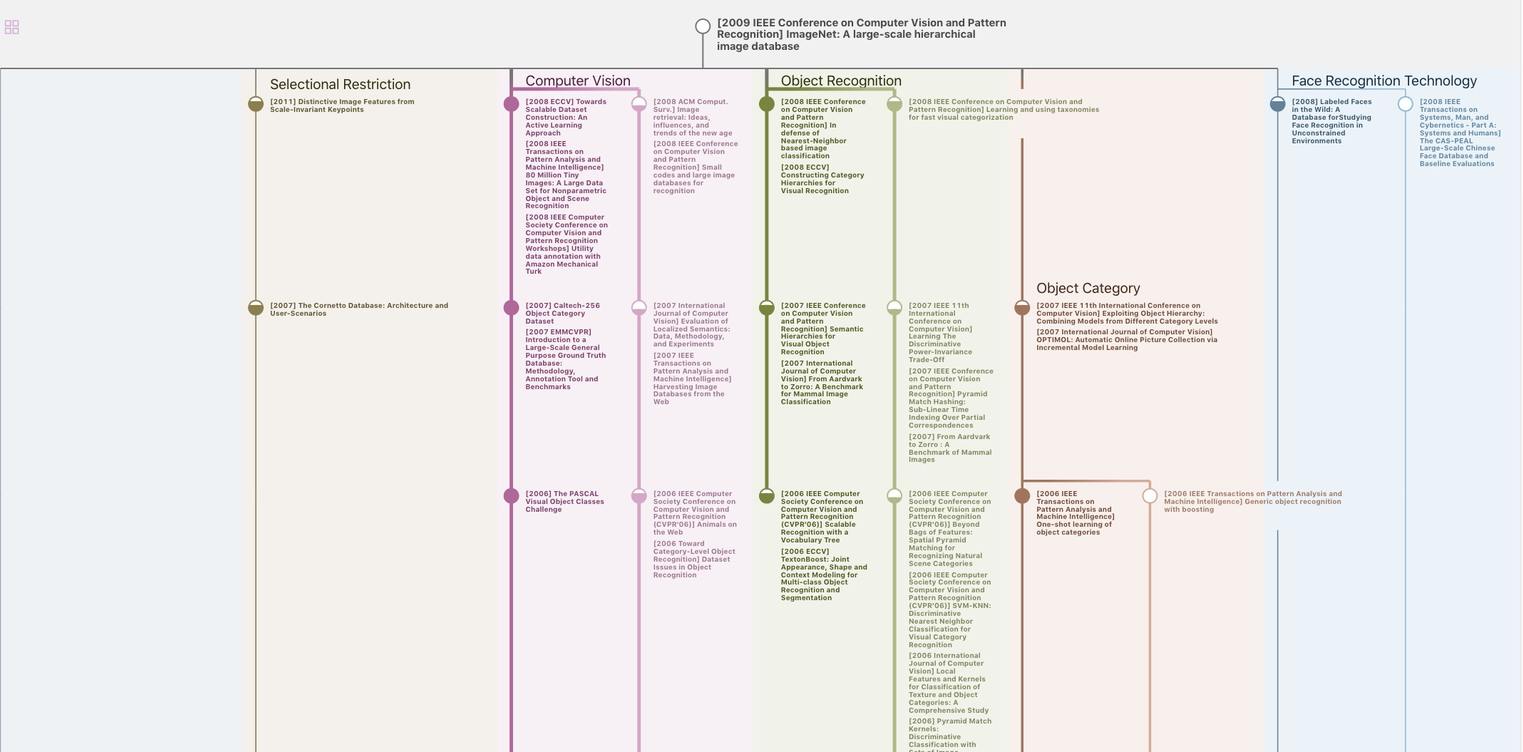
生成溯源树,研究论文发展脉络
Chat Paper
正在生成论文摘要