Relevance vector machine with hybrid kernel-based soft sensor via data augmentation for incomplete output data in sintering process
CONTROL ENGINEERING PRACTICE(2024)
摘要
A ratio of CO and CO2 (CO/CO2) is a key indicator of sintering carbon consumption, which is difficult to be determined in real-time. Therefore, the establishment of its soft sensing model is of great practical significance. This paper proposes a novel CO/CO2 soft sensing model with incomplete output data based on relevance vector machine with hybrid kernel via data augmentation. First, a least absolute shrinkage and selection operator is employed for determining key input variables of the model, and an automatic fuzzy clustering framework is used to automatically identify multiple operating modes. Then, a relevance vector machine with hybrid kernel method is presented to model each operating mode separately. Meanwhile, considering the problem of incomplete input and output data, data augmentation is applied in modeling to enhance the model performance. Finally, the soft sensing model of CO/CO2 is formed. Experimental results and analyses using actual production data coming from the sintering production process demonstrate that the prediction performance and accuracy of the proposed model outperform some existing algorithms.
更多查看译文
关键词
Sintering process,Actual production data,CO/CO2 soft sensing model,Data augmentation,Relevance vector machine
AI 理解论文
溯源树
样例
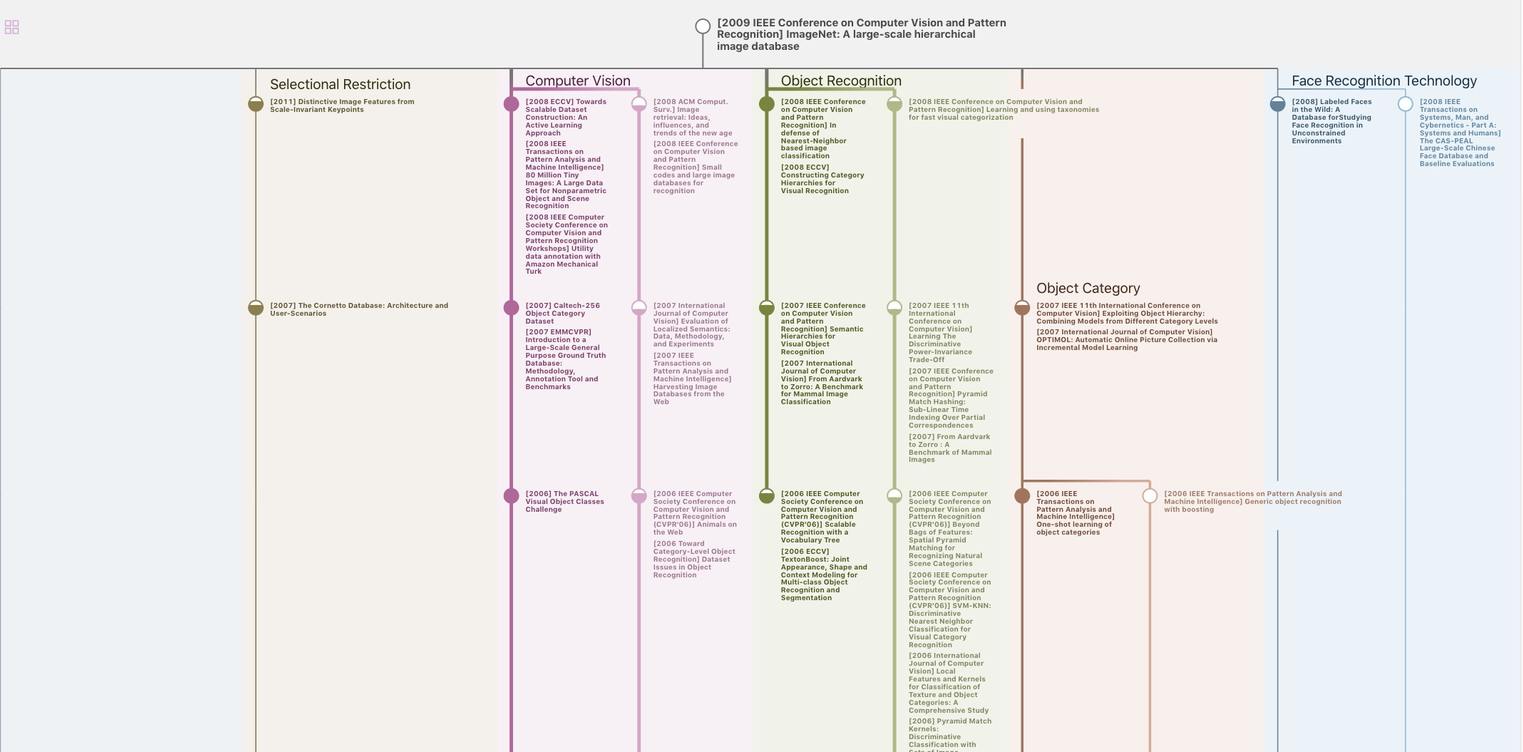
生成溯源树,研究论文发展脉络
Chat Paper
正在生成论文摘要