Machine learning coupled with group contribution for predicting the electrical conductivity of ionic liquids with experimental accuracy
FLUID PHASE EQUILIBRIA(2024)
摘要
Accurate prediction of the electrical conductivity of ionic liquids (ILs) is crucial for their efficient design and application in various fields. In this study, new group contribution (GC) method is proposed for the prediction of the electrical conductivity of pure ILs as a function of temperature (T) and pressure (p). This group contribution scheme enables the comprehensive description of diverse ionic liquids and the differentiation among isomers. Four machine learning algorithms, namely Extreme Gradient Boosting (XGBoost), Random Forest (RF), Support Vector Machines (SVM) and Deep Neural Networks (DNN) are developed and compared with a conventional approach and other methods recently proposed in the literature. The proposed models are developed using the largest collection of data reported to date, which includes a total of 7598 data points for 520 ionic liquids of various chemical families. This database underwent a rigorous and thorough review, evaluation, and reduction process to ensure its reliability. The GC scheme was adopted from our previous work. The results indicate that the DNN model outperforms other approaches, closely followed by the SVM model. The predictive accuracy of both models falls within the experimental uncertainty range, validating their reliability. Additionally, the influence of cationic and anionic families on model performance is explored, providing valuable insights into IL behavior.
更多查看译文
关键词
Ionic liquid,Electrical conductivity,Group contribution,Prediction,Machine learning
AI 理解论文
溯源树
样例
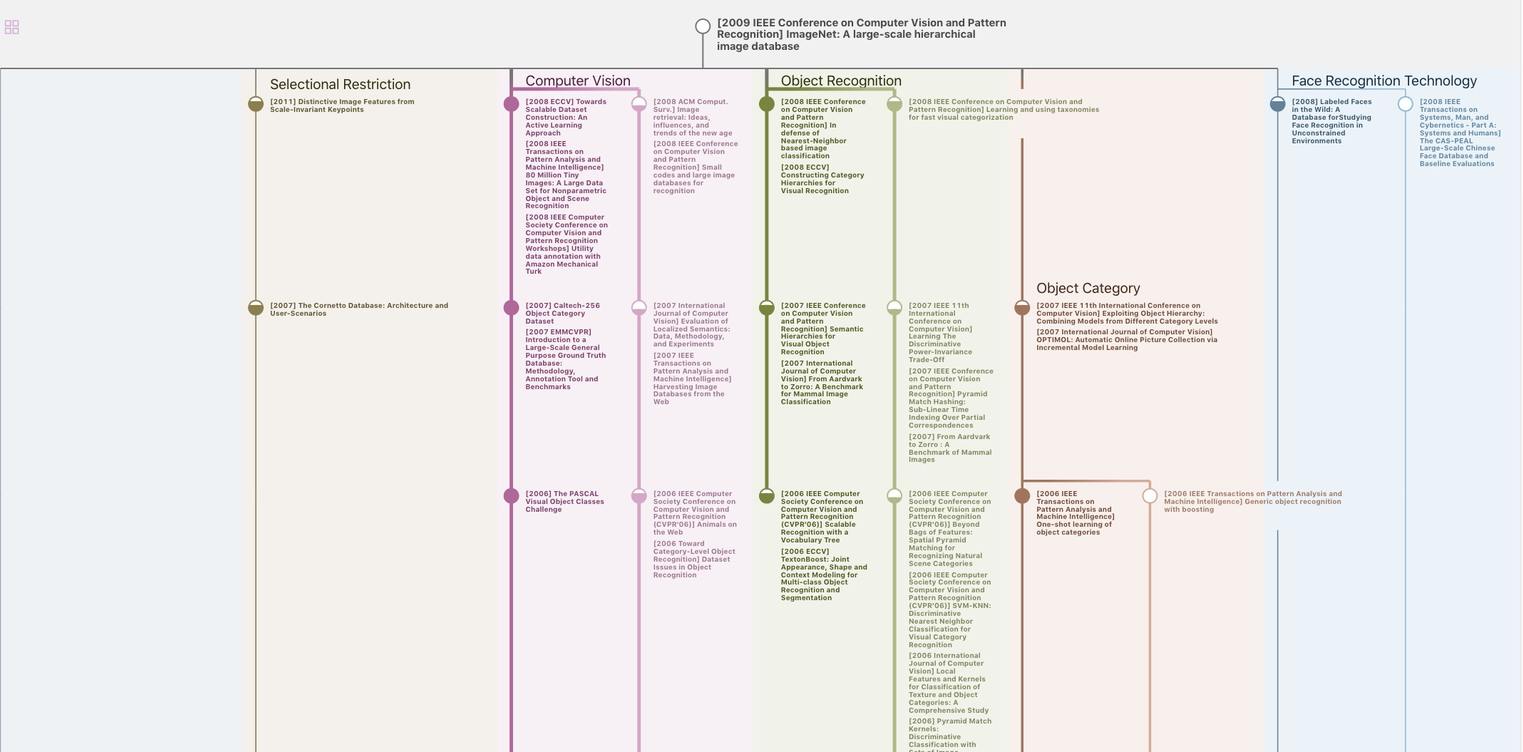
生成溯源树,研究论文发展脉络
Chat Paper
正在生成论文摘要