Time-aware and task-transferable adversarial attack for perception of autonomous vehicles
PATTERN RECOGNITION LETTERS(2024)
摘要
With rapid development of self-driving vehicles, recent work in adversarial machine learning started to study adversarial examples (AEs) for perception of autonomous driving (AD). However, generating practical AEs for the perception module remains a significant challenge. Traditional adversarial attacks tend to focus on a single computer vision task, making it difficult to compromise multiple perception tasks such as object detection and segmentation simultaneously. Additionally, the limited computational resources available on -board and the necessity for online operation pose further obstacles to deploying adversarial attacks on real autonomous driving platforms. To address the aforementioned issues, we propose the Time-aware Perception Attack (TPA), which is a real -time cross -task adversarial attack for perception of autonomous driving. In particular, we propose a novel backbone based adversarial attack method to modify input images to approach Lipschitz Constant Point (LCP), which results in erroneous inferences for all the sub-models in perception module. The novel part of this work is proposing an efficient yet effective LCP approaching algorithm. Comparing to conventional LCP based attacks, which consume significant amount of computation resources and can be only applied on small DNNs, TPA generates AEs on an intermediate layer of surrogate backbone, significantly enhancing the cross -task transferability and accelerates the attack process. Evaluation results on Berkeley Driving Dataset 100k (BDD100k) show that, comparing to the state -of -the -art baselines, the proposed TPA achieves higher attack effectiveness and faster processing speed and outperforms the baselines by a large margin.
更多查看译文
关键词
Adversarial attack,Black-box,Perception,Real-time
AI 理解论文
溯源树
样例
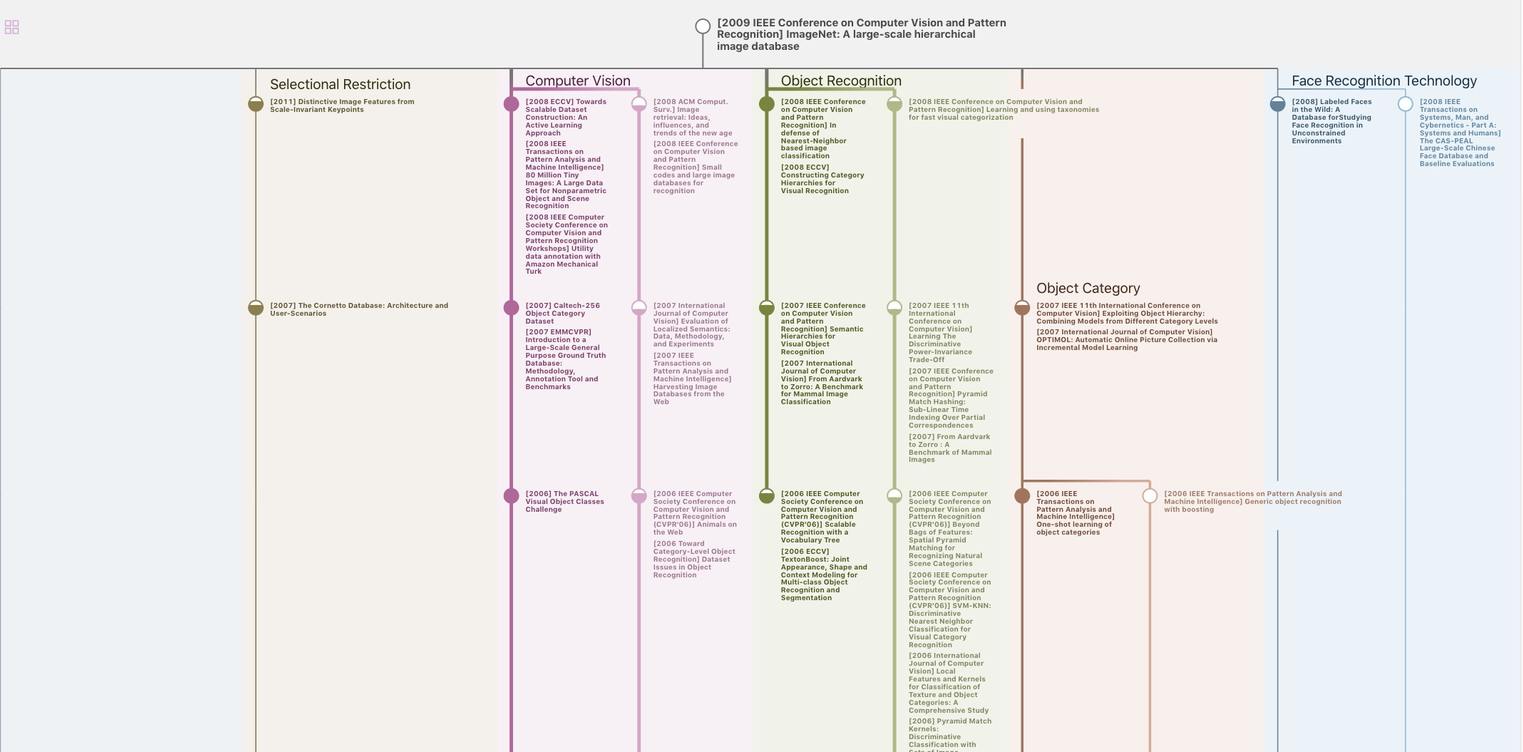
生成溯源树,研究论文发展脉络
Chat Paper
正在生成论文摘要