An Experimental Overview of Neural-Symbolic Systems
INDUCTIVE LOGIC PROGRAMMING, ILP 2023(2023)
摘要
Neural-symbolic AI is the field that seeks to integrate deep learning with symbolic, logic-based methods, as they have complementary strengths. Lately, more and more researchers have encountered the limitations of deep learning, which has led to a rise in the popularity of neural-symbolic AI, with a wide variety of systems being developed. However, many of these systems are either evaluated on different benchmarks, or introduce new benchmarks that other systems have not been tested on. As a result, it is unclear which systems are suited to which tasks, and whether the difference between systems is actually significant. In this paper, we give an overview and classification of the tasks used in state-of-the-art neural-symbolic system. We show that most tasks fall in one of five categories, and that very few systems are compared on the same benchmarks. We also provide a methodological experimental comparison of a variety of systems on two popular tasks: learning with distant supervision and structured prediction. Our results show that a systems based on (probabilistic) logic programming achieve superior performance on these tasks, and that the performance amongst these methods does not differ significantly. Finally, we also discuss how the properties of the (probabilistic) logic programming-based systems are desirable for most neural-symbolic tasks.
更多查看译文
关键词
Neural-symbolic AI,Probabilistic Logic Programming,Experimental Survey
AI 理解论文
溯源树
样例
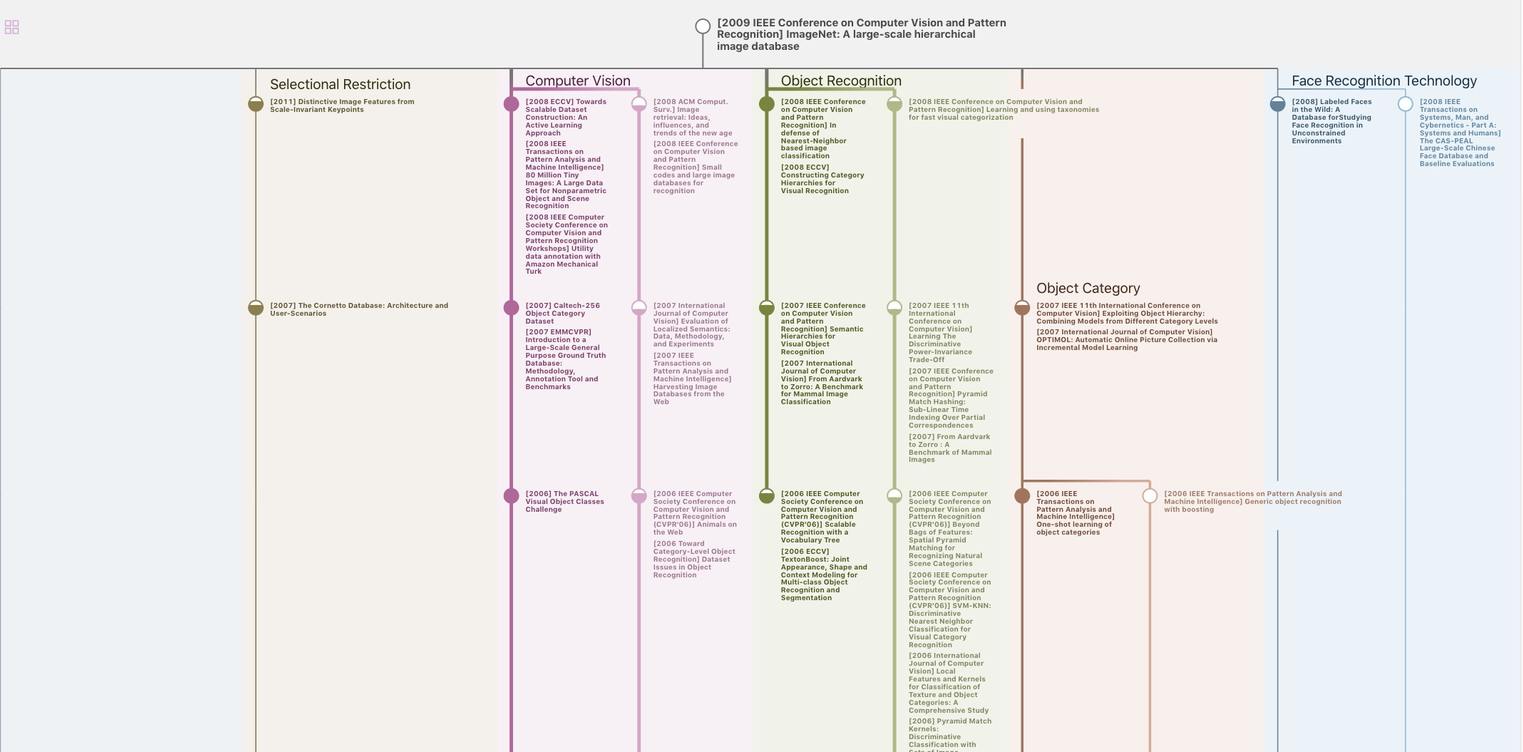
生成溯源树,研究论文发展脉络
Chat Paper
正在生成论文摘要