Select First, Transfer Later: Choosing Proper Datasets for Statistical Relational Transfer Learning
INDUCTIVE LOGIC PROGRAMMING, ILP 2023(2023)
摘要
Statistical Relational Learning (SRL) relies on statistical and probabilistic modeling to represent, learn, and reason about domains with complex relational and rich probability structures. Although SRL techniques have succeeded in many real-world applications, they follow the same assumption as most ML techniques by assuming training and testing data have the same distribution and are sampled from the same feature space. Changes between these distributions might require training a new model using new data. Transfer Learning adapts knowledge already learned to other tasks and domains to help create new models, particularly in a low-data regime setting. Many recent works have succeeded in applying Transfer Learning to relational domains. However, most focus on what and how to transfer. When to transfer is still an open research problem as a pre-trained model is not guaranteed to help or improve performance for learning a new model. Besides, testing every possible pair of source and target domains to perform transference is costly. In this work, we focus on when by proposing a method that relies on probabilistic representations of relational databases and distributions learned by models to indicate the most suitable source domain for transferring. To evaluate our approach, we analyze the performances of two transfer learning-based algorithms given the most similar target domain to a source domain according to our proposal. In the experimental results, our method has succeeded as both algorithms reach their best performance when transferring between the most similar pair of source and target domains.
更多查看译文
关键词
Statistical relational learning,Transfer learning,Relational data similarity
AI 理解论文
溯源树
样例
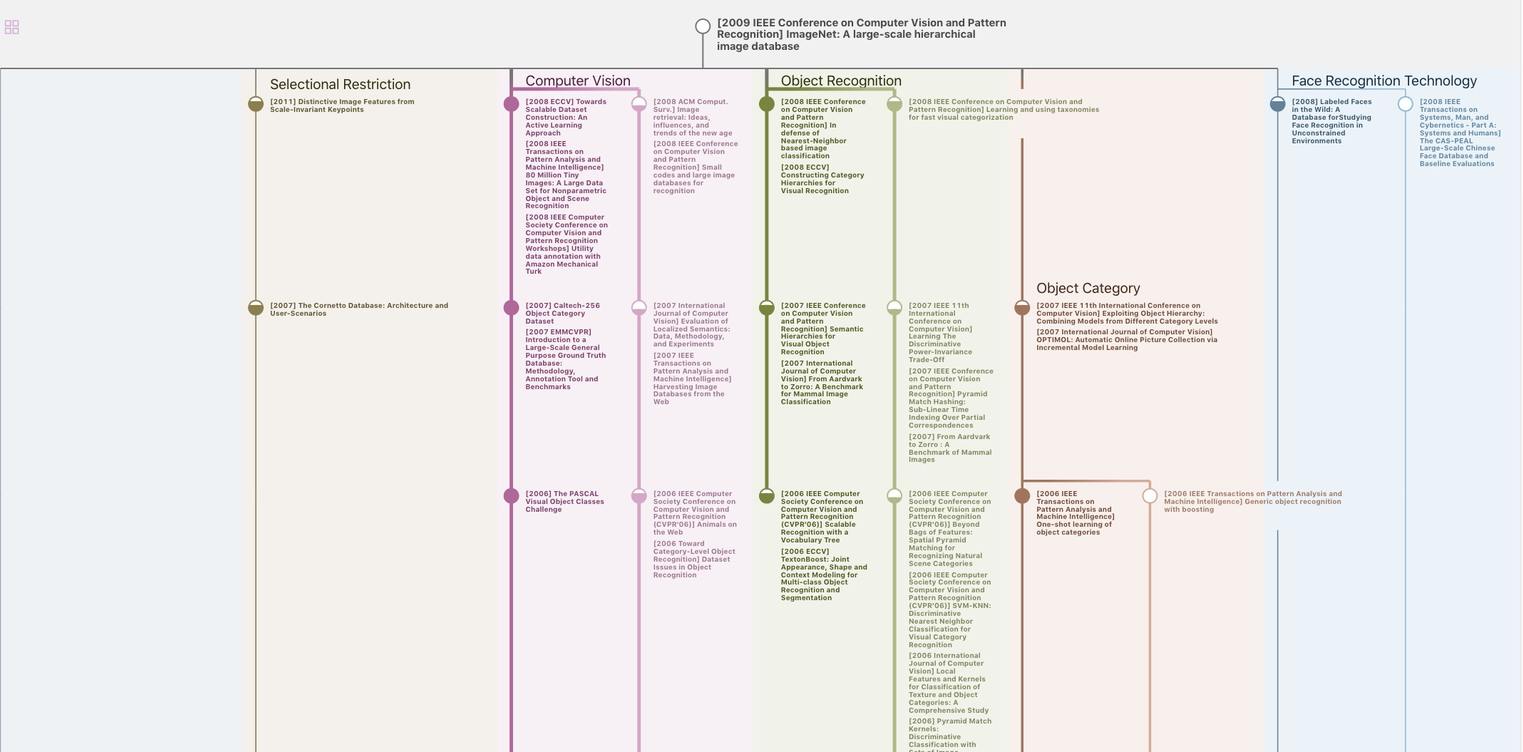
生成溯源树,研究论文发展脉络
Chat Paper
正在生成论文摘要