Model-Guided Synthesis for LTL over Finite Traces
VERIFICATION, MODEL CHECKING, AND ABSTRACT INTERPRETATION, VMCAI 2024, PT I(2024)
摘要
Satisfiability and synthesis are two fundamental problems for Linear Temporal Logic, both of which can be solved on the automaton constructed from the input formula. In general, satisfiability is easier than synthesis in both theory and practice, as satisfiability needs only to find a satisfying trace, while synthesis has to find a winning strategy. This paper presents a novel technique called MoGuS, which improves the performance of synthesis for LTLf, a variant of LTL interpreted over finite traces, by repeatedly invoking an LTLf satisfiability checker to guide its search for a winning strategy. Satiisfiabiity checkers have not been used before in the context of LTLf synthesis. MoGuS computes a satisfying trace of the input formula, and then uses the formulaprogression technique to compute the states on the fly in the automaton run. It then checks whether there exists a winning strategy from each of the states. If not, the current state is marked as a ' failure ' state (as it can never produce a winning strategy), the checking rolls back to its predecessor state, and the process repeats. MoGuS returns ' Realizable ' if the initial state turns out to be winning, and ' Unrealizable ' otherwise. We conducted an extensive experimental evaluation of MoGuS by comparing it to different state-of-the-art LTLf synthesis algorithms on a large set of benchmarks. The results show that MoGuS has the most stable and the best overall performance on the tested benchmarks.
更多查看译文
AI 理解论文
溯源树
样例
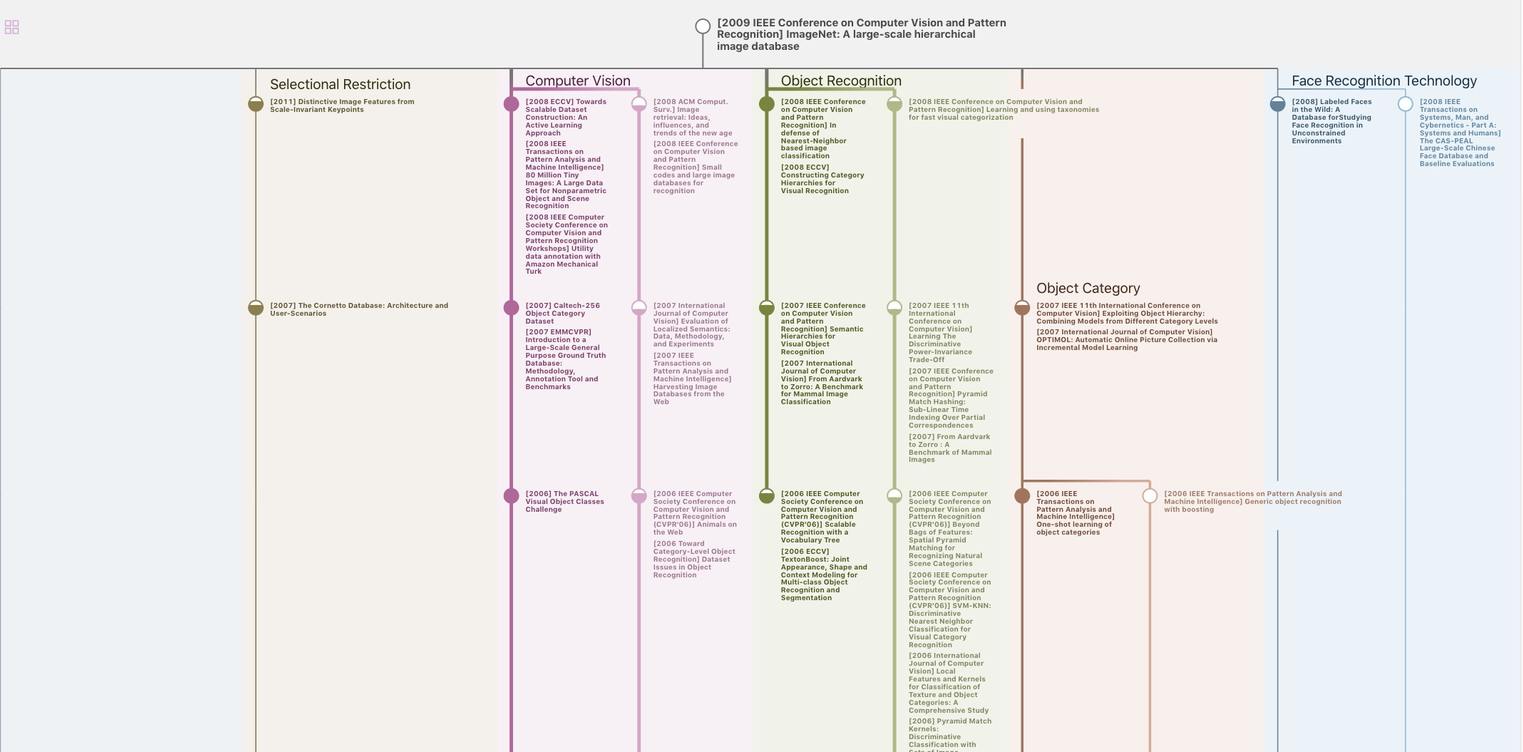
生成溯源树,研究论文发展脉络
Chat Paper
正在生成论文摘要