CenterMatch: A Center Matching Method for Semi-supervised Facial Expression Recognition
PATTERN RECOGNITION AND COMPUTER VISION, PRCV 2023, PT VI(2024)
摘要
The label uncertainty in large-scale qualitative facial expression datasets, caused by low-quality images and subjective annotations, combined with the high similarity between facial expression categories, makes facial expression recognition (FER) more challenging compared to traditional classification tasks. To address this problem, this paper proposes a new method called CenterMatch for semi-supervised facial expression recognition. Our approach sets class centers in a high-dimensional space and independent adaptive confidence thresholds for each category. As the positions of class centers are continuously updated by high-quality sample features, the confidence thresholds for each category adapt accordingly. Subsequently, high-confidence unlabeled samples are selected and assigned high-quality pseudo-labels, effectively suppressing label uncertainty. Additionally, we introduce a distance loss to constrain the updating direction of class centers, encouraging them to move away from each other and overcome the challenge of high similarity between expression categories. Experimental results demonstrate that our model outperforms other semi-supervised learning methods by adaptively adjusting confidence thresholds based on the learning status and difficulty of different categories, achieving a favorable balance between the quality and quantity of pseudo-labels. Furthermore, our approach exhibits excellent performance on multiple widely-used challenging in-the-world datasets, confirming its effectiveness and generalizability.
更多查看译文
关键词
Facial Expression Recognition,Semi-supervised Learning,Affective Computing
AI 理解论文
溯源树
样例
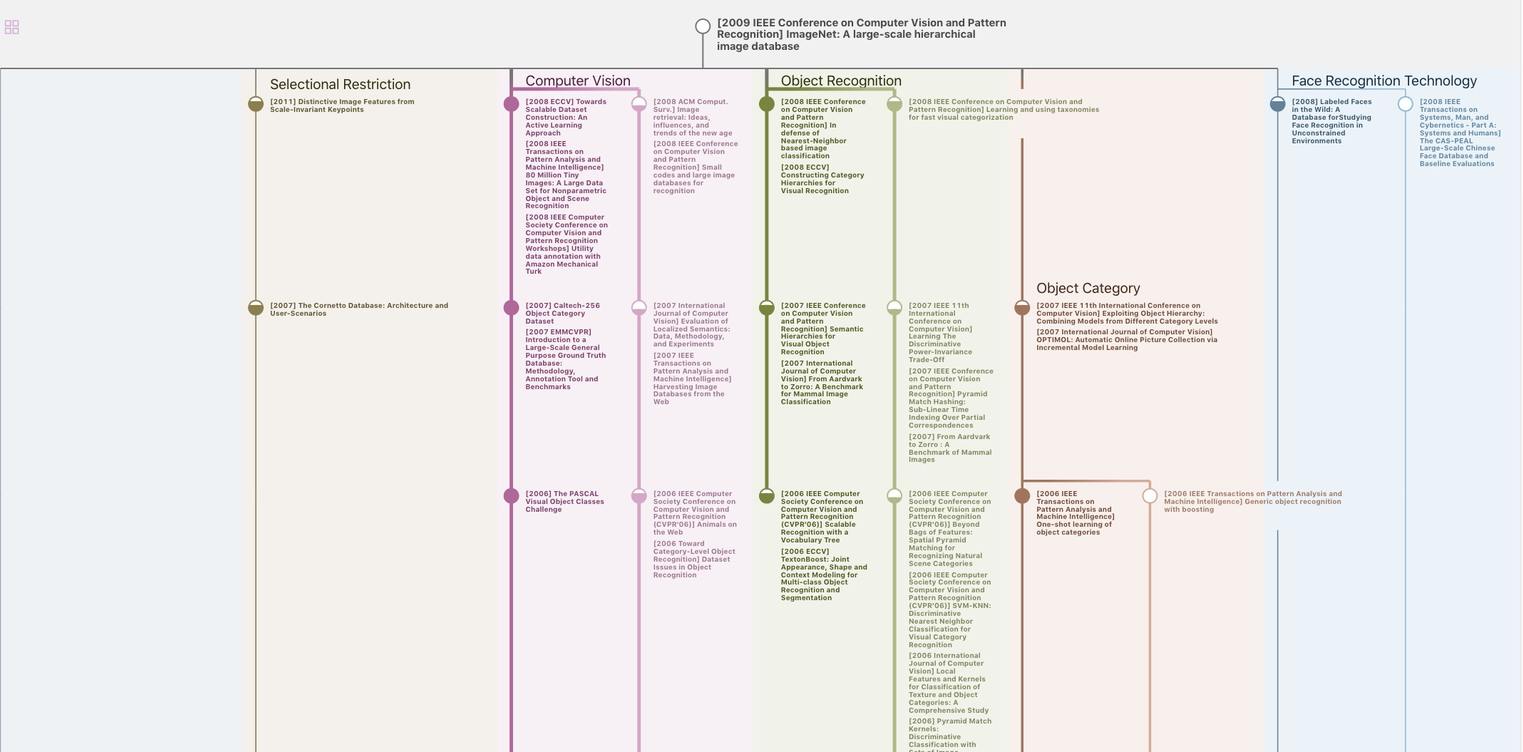
生成溯源树,研究论文发展脉络
Chat Paper
正在生成论文摘要