JLInst: Boundary-Mask Joint Learning for Instance Segmentation.
PATTERN RECOGNITION AND COMPUTER VISION, PRCV 2023, PT XII(2024)
Key words
Instance Segmentation,Boundary Information Enhancement
AI Read Science
Must-Reading Tree
Example
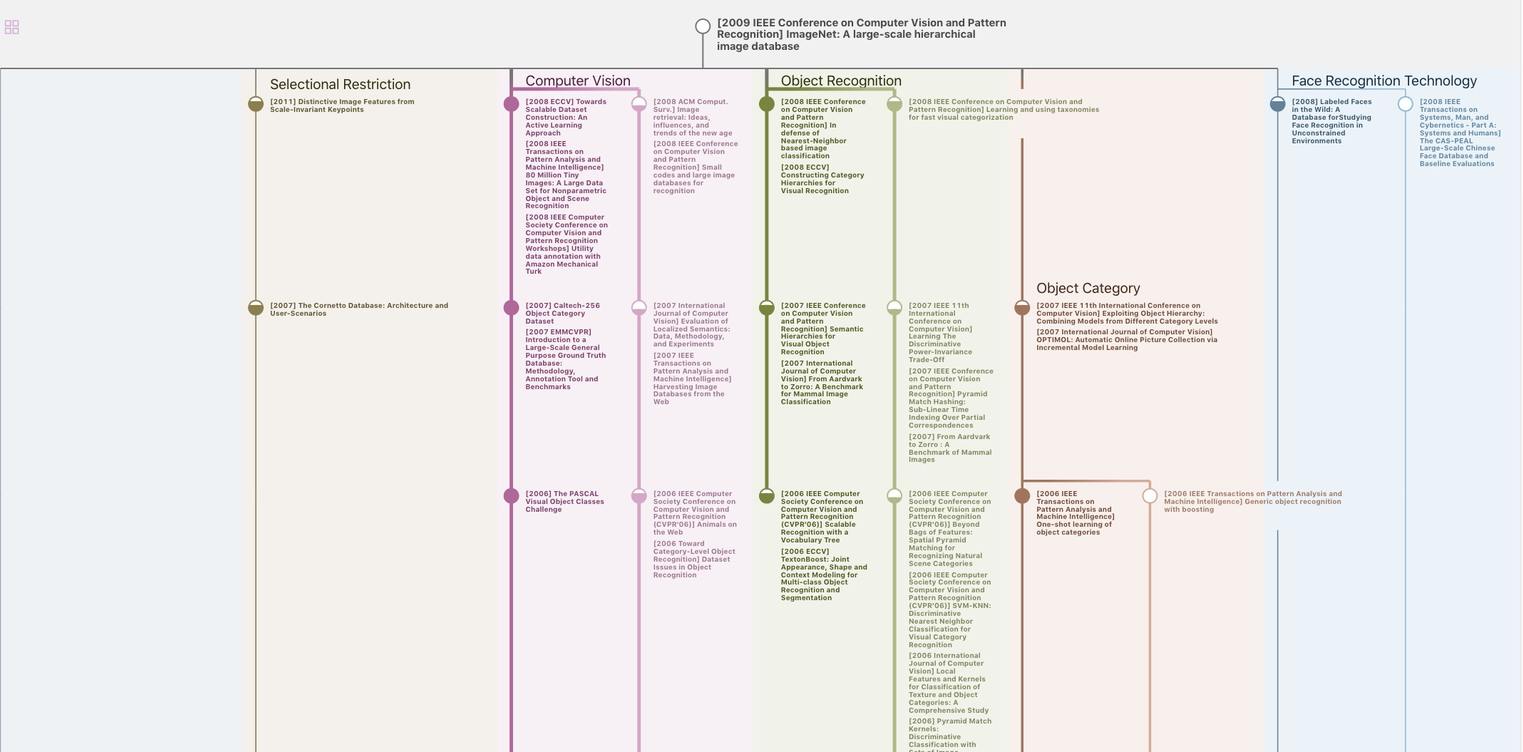
Generate MRT to find the research sequence of this paper
Chat Paper
Summary is being generated by the instructions you defined