Event Sparse Net: Sparse Dynamic Graph Multi-representation Learning with Temporal Attention for Event-Based Data
PATTERN RECOGNITION AND COMPUTER VISION, PRCV 2023, PT IX(2024)
摘要
Graph structure data has seen widespread utilization in modeling and learning representations, with dynamic graph neural networks being a popular choice. However, existing approaches to dynamic representation learning suffer from either discrete learning, leading to the loss of temporal information, or continuous learning, which entails significant computational burdens. Regarding these issues, we propose an innovative dynamic graph neural network called Event Sparse Net (ESN). By encoding time information adaptively as snapshots and there is an identical amount of temporal structure in each snapshot, our approach achieves continuous and precise time encoding while avoiding potential information loss in snapshot-based methods. Additionally, we introduce a lightweight module, namely Global Temporal Attention, for computing node representations based on temporal dynamics and structural neighborhoods. By simplifying the fully-connected attention fusion, our approach significantly reduces computational costs compared to the currently best-performing methods. We assess our methodology on four continuous/discrete graph datasets for link prediction to assess its effectiveness. In comparison experiments with top-notch baseline models, ESN achieves competitive performance with faster inference speed.
更多查看译文
关键词
dynamic graph representations,self-attention mechanism,light sparse temporal model,link prediction
AI 理解论文
溯源树
样例
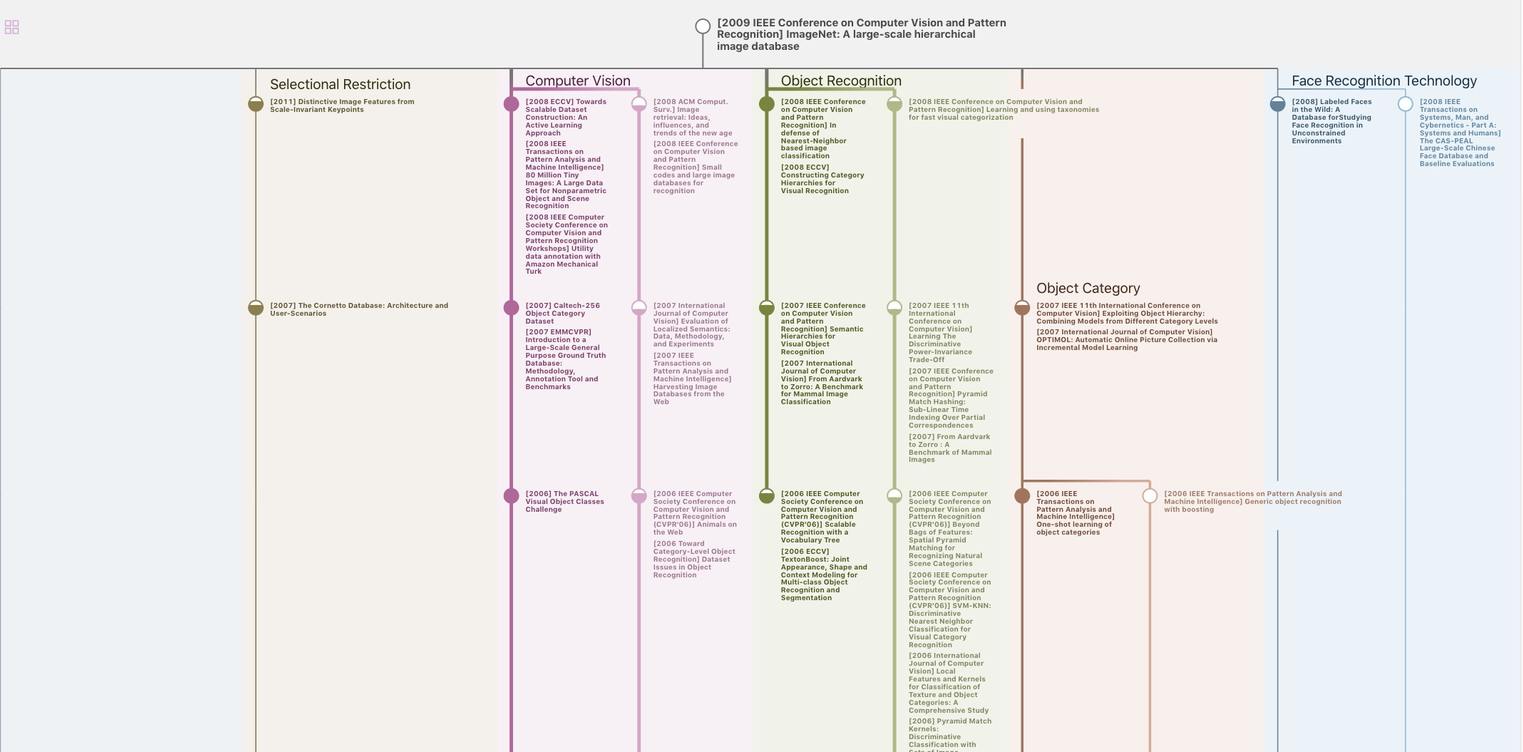
生成溯源树,研究论文发展脉络
Chat Paper
正在生成论文摘要