FGPTQ-ViT: Fine-Grained Post-training Quantization for Vision Transformers
PATTERN RECOGNITION AND COMPUTER VISION, PRCV 2023, PT IX(2024)
Abstract
The complex architecture and high training cost of Vision Transformers (ViTs) have prompted the exploration of post-training quantization (PTQ). However, introducing previous PTQ into ViTs performed worse because the activation values after processing by the softmax and GELU functions were extremely unbalanced distributed and not the common Gaussian distribution. To solve this problem, we propose a fine-grained ViT quantization method to fit this special distribution and reduce the quantization error of the activation values. We also design an adaptive piecewise point search algorithm that can automatically find the optimal piecewise point. Both the piecewise point and its search process are in the form of a power of two, making it possible to be implemented on general-purpose hardware with a simple shift operation. Experiments show that the quantization algorithm requires only 32 calibration images and achieves nearly lossless prediction accuracy in the classification task of ImageNet dataset. (The accuracy degradation for 8-bit quantization does not exceed 0.45%, and the average degradation is 0.17%).
MoreTranslated text
Key words
Vision transformer quantization,Piecewise quantization,Post-training quantization,Adaptive search algorithm
AI Read Science
Must-Reading Tree
Example
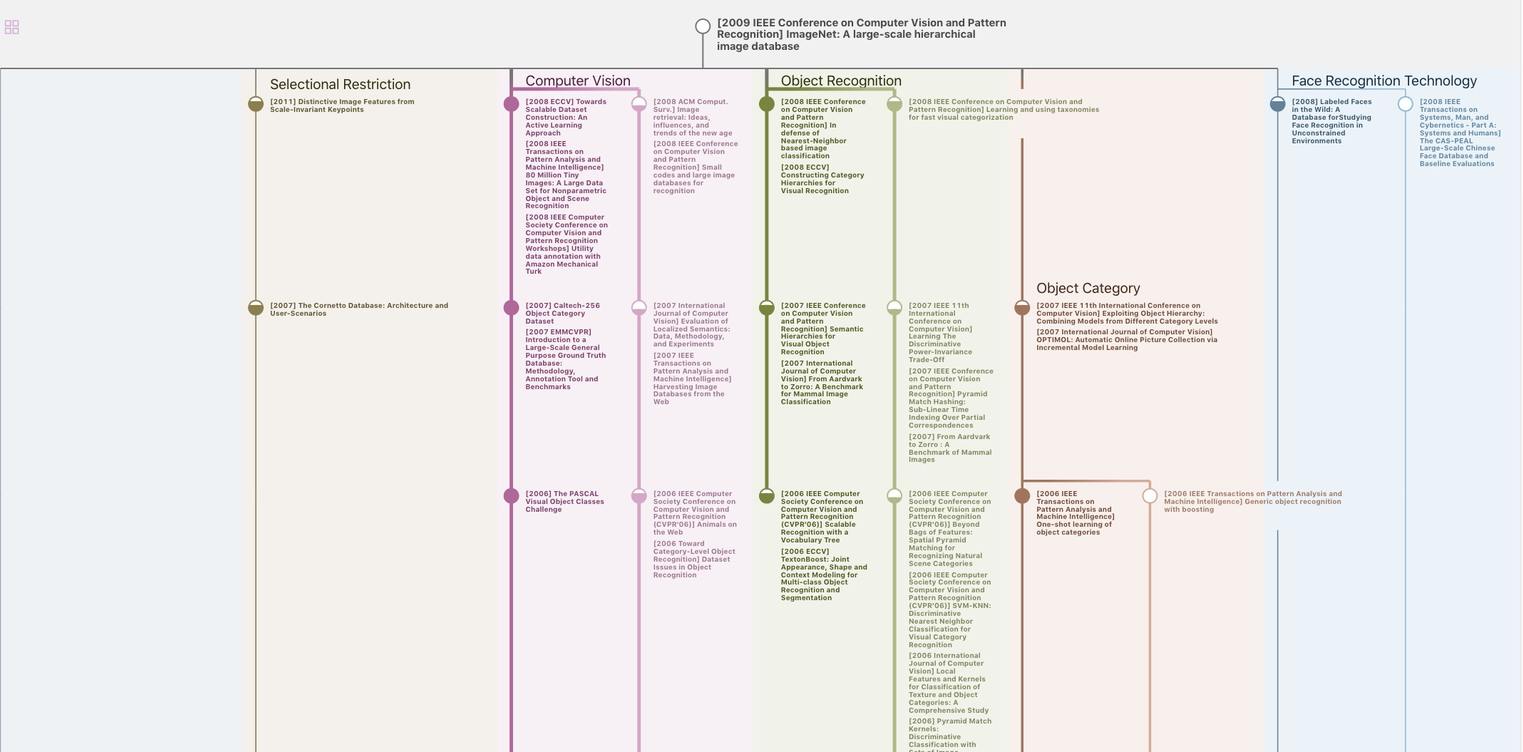
Generate MRT to find the research sequence of this paper
Chat Paper
Summary is being generated by the instructions you defined