Multi-atlas Representations Based on Graph Convolutional Networks for Autism Spectrum Disorder Diagnosis
PATTERN RECOGNITION AND COMPUTER VISION, PRCV 2023, PT XIII(2024)
Abstract
Constructing functional connectivity (FC) based on brain atlas is a common approach to autism spectrum disorder (ASD) diagnosis, which is a challenging task due to the heterogeneity of the data. Utilizing graph convolutional network (GCN) to capture the topology of FC is an effective method for ASD diagnosis. However, current GCN-based methods focus more on the relationships between brain regions and ignore the potential population relationships among subjects. Meanwhile, they limit the analysis to a single atlas, ignoring the more abundant information that multi-atlas can provide. Therefore, we propose a multiatlas representation based ASD diagnosis. First, we propose a dense local triplet GCN considering the relationship between the regions of interests. Then, further considering the population relationship of subjects, a subject network global GCN is proposed. Finally, to utilize multi-atlas representations, we propose multi-atlas mutual learning for ASD diagnosis. Our proposed method is evaluated on 949 subjects from the Autism Brain Imaging Data Exchange. The experimental results show that the accuracy and an area under the receiver operating characteristic curve (AUC) of our method reach 78.78% and 0.7810, respectively. Compared with other methods, the proposed method is more advantages. In conclusion, our proposed method guides further research on the objective diagnosis of ASD.
MoreTranslated text
Key words
Autism Spectrum Disorder Diagnosis,Multi-atlas Representations,Graph Convolutional Network
AI Read Science
Must-Reading Tree
Example
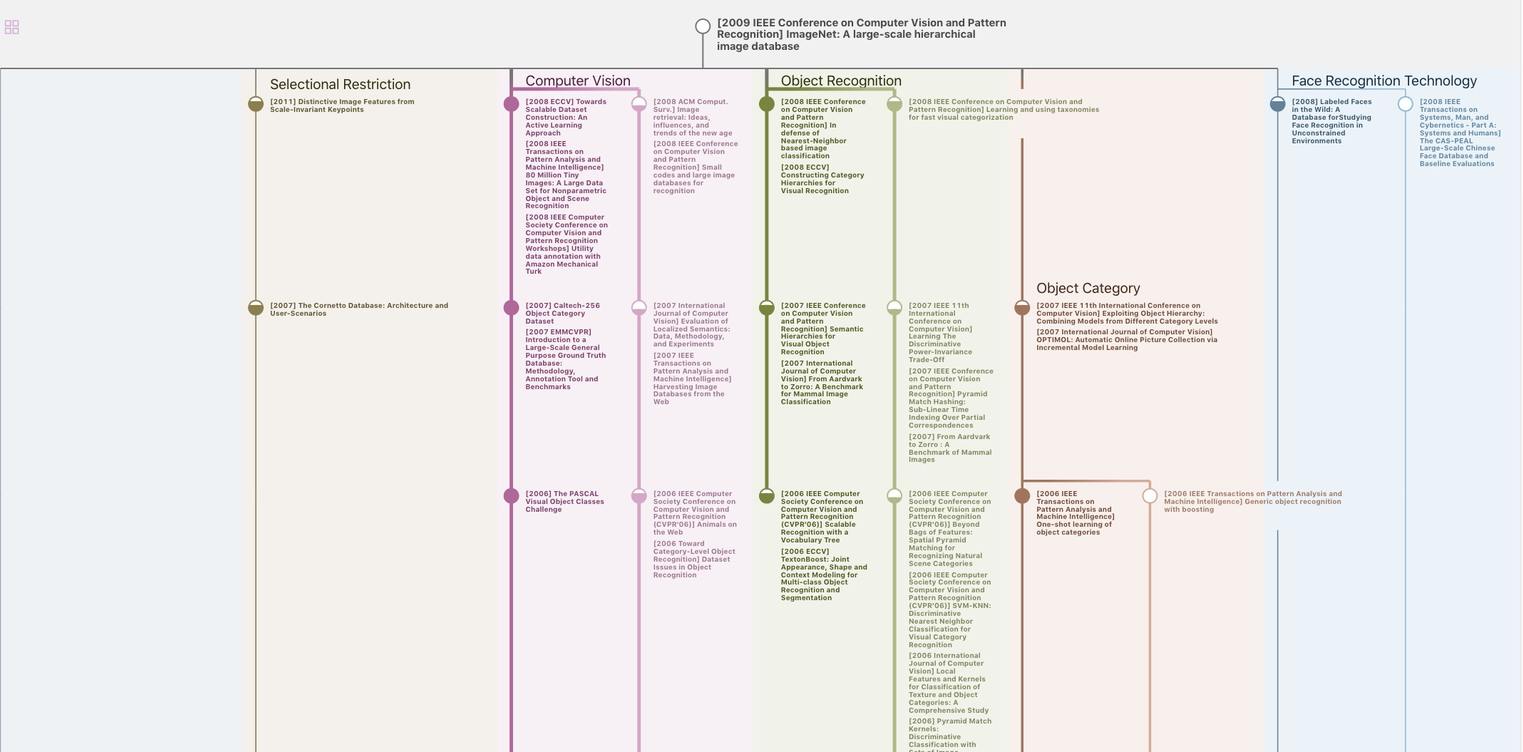
Generate MRT to find the research sequence of this paper
Chat Paper
Summary is being generated by the instructions you defined