An ANN-Guided Approach to Task-Free Continual Learning with Spiking Neural Networks
PATTERN RECOGNITION AND COMPUTER VISION, PRCV 2023, PT VIII(2024)
摘要
Task-Free Continual Learning (TFCL) poses a formidable challenge in lifelong learning, as it operates without task-specific information. Leveraging spiking neural networks (SNNs) for TFCL is particularly intriguing due to their promising results in low-energy applications. However, existing research has predominantly focused on employing SNNs for solving single-task classification problems. In this work, our goal is to utilize ANN to guide SNN in addressing catastrophic forgetting and model compression issues, while treating SNNs as the basic network of the model. We introduce AGT-SNN (ANN-Guided TFCL for Spiking Neural Networks), a novel framework that empowers SNNs to engage in lifelong learning without relying on task-specific information. We conceptualize the learning process of the model as a multiplayer game, involving participants in the roles of players and referees. Our model's fundamental components comprise player-referee pairs, where the player module adopts a SNN-based Variational Autoencoder (VAE) and the referee module employs a ANN-based Generative Adversarial Network (GAN). To dynamically expand the number of components, we propose an innovative method called Adversarial Similarity Expansion (ASE). ASE evaluates the performance of the current player against previously learned players without accessing any task-specific information. Additionally, we propose a innovative pruning strategy that selectively removes redundant components while preserving the diversity of knowledge, thereby reducing the model's complexity. Through comprehensive experimental validation, we demonstrate that our proposed framework enables SNNs to achieve exceptional performance while maintaining an appropriate network size.
更多查看译文
关键词
Task-free continual learning,Spiking nerual networks,Model expansion and compression,Image classification
AI 理解论文
溯源树
样例
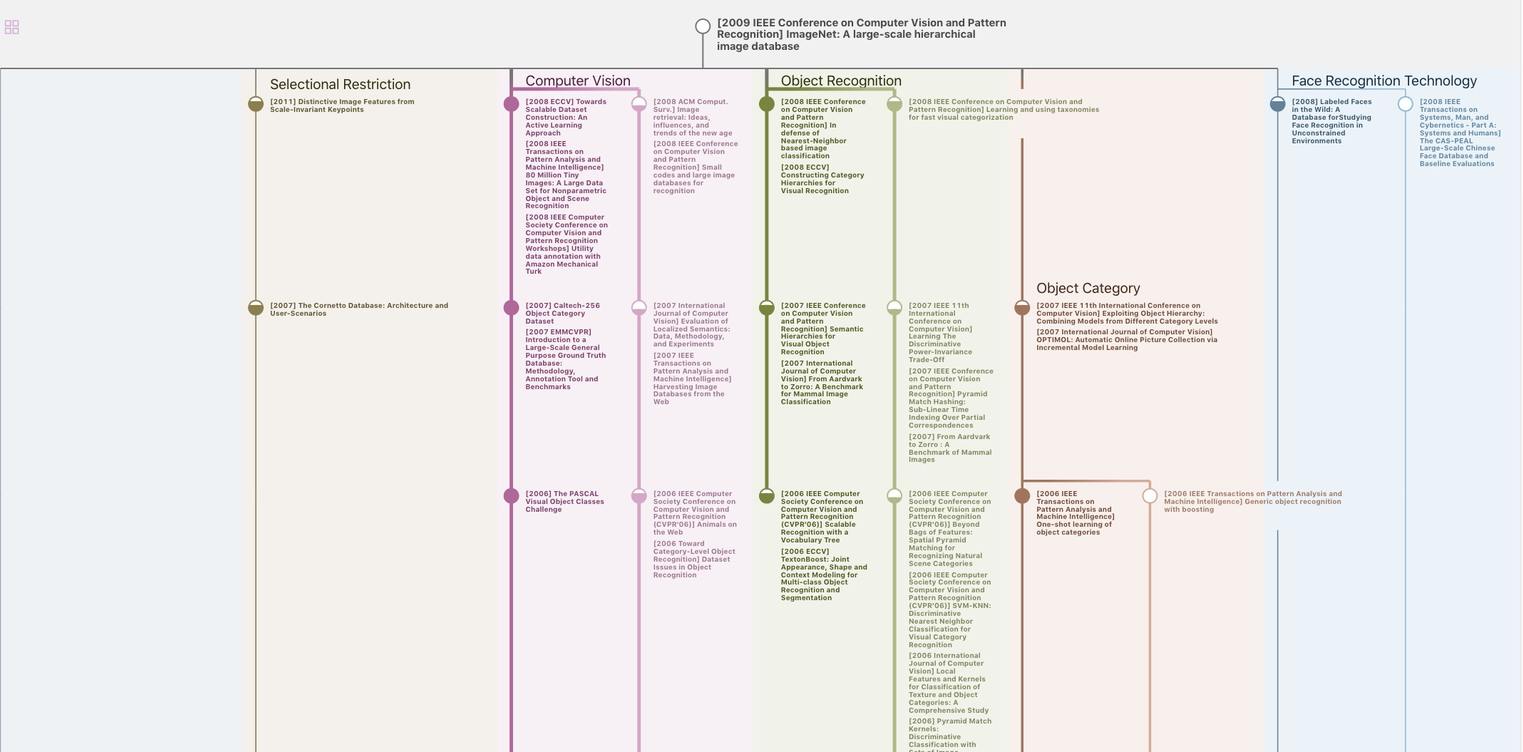
生成溯源树,研究论文发展脉络
Chat Paper
正在生成论文摘要