Space-Transform Margin Loss with Mixup for Long-Tailed Visual Recognition
PATTERN RECOGNITION AND COMPUTER VISION, PRCV 2023, PT VIII(2024)
摘要
In the real world, naturally collected data often exhibits a long-tailed distribution, where the head classes have a larger number of samples compared to the tail classes. This long-tailed data distribution often introduces a bias in classification results, leading to incorrect classifications that harm the tail classes. Mixup is a simple but effective data augmentation method that transforms data into a new shrinking space, resulting in a regularization effect that is beneficial for classification. Therefore, many researchers consider using Mixup to promote the performance of long-tailed learning. However, these methods do not consider the special space transformation of data caused by Mixup in long-tail learning. In this paper, we present the Space-Transform Margin (STM) loss function, which offers a novel approach to dynamically adjusting the margin between classes by leveraging the shrinking strength introduced by Mixup. In this way, the margin of data can adapt to the special space transformation of Mixup. In the experiments, our solution achieves state-of-the-art performance on benchmark datasets, including CIFAR10-LT, CIFAR100-LT, ImageNet-LT, and iNaturalist 2018.
更多查看译文
关键词
Long-tail learning,Mixup,Visual recognition
AI 理解论文
溯源树
样例
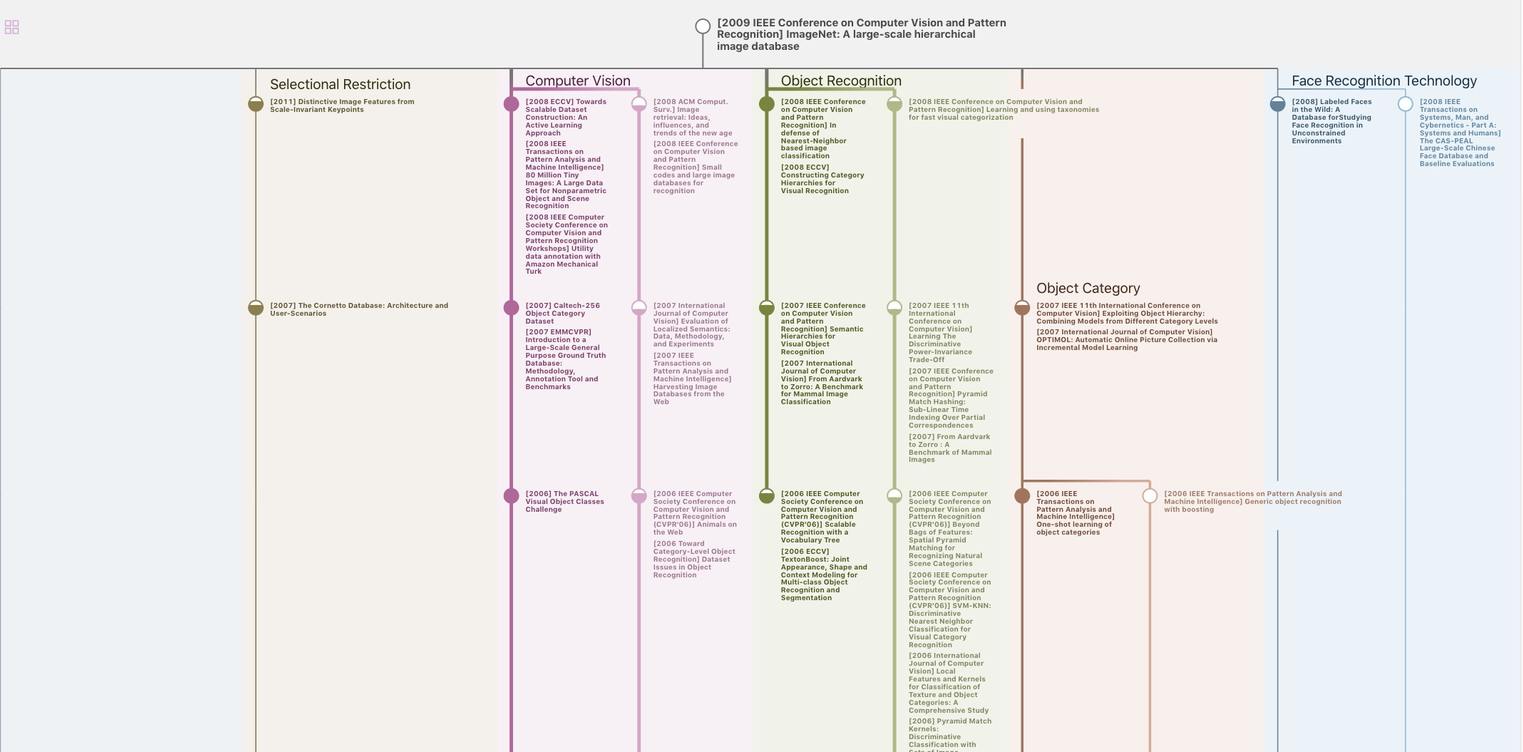
生成溯源树,研究论文发展脉络
Chat Paper
正在生成论文摘要