LATrans-Unet: Improving CNN-Transformer with Location Adaptive for Medical Image Segmentation
PATTERN RECOGNITION AND COMPUTER VISION, PRCV 2023, PT XIII(2024)
摘要
Convolutional Neural Networks (CNNs) and Vision Transformers (ViTs) have been widely employed in medical image segmentation. While CNNs excel in local feature encoding, their ability to capture long-range dependencies is limited. In contrast, ViTs have strong global modeling capabilities. However, existing attention-based ViT models face difficulties in adaptively preserving accurate location information, rendering them unable to handle variations in important information within medical images. To inherit the merits of CNN and ViT while avoiding their respective limitations, we propose a novel framework called LATrans-Unet. By comprehensively enhancing the representation of information in both shallow and deep levels, LATrans-Unet maximizes the integration of location information and contextual details. In the shallow levels, based on a skip connection called SimAM-skip, we emphasize information boundaries and bridge the encoder-decoder semantic gap. Additionally, to capture organ shape and location variations in medical images, we propose Location-Adaptive Attention in the deep levels. It enables accurate segmentation by guiding the model to track changes globally and adaptively. Extensive experiments on multi-organ and cardiac segmentation tasks validate the superior performance of LATrans-Unet compared to previous state-of-the-art methods. The codes and trained models will be available soon.
更多查看译文
关键词
Medical image segmentation,Transformer,Location information,Skip connection
AI 理解论文
溯源树
样例
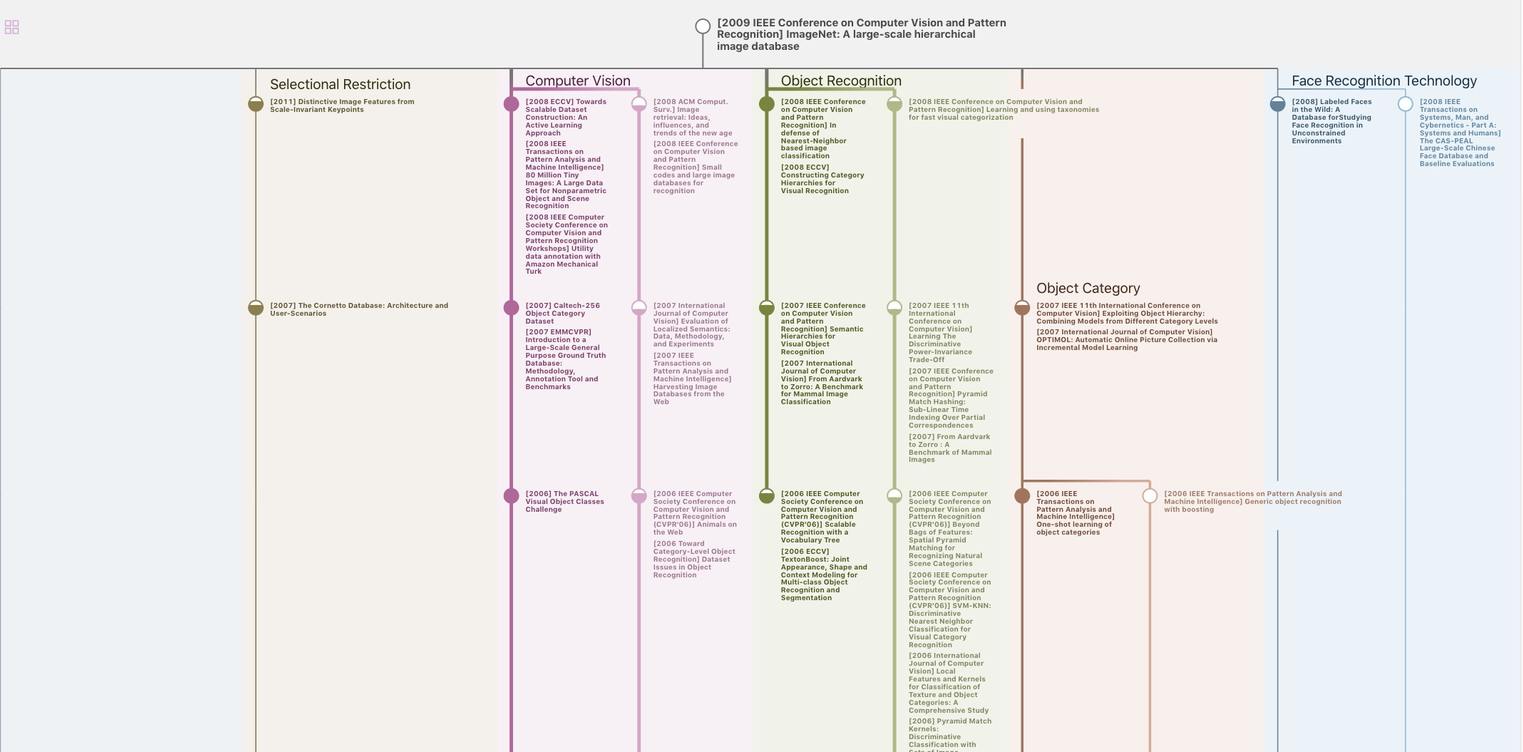
生成溯源树,研究论文发展脉络
Chat Paper
正在生成论文摘要