Misalignment Insensitive Perceptual Metric for Full Reference Image Quality Assessment
PATTERN RECOGNITION AND COMPUTER VISION, PRCV 2023, PT XI(2024)
摘要
Full-reference (FR) image quality assessment (IQA) is crucial in the evaluation of restored images by comparing them with pristine-quality reference images, offering invaluable insights into the effectiveness of image restoration algorithms. Recently, with the advancement of generative adversarial networks (GANs), the GAN-based restoration algorithms demonstrate excellent restoration capability. Nevertheless, these algorithms introduce local spatial misalignment between the restored and the original reference images, posing a challenge for FR-IQA. To tackle this issue, we present a Misalignment Insensitive Perceptual Metric (MIPM) that strengthens the three components of FR-IQA, namely feature extraction, difference representation and quality regression. Specifically, a Vision Transformer-based network for global feature extraction is employed. Furthermore, MIPM utilizes Local Overlapping Wasserstein Difference (LOWD) and Channel Attention Block (CAB) to provide more accurate difference representation between the features of reference and distorted images in the spatial and channel dimensions, respectively. Lastly, a hybrid loss aids in regressing scores that align better with human subjective perception. Coupled with three key improvements, our MIPM exhibits superior performance over state-of-the-art approaches on five IQA datasets, LIVE, CSIQ, TID2013, KADID-10k, and PIPAL.
更多查看译文
关键词
Image quality assessment,Misalignment insensitive,Wasserstein distance
AI 理解论文
溯源树
样例
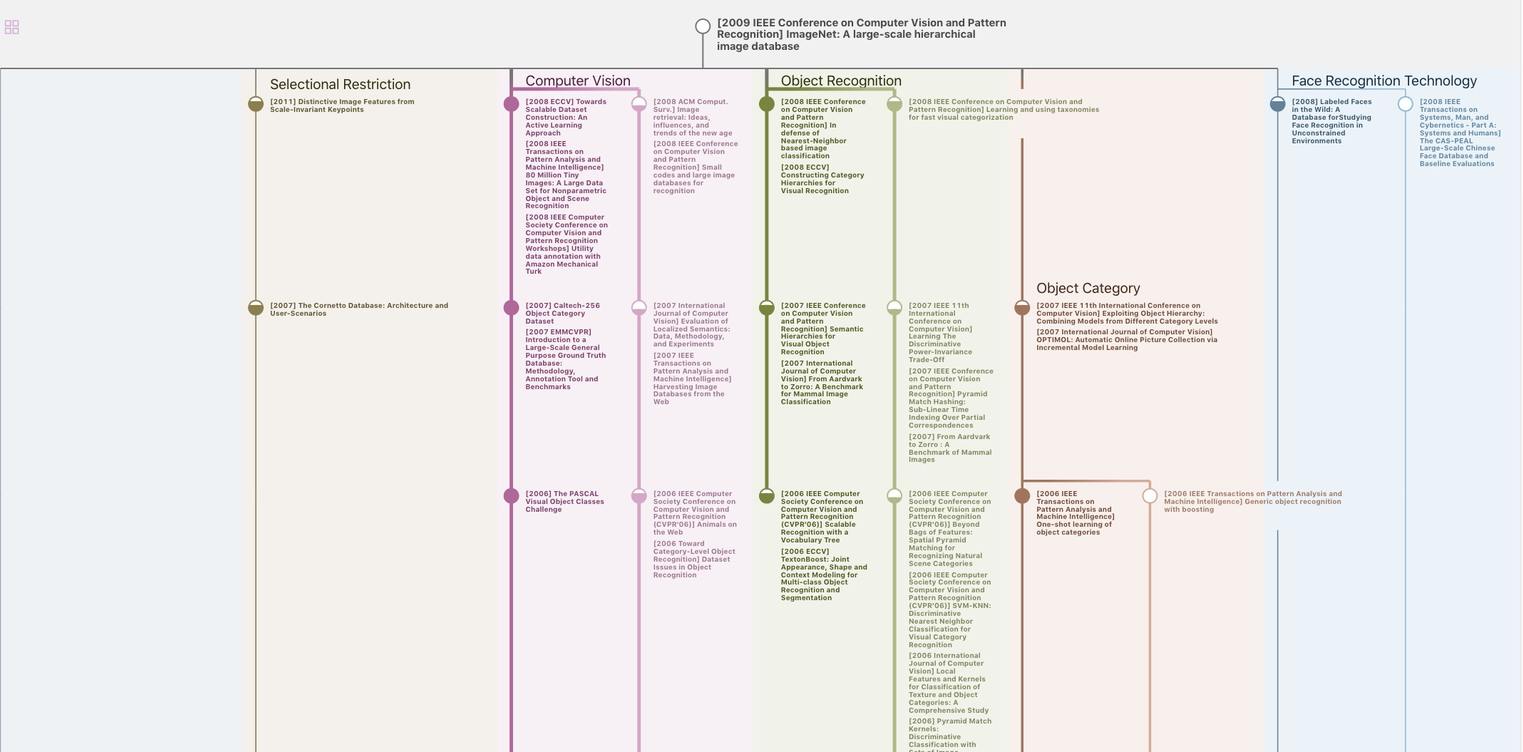
生成溯源树,研究论文发展脉络
Chat Paper
正在生成论文摘要