Dynamic Neural Networks for Adaptive Implicit Image Compression
PATTERN RECOGNITION AND COMPUTER VISION, PRCV 2023, PT XI(2024)
摘要
Compression with Implicit Neural Presentations (COIN) is a neural network image compression method based on multilayer perceptron (MLP). COIN encodes an image with an MLP that maps pixel positions to RGB values matching, the weights of the MLP are quantized to obtain a code stored as an image. However, this single implicit network structure performs generally when dealing with images of multiple complexities. In this paper, we propose a novel implicit dynamic neural network to process images in a dynamic and adaptive manner. Specifically, this paper uses the Sobel operator to divide the complexity of the images and use it as a criterion to select the network width and depth adaptively. To better fit the image features, this paper concludes with further quantification of the dynamic network parameters and storage matrices. Therefore, only some of the relevant network parameters with their storage matrices are required when storing the images. In training this dynamic network, this paper uses a meta-learning approach for the multi-image compression task. Experimental results show that our method outperforms COIN and JPEG in terms of image reconstruction results for the CIFAR-10 dataset.
更多查看译文
关键词
implicit neural representation,dynamic neural network,multi-level image compression,low-rank matrix synthesis
AI 理解论文
溯源树
样例
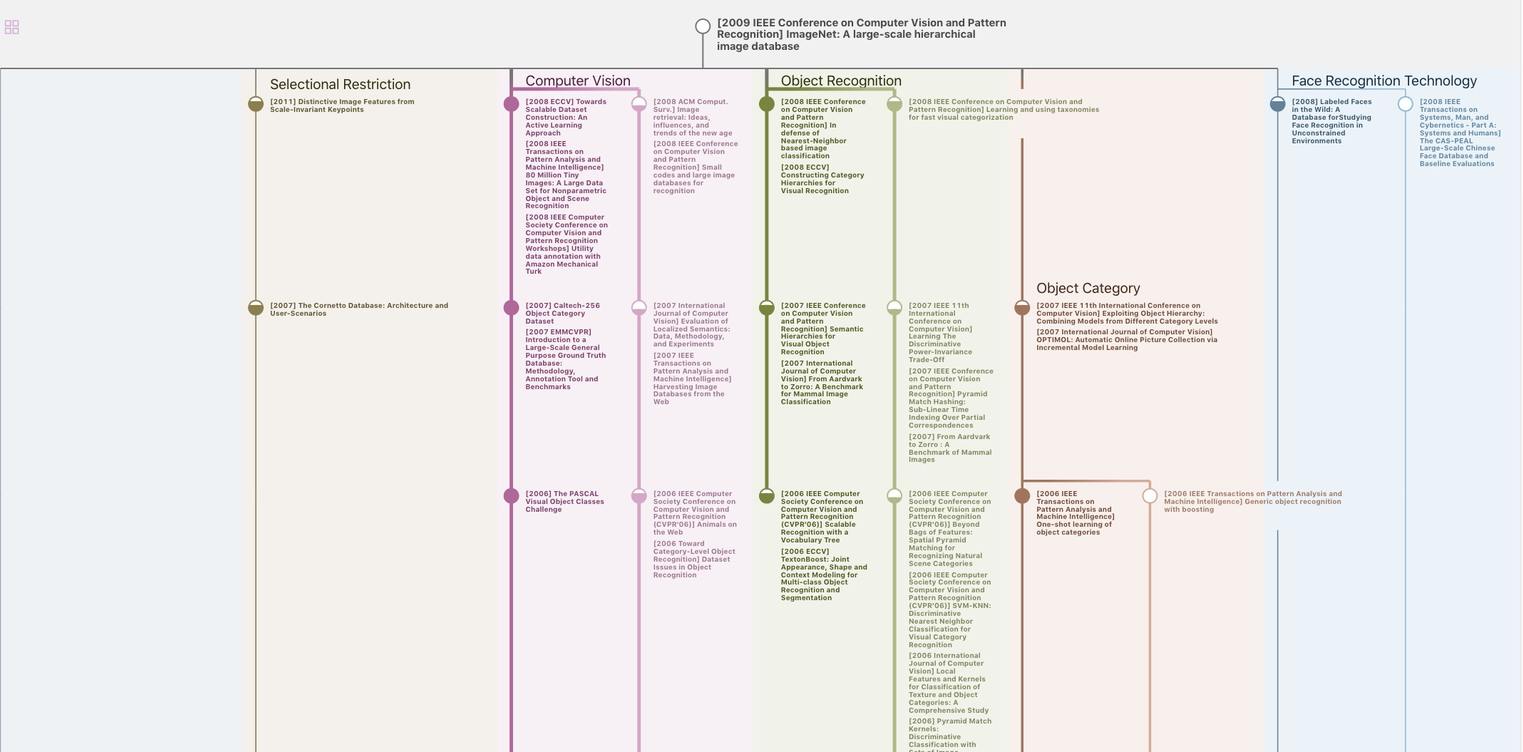
生成溯源树,研究论文发展脉络
Chat Paper
正在生成论文摘要