Semantically Guided Bi-level Adaptation for Cross Domain Crowd Counting
PATTERN RECOGNITION AND COMPUTER VISION, PRCV 2023, PT XI(2024)
摘要
Visual crowd counting has played an important role in various practical applications. However, domain gap remains a major barrier preventing models trained on the source domain (e.g., training scenes) generalize well to the target domain (e.g., unseen testing scenes). Crowd semantic information are shown to be beneficial to assist crowd counting in supervised training settings, implying the close relationship between crowd density and semantics. Nevertheless, the potential of this powerful cue has bot been fully explored in the unsupervised domain adaptation (UDA) setting. Motivated by the observation that crowd density map share domain-invariant correspondence with the crowd segmentation map, we propose to adapt this correspondence correlation from the source domain to the target domain to address the domain gap. To this end, a semantically guided task correlation layer is introduced to extract the task correspondence map, whose coherence is enforced across domains by adversarial training. To drive the adaption of earlier hidden layers directly, we further align the task correspondence correlation upon intermediate-level outputs. Extensive experiments are conducted on three benchmark datasets. The performances of our method either surpass or are on par with the counterparts, demonstrating the effectiveness of the proposed approach for cross-domain crowd counting.
更多查看译文
关键词
Crowd counting,Task correspondence,Domain adaptation
AI 理解论文
溯源树
样例
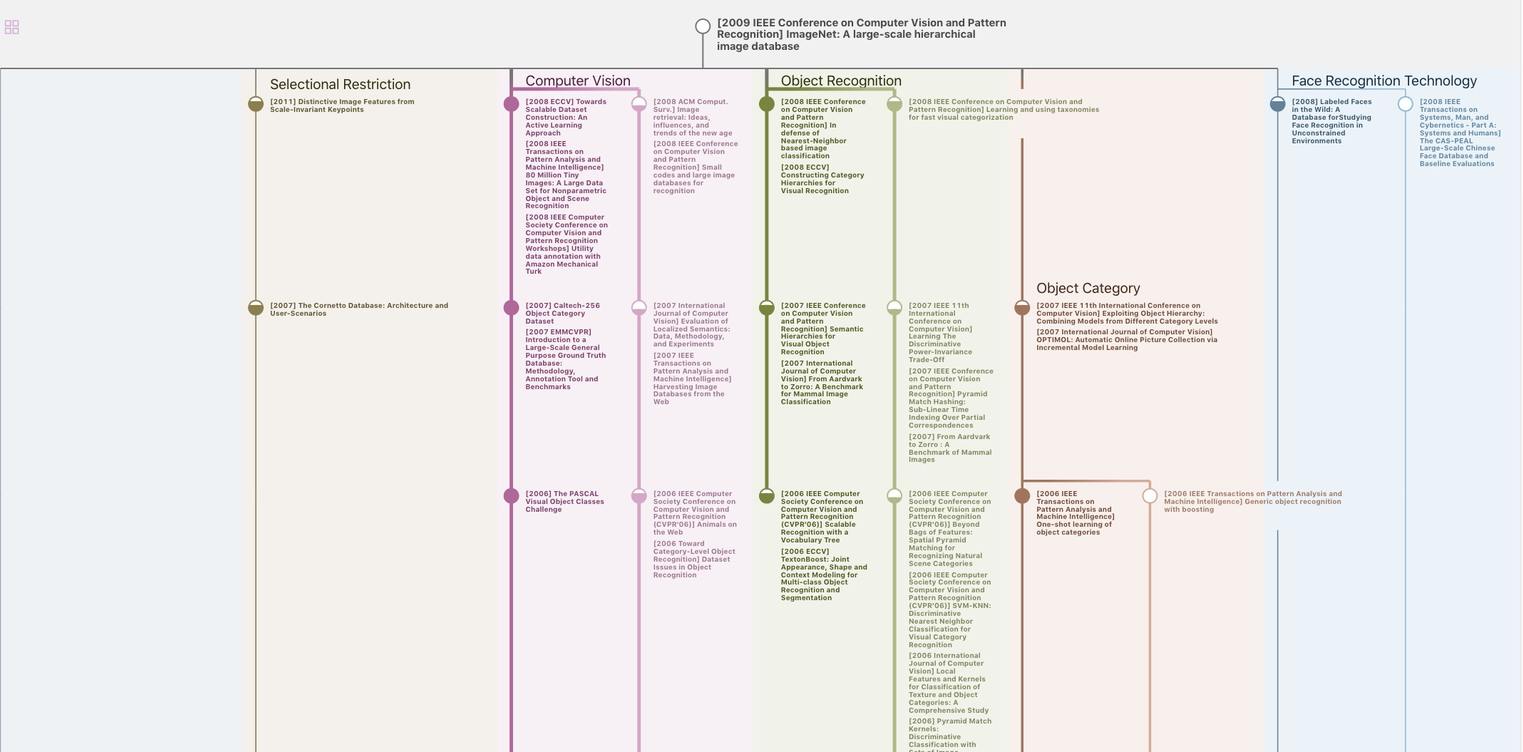
生成溯源树,研究论文发展脉络
Chat Paper
正在生成论文摘要