Robust Subspace Learning with Double Graph Embedding
PATTERN RECOGNITION AND COMPUTER VISION, PRCV 2023, PT VII(2024)
摘要
Low-rank-based methods are frequently employed for dimensionality reduction and feature extraction in machine learning. To capture local structures, these methods often incorporate graph embedding, which requires constructing a zero-one weighted neighborhood graph to extract local information from the original data. However, these methods are incapable of learning an adaptive graph that reveals intricate relationships among distinct samples within noisy data. To address this issue, we propose a novel unsupervised feature extraction method called Robust Subspace Learning with Double Graph Embedding (RSL_DGE). RSL_DGE incorporates a low-rank graph into the graph embedding process to preserve more discriminative information and remove noise simultaneously. Additionally, the l(2,1)-norm constraint is also imposed on the projection matrix, making RSL_DGE more flexible in selecting feature dimensions. Several experiments demonstrate that RSL_DGE achieves competitive performance compared to other state-of-the-art methods.
更多查看译文
关键词
Low-rank representation,Graph embedding,Feature extraction,Subspace learning
AI 理解论文
溯源树
样例
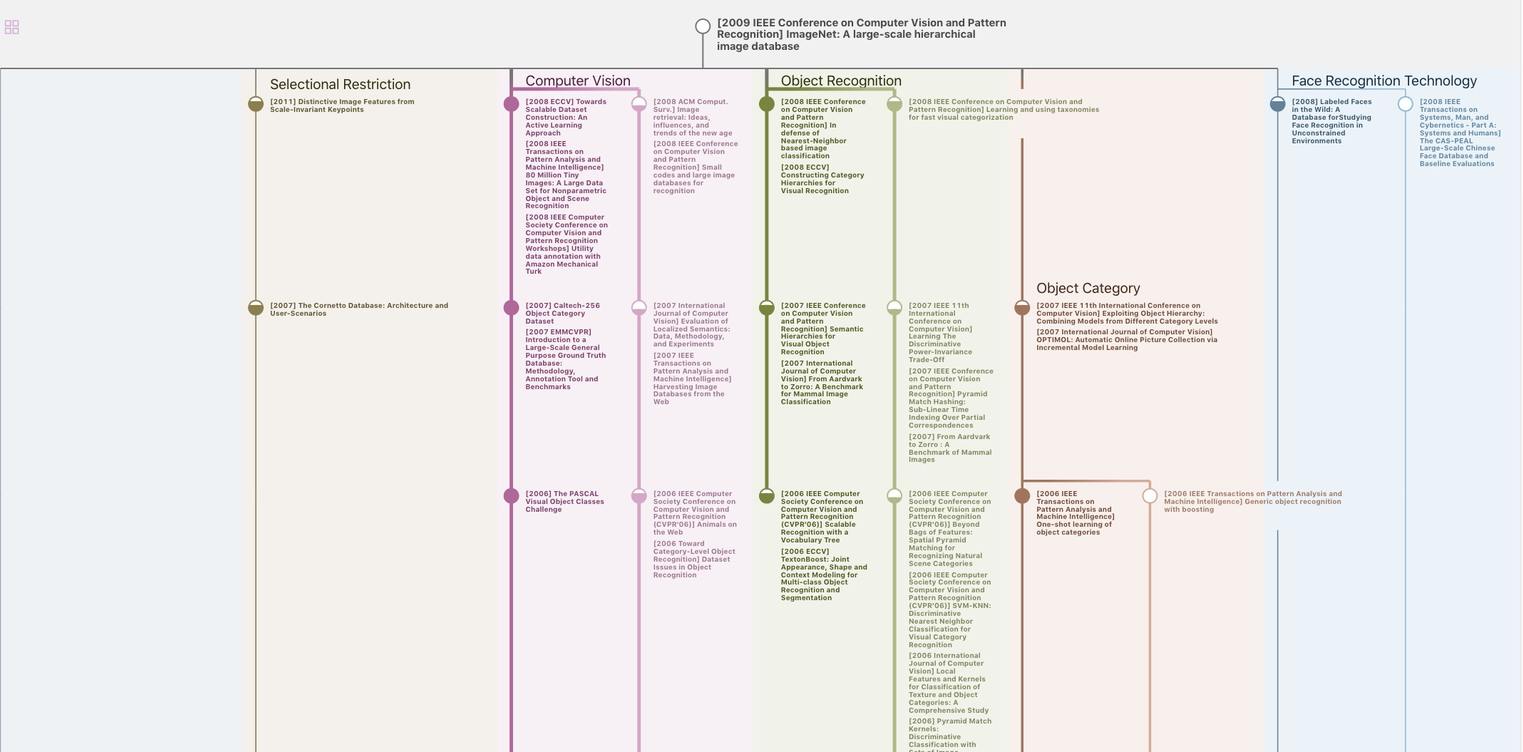
生成溯源树,研究论文发展脉络
Chat Paper
正在生成论文摘要